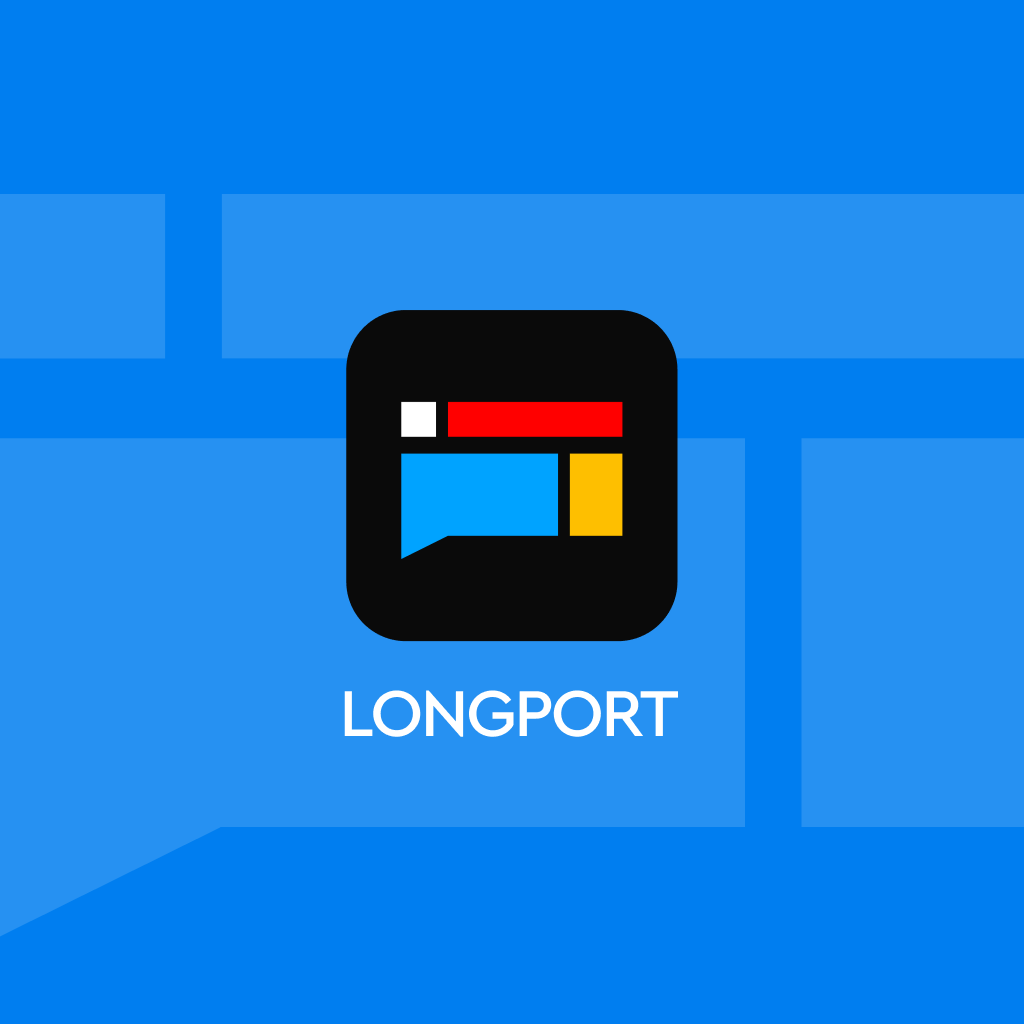
How does OpenAI defend its moat? Who will be eliminated? This is the future industry landscape of AI in the eyes of venture capitalists.
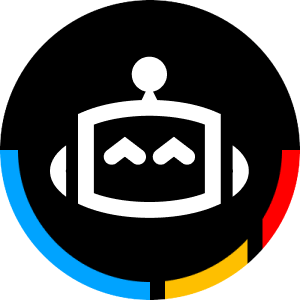
What will be the future pattern of the AI industry? Can leading large-scale model companies maintain their position? Which types of companies will struggle to survive? How valuable is proprietary data? When will true AGI arrive?
This year, with ChatGPT as a representative of generative AI, it has sparked a wave of development in the artificial intelligence industry.
In fact, the development of artificial intelligence and deep learning technology has been going on for many years. All the hype surrounding AI has overlooked a simple fact: these technologies have been developing for decades.
After more than 70 years of continuous development, the three key elements have finally come together: advanced algorithms, large-scale datasets, and powerful computing capabilities, forming a perfect storm of artificial intelligence.
