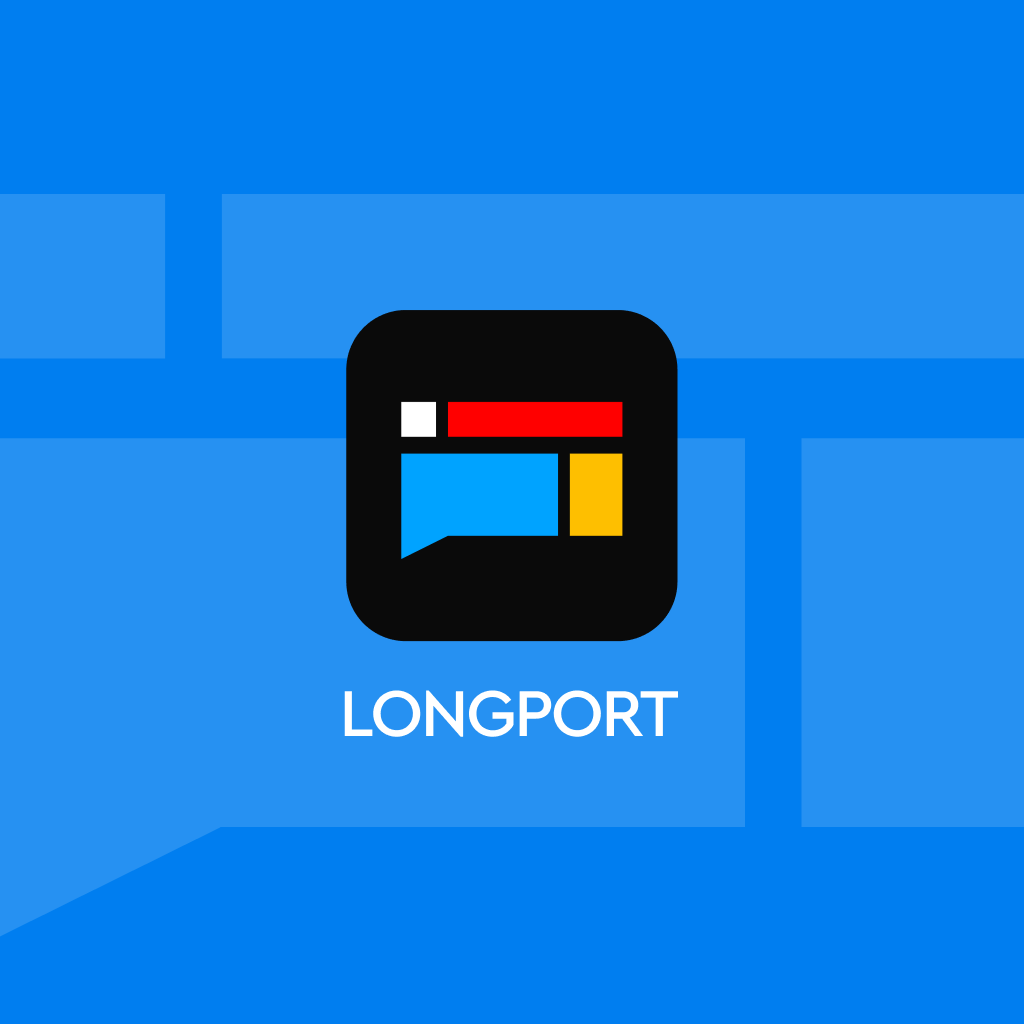
Track Hyper | AI Large Models Sprinting Towards Intelligent Mobile Endpoints
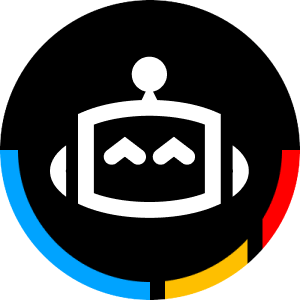
Unexpected success of Honor, Huawei's efforts, and Qualcomm's silent progress.
The revolutionary technological breakthrough in smartphones has been stagnant for a long time. After the emergence of GPT, the industry gradually reached a consensus: the deployment of large models on the terminal side of smart terminals (including smartphones) will once again usher in an exciting era of major innovation.
