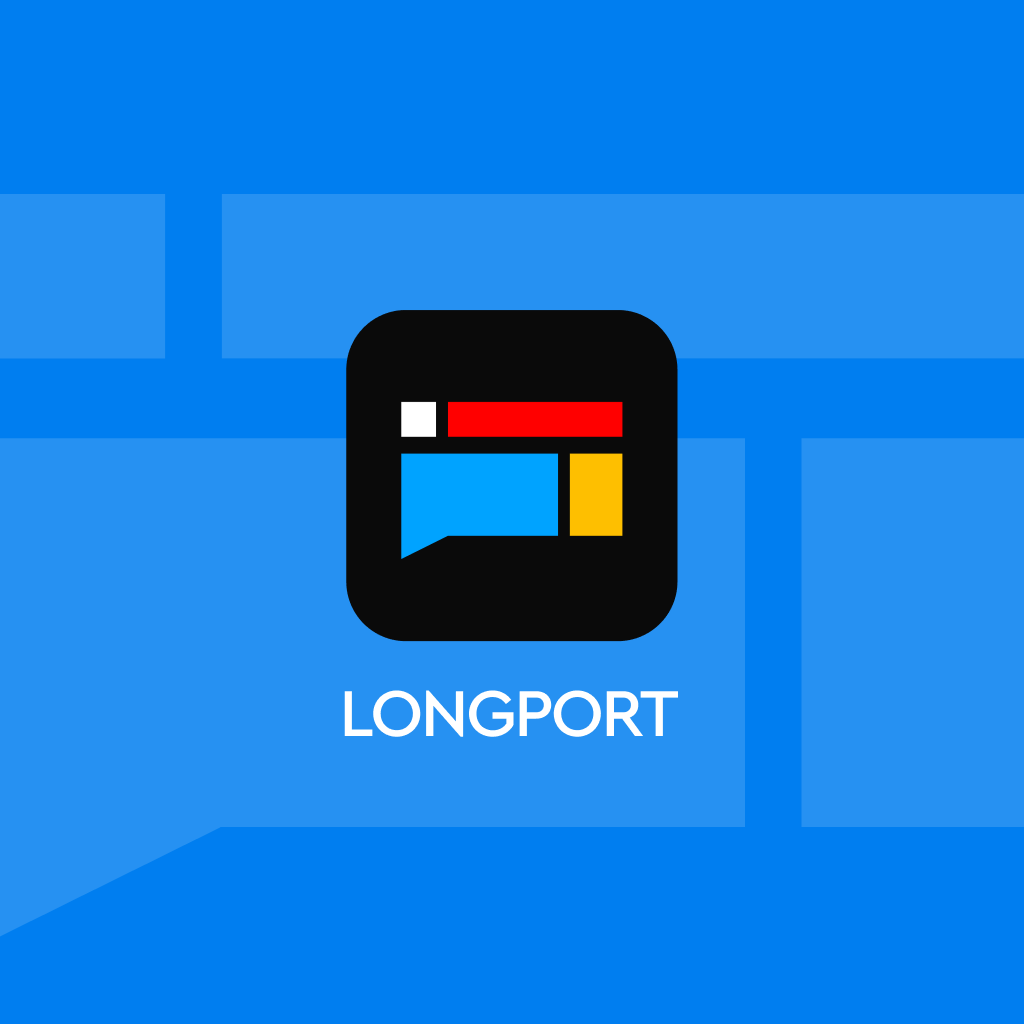
Track Hyper | Universal Large Model for Autonomous Driving: UniAD Technology Vision
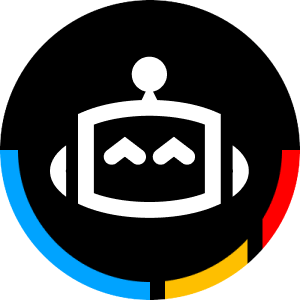
What role does SenseTime play in it?
On June 21st, North American time, the CVPR (Conference on Computer Vision and Pattern Recognition), saw the first-ever best paper on autonomous driving at the conference.
This is somewhat similar to the ChatGPT application, which uses the underlying Transformer model, a paper published by Google at the 2017 Neural Information Processing Systems Conference, which has become a breakthrough in AGI (General Artificial Intelligence) technology, and this paper, which won the "Best" crown at CVPR, may also become a promoter of future advanced automatic driving technology applications.
The significance of advanced autonomous driving technology is that it has proposed a universal large model of autonomous driving with integrated perception and decision-making, called "UniAD", which has opened up a new direction and space for the development of autonomous driving technology and industry by pioneering a large model architecture for autonomous driving with a global task as its goal.
The first self-driving theme in 40 years
CVPR, a professional technical conference in the field of computer vision and pattern recognition hosted by IEEE, is one of the top technical conferences with the most academic influence in the AI field, held once a year.
At this year's conference in 2023, a total of 9,155 technical papers participated in the "Best" competition.
In the end, there were two "Best Papers" and another best student paper. That is, out of 9,155 papers, a total of three technical papers won the "Best Paper Award".
Among them, one of the best papers was jointly researched by Shanghai AI Laboratory, Wuhan University, and SenseTime, titled "Planning-oriented Autonomous Driving", which is the first best paper on autonomous driving in the 40 years since CVPR was held in 1983; it is also the best paper in the last 10 years of the conference with the first author from a Chinese institution.
It is worth mentioning that one of the authors of this best paper, SenseTime, has another best paper candidate, seven highlight papers, and 54 collected papers at this year's CVPR. Industry insiders told Wall Street that the core personnel involved in writing this paper at Shanghai AI Laboratory all have a background in SenseTime.