To better understand the areas where startups still have opportunities, as well as the formation of oligopoly and first-mover advantages, TechCrunch interviewed different investors in a column article, aiming to delve into the development trends of artificial intelligence and the industry as a whole.
Can leading large-scale model companies maintain their position? What is the value of proprietary data? When will true AGI arrive?
Manish Singhal, founding partner of pi Ventures, shared his views on whether leading large-scale model companies can maintain their position, the geometric value of proprietary data, and when true AGI will arrive.
Manish Singhal pointed out that the AI industry is in a dynamic and changing landscape. Many companies have been established for downstream applications, but only a few have been successful in scaling up. As for the foundational models, it is expected that OpenAI will face competition from other companies in the future. However, they have already gained a favorable advantage, so it won't be easy to surpass them.
In the next five years, there should be significant consolidation in the field of artificial intelligence applications. AI is expanding horizontally, so companies built on existing models will face challenges in maintaining their moats. However, there is a lot of foundational innovation happening in the application and infrastructure (tools and platforms) areas, and they may outperform other companies.
Regarding whether open source is the most obvious market route for AI startups, Singhal pointed out that it depends on what problem they are trying to solve. It is an effective approach for companies in the infrastructure layer, but it may not be effective for all companies. Therefore, whether open source is a good path should be considered based on the specific problem they are trying to solve.
As for the future development of LLM (large language model), Singhal mentioned that English is the most widely used language. Depending on the situation in different regions, LLM models for different languages definitely make sense. In addition to language differences, there is also a desire to see large model variants specifically tailored to certain fields such as medicine, law, and finance, in order to provide more accurate and relevant information in these domains. Some work is already being done in this regard, such as BioGPT and Bloomberg GPT.
Regarding the value of data, Singhal pointed out that proprietary data can provide a good starting point, but it is difficult to maintain data exclusivity in the long run. Therefore, the technological moat comes from the combination of intelligent design algorithms, which are productized and fine-tuned for specific applications along with the data.In addition, Singhal believes that there is still a lot of work to be done in terms of the accuracy of the answers provided by the models. Currently, it may take longer than a few months for the LLM method based on neural networks to be disrupted. Once quantum computing becomes mainstream, the landscape of artificial intelligence will undergo significant changes again.
Currently, some applications are approaching human-level performance, but true AGI is still far away. Singhal believes that this is an asymptotic curve that may take a long time to fully achieve. To achieve true AGI, the development of several technologies such as neuroscience and behavioral science must also catch up.
What is the future landscape of the AI industry? Which types of companies will struggle to survive?
Regarding the future landscape of the AI industry, Rudina Seseri, the founder and managing partner of AIGlasswing Ventures, pointed out that Alphabet, Microsoft/OpenAI, Meta, and other foundational model providers are likely to maintain their market leadership and exert oligopoly power in the long term. However, there are also competitive opportunities for foundational layer companies that provide differentiated models, such as Cohere and other well-funded companies.
Rudina Seseri further pointed out that foundational layer startups may end up in one of two states: one is an oligopoly situation, where a few companies will dominate the majority of the value, similar to what we have seen in the cloud computing market. The other possibility is that foundational models will be primarily provided by the open-source ecosystem.
For founders and venture capitalists, the application layer holds the greatest opportunities. Companies that can provide tangible and measurable value to customers can replace large companies in existing categories and dominate in new categories. Just as the value creation of cloud computing did not end with the emergence of cloud computing infrastructure providers, the significant value creation in the entire field of artificial intelligence has yet to come, and the competition in artificial intelligence is far from over.
As for which companies will struggle to survive, Rudina Seseri pointed out that some niche markets in the AI field may not be able to sustain long-term development. One example is the "GPT packaging" category, which revolves around solutions or products built on OpenAI's GPT technology. These solutions lack differentiation and are easily disrupted by features introduced by dominant companies in the market. Therefore, in the long run, they will struggle to maintain a competitive advantage.
Similarly, companies that cannot provide significant commercial value or solve high-value and expensive domain problems will not become sustainable enterprises. Consider this: a solution that simplifies simple tasks for interns will not scale into a significant business, while a platform that solves complex problems for chief architects is different as it can provide unique high-value advantages.
Finally, if a product cannot seamlessly integrate into current enterprise workflows and architectures or requires significant upfront investment, companies will face challenges in implementation and adoption. This will be a major obstacle to achieving meaningful return on investment, as the threshold is much higher when behavior and costly architectures need to be changed.