During MWC 2023 in Shanghai, Honor CEO Zhao Ming announced that Honor will promote the deployment of large models on the terminal side of smartphones as the starting point for a new round of product technological breakthroughs. However, on July 12th, Zhao Ming did not disclose any information about the terminal-side AI large model of their new generation foldable screen phone, Magic V2.
Currently, the presentation form of terminal-side large models is all software-based. It is understood that Honor's AI large model will also be presented in software form and is likely to be integrated into the MagicOS 8.0 version.
Unlike Honor's focus on the future deployment of AI large models on smartphones, in February of this year, Qualcomm has already deployed the Stable Diffusion model on Android smartphones, which can generate AI images in just over ten seconds.
Ziad Asghar, Senior Vice President of Product Management and AI at Qualcomm, believes that large models will quickly reshape human-computer interaction.
Qualcomm takes the lead: Insight into individual needs
On July 12th, Honor released its new flagship phone, "Magic V2." On June 29th, Honor CEO Zhao Ming publicly stated that Magic V2 will have a revolutionary leap in experience. Honor hopes to break Apple's dominance in the industry with this phone. Zhao Ming also stated that Honor will be the first to introduce AI large models on the terminal side.
However, on July 12th, Zhao Ming's description of the technical features or product characteristics of Magic V2 focused on its thickness (9.9mm) and weight (231 grams), without mentioning the terminal-side AI large model. This is in stark contrast to Zhao Ming's previous promotion of the terminal-side AI large model of Magic V2. If we carefully consider Zhao Ming's statement on June 29th - "We will be the first to introduce AI large models on the terminal side in the future," Zhao Ming may have hinted at something. According to reports, the upcoming MagicOS 8.0 version from Honor is likely to take action in deploying AI large models.
The capabilities of Honor's AI large models on the terminal side and the nature of the software matrix (including compilers/decoders, computing platforms, power consumption control, parameter quantity, and development tools) are currently unknown.
From an industry perspective, Qualcomm achieved AI model deployment on smartphones for the first time in February this year. By May, the parameters of the Stable Diffusion model deployed by Qualcomm had increased to over 1 billion.
Stable Diffusion is a generative AI diffusion model from text to image, which can create realistic images based on any text input in a matter of seconds. Currently, the most popular AI painting models are Midjorney and Stable Diffusion, but the Midjourney model is not currently open source. Stable Diffusion was proposed by StabilityAI in 2022, and both the paper and the code have been open sourced. Stable Diffusion is an improved version of Diffusion, mainly aimed at solving the speed issue of the Diffusion model.
As for how text generates images, the technical explanation is too complex. Simply put, from the initial name of Stable Diffusion, "Latent Diffusion Model (LDM)", it essentially compresses the pixels of the image, reducing its size, and then uses a compiler (why mention the compiler included in the Honor side large model?) to restore the compressed image to its original size. The rest of the process is similar to the Diffusion model.
During the compression of the image, the speed of converting text into images has been improved, which is the main function of Stable Diffusion.
Now let's talk about the deployment of the Stable Diffusion model by Qualcomm in Android smartphones. Implementing text-to-image conversion is just a tiny "element" of the revolutionary application experience of smartphones when deploying large models on the edge, like a speck of dust in the universe.
By deploying large models on the edge, digital assistants will become an existence beyond imagination. In the future, users will have the privilege of controlling all commercial services through smartphones, including catering, various types of ticketing, professional consulting, entertainment, photography and videography, writing, office work, and participating in financial activities, and so on.
This can truly achieve what Ziad Asghar, the AI head of Qualcomm, said, "Large models have the ability to truly reshape the way we interact with applications."
Only by truly deploying large AI models on the edge can the term "intelligence" in smart terminals be truly justified.
Zhao Ming said, "The mission of edge AI models is to better understand users: knowing when I go to sleep, knowing what I like to eat, and being able to meet my immediate needs, which is equivalent to having the ability to understand my needs."
To achieve insight into the personalized needs of users, the reason is that the personal application data contained in each smartphone, combined with large language models that can understand text, audio, images, and other multimodal inputs, can ultimately enable digital forms of smartphones (such as virtual digital humans) to accurately grasp the user's preferences. What's more important is that such powerful personalized experiences can be built on the basis of protecting individual privacy.