The "Universal Large Model of Autonomous Driving with Integrated Perception and Decision-Making" proposed in the paper, called "UniAD", has a core technical value of establishing an end-to-end perception and decision-making framework, integrating multi-task joint learning new paradigms, and enabling more effective information exchange and coordination of perception, prediction, and decision-making, thereby further improving path planning capabilities. This is also the reason why the paper won the Best Paper Award. Quite a few insiders in the autonomous driving industry have a similar view when it comes to advanced autonomous driving technology, that is, "advanced autonomous driving is not difficult to overcome or solve technically, but regulations are difficult to synchronize." This statement not only has a literal meaning, but also implies a technical meaning, that is, advanced autonomous driving still cannot form efficient interaction with other vehicles or pedestrians when driving. This is essentially within the scope of multi-task application requirements.
This implied meaning acknowledges that the technology of advanced autonomous driving has not yet achieved effective breakthroughs. Previously, most of the technology focused on solving modular problems, such as improving radar scanning range and accuracy, domain controller performance, or autonomous driving chip performance, etc. These efforts are difficult to balance the "multi-task" and "high-performance" application requirements, especially the former.
UniAD (Unified Autonomous Driving), a universal algorithm framework for autonomous driving, consists of four perception prediction modules based on Transformer decoders and one planning module, which is an overall universal model framework for autonomous driving.
UniAD is the first to integrate the three main tasks of perception, prediction, and planning, as well as six sub-tasks including target detection, target tracking, scene mapping, trajectory prediction, grid prediction, and path planning, into a unified end-to-end network framework based on Transformer, becoming a universal model for driving critical tasks.
In the NuScenes real-world scenario dataset framework, all relevant tasks of UniAD have achieved SoTA (State of The Art), especially the prediction and planning effects far exceed other models.
In short, it solves the "multi-task" problem and achieves hierarchical integration of multiple tasks through multiple Transformer modules. It can also achieve full-angle and multi-directional interaction between different tasks. UniAD models objects and maps through multiple query vectors, and then passes the prediction results to the planning module for planning a safe path.
The autonomous driving full-stack solution using this framework can improve the accuracy of multi-target tracking by 20%, the accuracy of lane prediction by 30%, and reduce the errors of predicted motion displacement and planning by 38% and 28%, respectively.
What's Strong? Can Handle Multiple Tasks
If we observe the reason for the award of this article, it is not difficult to find that UniAD solves the demand for "multi-task" by starting from planning and integrating the entire stack of critical tasks into a unified framework from end to end.
It should be acknowledged that the application of advanced autonomous driving technology was not all modular solutions before, and many international companies have done a lot of framework patterns.
For example, American self-driving companies such as Waymo and Cruise adopt the "independent parallel model" architecture design, while American Tesla and Chinese Xiaopeng Motors propose the "multi-task shared network" architecture pattern. American NVIDIA, the Max Planck Institute in Germany, and Wayve, a self-driving company in the UK, have used a "direct" end-to-end solution. UniAD has for the first time included end-to-end full-stack key tasks in a unified network architecture, proposing a brand new "full-stack controllable" end-to-end solution that achieves better application effects than all previous architectures through system joint optimization.
From a technical perspective, UniAD uses multiple sets of query vectors to link multiple tasks and achieve network information transmission, and then transmits all fused information to the final planning module. At the same time, the Transformer architecture of each module can effectively interact with the query vector through attention mechanism.
In practical applications, UniAD can significantly save computing resources and avoid the accumulation error of different task modules (the previous single modular solution formed a redundant error problem that is difficult to solve after multiple runs). Through UniAD's proof, once a framework that can take into account both "multi-task" and "high-performance" full-stack controllable end-to-end solutions is adopted, the preceding multi-tasks can support the subsequent tasks, ultimately improving the driving safety experience.
In fact, most end-to-end autonomous driving solutions also focus on perception, decision-making, and planning. However, when promoting the multi-tasks formed by these three parts to play a practical role, there are significant differences, and no one can design a unified framework to integrate these tasks that meet different application requirements into a whole.
Why can UniAD solve the problem of multi-task fusion?
The research team used a full Transformer model with multiple sets of query vectors, and the team also based its full-stack design on the "planning" goal.
For example, in the scenario where the vehicle is driving straight on a sunny day, UniAD can perceive the black vehicle waiting in the left front, predict its future trajectory (turning left into the lane of the vehicle), and immediately decelerate to avoid it, and then resume normal speed after the black vehicle leaves.
If it is a rainy day turning scenario, in a complex crossroads with large visual interference, UniAD can generate the overall road structure of the crossroads through the segmentation module, achieving a large-scale left turn planning.
UniAD is called a universal large model for autonomous driving, how should we understand this?
This framework lays the foundation for a multi-task end-to-end autonomous driving large model and has strong scalability. By increasing the model parameters and expanding the massive data-driven, it can further realize the autonomous driving large model, empowering industry applications and related self-driving product landing.
This paragraph is an explanation from Dr. Li Hongyang of the Shanghai Artificial Intelligence (AI) Laboratory.
Wall Street News noticed that UniAD's ability to solve multi-task application requirements may have an inherent connection with the multi-modal multi-task universal large model "Shusheng (INTERN) 2.5" released by SenseTime Technology on March 14 this year.
"Shusheng (INTERN) 2.5" has "good cross-modal open task processing capabilities for graphics and text, and can provide efficient and accurate perception and understanding support for general scenario tasks such as autonomous driving and robots." Its first-generation version was jointly released by SenseTime Technology, Shanghai Artificial Intelligence Laboratory, Tsinghua University, the Chinese University of Hong Kong, and Shanghai Jiaotong University in November 2021, and has been continuously jointly developed. SenseTime announced that the Intern 2.5 is dedicated to building a multimodal and multitask universal model, which can receive and process inputs of various modalities, and adopt a unified model architecture and parameters to handle various tasks.