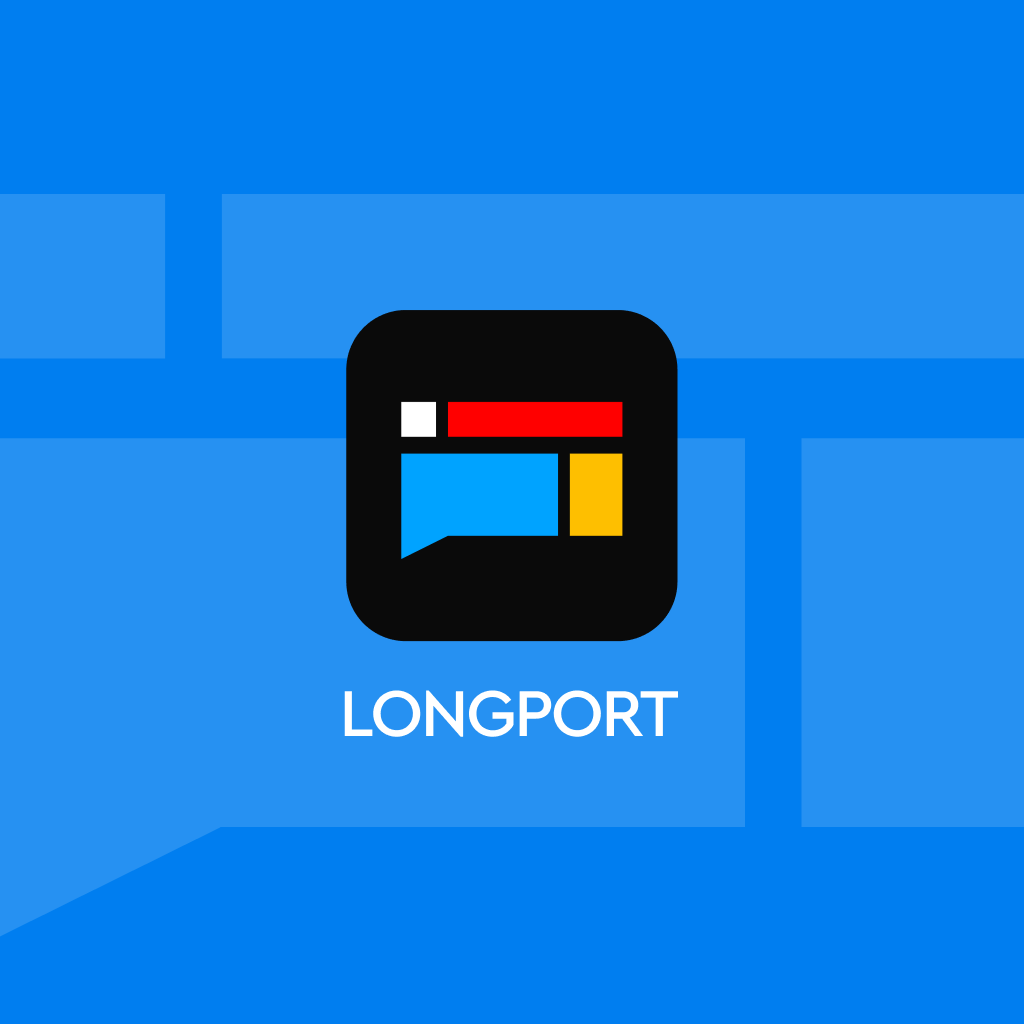
In the past six to eight weeks, the investment logic of AI has undergone a dramatic change!
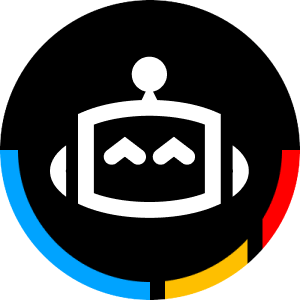
As AI large models shift from pre-training to inference, network architecture, chip cluster layout, and the logic that supports a significant portion of the investment world need to be rethought. Meanwhile, over the past six to eight weeks, during this large model platform period, small teams have continuously emerged, utilizing open-source models to develop new models with relatively little funding, competing in performance with cutting-edge models. In this trend, the limitations of GPUs have also diminished
From pre-training to inference, a key shift is occurring in the field of artificial intelligence, which will disrupt the logic of AI investment.
Recently, in the well-known business podcast Invest Like The Best, Patrick O'Shaughnessy discussed with Benchmark General Partner Chetan Puttagunta and fund manager Modest Proposal topics such as the current scaling challenges faced by AI models, the astonishing popularity of open-source models, and the investment implications for primary and secondary markets.
Due to the depletion of human text data, large model training has shifted to using synthetic data generated by LLMs, but this has failed to continue scaling pre-training, leading AI large models to transition to a new paradigm—shifting from pre-training to test-time compute.