How to address the shortcomings of edge AI models
Currently, no technology company has been able to fully deploy large AI models on the edge.
Qualcomm and Huawei have become pioneers in this field. The difference between the two lies in the fact that Qualcomm is more systematic, starting from the underlying technology, such as using the Qualcomm AI Stack to perform full-stack AI optimization, while Huawei focuses more on specific application experiences. However, compared to Qualcomm, Huawei's exploration is more concrete, making it more characteristic of incremental attempts. From a technical perspective, Qualcomm's deployment of the Stable Diffusion model in smartphones is actually integrating the model into the hybrid AI architecture of the phone. This allows for the quantization, compilation, and hardware acceleration optimization of AI technologies, supporting highly intelligent application experiences.
If Honor truly deploys edge-side AI large models in MagicOS 8.0, it will also be based on this technological principle.
In fact, through natural language processing (NLP) search, the Huawei P60 is already capable of matching photos that correspond to the description. This functionality is just a small application within the powerful capabilities of edge-side AI large models.
The implementation of this application experience is supported by Huawei's multimodal large model technology and model miniaturization processing technology. Huawei has integrated the natural language intelligent image search model into the HarmonyOS system, creating a unique and precise natural language mobile gallery search experience.
Compared to Huawei, Qualcomm's deployment of edge-side AI large models focuses more on systematic features.
For example, Qualcomm's full-stack AI research refers to optimizing cross-application neural network models, algorithms, software, and hardware. Regarding Stable Diffusion, Qualcomm starts with the open-source models from Hugging Face (an open-source model library company, with its flagship open-source library being "Transformers") versions FP32 1-5. Through quantization, compilation, and hardware acceleration optimization, Qualcomm enables these models to run on smartphones powered by the second-generation Snapdragon 8 mobile platform.
Deploying AI large models on intelligent terminals requires addressing performance and power consumption issues.
Firstly, by efficiently running large models on Qualcomm's dedicated AI hardware and reducing memory bandwidth consumption, quantization not only improves performance but also reduces power consumption. These include Qualcomm AIMET quantization techniques such as Adaptive Rounding (AdaRound), which can maintain model accuracy at lower precision levels without the need for retraining.
Secondly, training and quantizing with the Qualcomm AI Model Efficiency Toolkit (AIMET) can compress large models from FP32 to INT8. This toolkit was developed based on technology created by Qualcomm AI Research and is currently integrated into Qualcomm AI Studio.
This quantization process transforms the large models from floating-point numbers to integers, reducing computation time and overall model size while maintaining accuracy, making them easier to deploy on terminals.
In addition, the key to efficient operation of AI models with high performance and low power consumption lies in the compiler. The AI compiler converts the input neural network into code that can run on intelligent application terminals, continuously optimizing for latency, performance, and power consumption.
It is worth mentioning that the AI-dedicated Hexagon processor integrated into the Qualcomm Snapdragon 8 Gen2 5G mobile platform features an independent power supply system. It supports micro-sliced inference, INT4 precision, and Transformer network acceleration, providing higher performance while reducing power consumption and memory usage. This is also part of the Qualcomm AI software stack.
These technologies can be applied to all component models that make up Stable Diffusion, including Transformer-based text encoders, VAE decoders, and UNet. This is crucial for the smooth operation of large models on end devices.
Qualcomm's full-stack AI optimization ultimately enables the execution of 20 steps of inference within 15 seconds and the generation of a 512x512 pixel image using the Stable Diffusion model on smartphones. This is the fastest inference speed on smartphones, comparable to cloud latency, and user text input is completely unrestricted.
Whether it is a large-scale model company, a terminal software and hardware technology company like Qualcomm, or smart terminal vendors like Honor and Huawei, when the industry achieves upstream and downstream collaboration and jointly promotes the ubiquitous deployment of AI large models at the edge, it will truly trigger a new wave of technological innovation in smart terminals and effectively shoulder the responsibility of bringing revolutionary application experiences to smart terminals, as Zhao Ming mentioned.