Chetan explained that test-time compute essentially allows large language models to examine problems, come up with a range of possible solutions, and pursue multiple solutions in parallel, while having something called a validator that iteratively processes the solutions.
What does the shift from pre-training to inference mean for AI? This has changed the investment logic of venture capital over the past six to eight weeks.
What does the shift from pre-training to inference really mean?
Chetan emphasized two key challenges of the test-time inference paradigm: First, the algorithms used for test-time compute may quickly exhaust the useful search space of solutions; second, the validator's ability to distinguish between good and bad solutions and find the optimal path is uncertain as to whether it can grow linearly with the unlimited expansion of computing power. Additionally, the complexity and ambiguity of the tasks themselves mean that limiting factors may not solely be computing power.
Despite these challenges, Chetan remains optimistic about addressing these issues through improvements in algorithms, data, hardware, and optimization.
Modest analyzed several significant impacts of the shift from pre-training to inference time from a micro perspective. First, it can better align revenue generation with expenditure:
I think this is a very, very beneficial outcome for the entire industry, as that was not the case in the pre-training space. Spending $20 billion, $30 billion, or $40 billion on capital expenditures to train models over 9 to 12 months, doing post-training work, then rolling it out, and hoping to generate revenue from inference. In a world where test-time compute scales, you are now aligning your expenditure with the underlying usage of the model. Therefore, in terms of pure efficiency and financial scalability, this is much better for hyper-scale enterprises.
Modest believes the second significant impact is that if we indeed see a shift towards inference time, many narratives that support a large part of the investment world need to be rethought in terms of network architecture, chip cluster layout, and so on:
Do we need to start thinking about how to redesign the network architecture? Should we establish millions of chip superclusters on land with low energy costs, or should we distribute smaller, lower-latency, more efficient inference-time data centers across the country? And when you redesign the network architecture, what is the impact on power utilization and grid design?
I want to say that many narratives that support a large part of the investment world need to be rethought. Moreover, I must say that, as this is a relatively new phenomenon, the public markets have not yet begun to address what this potential new architecture might look like and how it could affect some potential expenditures.
In the past six to eight weeks, small models have changed venture capital thinking
Chetan and Modest are uncertain whether pre-training will make a comeback, but the current bottleneck means that small teams have the opportunity to showcase innovation in specific areas.
Chetan observed that in the past six to eight weeks, there has been a continuous emergence of small teams (2-5 people) developing new models with relatively little funding, competing in performance with cutting-edge models, a phenomenon not seen in the past two years.
Chetan pointed out that open-source models, especially Meta's LLaMA series, have enabled small teams to quickly catch up with the technological frontier without massive investments. Small teams can reach the technological frontier quickly and at a lower cost by downloading, deploying, and optimizing open-source models. Since they do not require large amounts of computational resources or data, small teams can rapidly demonstrate innovation in specific areas and quickly reach the technological frontier. Once small teams reach the technological frontier, they can establish partnerships with large service providers (such as AWS).
In this trend, the limitations of GPUs have also decreased. Compared to 2022, the limitations of GPU resources for teams at the technological frontier are no longer as severe, especially in terms of inference and computation during testing.
This has also led to a shift in venture capital, moving from once avoiding capital-intensive large model training and focusing on application investments to beginning to pay attention to the innovations of small model teams that are more flexible and capital-efficient. Chetan stated:
The venture capital model has always been about whether you can assemble an extraordinary team, achieve technological breakthroughs, become capital-light, rapidly surpass existing companies, and then somehow gain a distribution foothold and advance. In terms of the model over the past two years, this seemed impossible to achieve. But in the past six to eight weeks, this situation has indeed changed.
Here are some key points and highlights from the discussion:
-
In terms of testing time or inference examples, two things will soon become apparent. First, large language models (LLMs) will very quickly explore the space of potential solutions , and the algorithms for testing time computation may quickly exhaust the useful solution search space. Second, there is something called a validator that is examining which might be good solutions, which might be bad solutions, and what should be pursued.
-
On a micro level, there are several significant impacts of shifting from pre-training to inference time. Firstly, it allows for better alignment of revenue generation with expenditures. In a world where testing time computation is scaled, you are now aligning your expenditures with the underlying usage of the model Therefore, in terms of pure efficiency and financial scalability, this is much better for ultra-large enterprises.
-
The second major impact is that the shift towards inference time requires rethinking how to redesign network architecture. Should we establish millions of chip superclusters on land with low energy costs, or should we deploy smaller, lower-latency, more efficient inference time data centers nationwide? And what is the impact on power utilization and grid design when redesigning network architecture?
-
In the current era of large model platforms, small teams are starting to catch up with cutting-edge models. Teams of only two to five people can catch up with the forefront at a cost far lower than that of large laboratories. Part of the reason is the astonishing surge in the number of open-source models. Specifically, Meta's work on LLaMA has had a huge impact here.
-
You don't need a lot of computing resources, or you don't need a lot of data, to demonstrate exceptional intelligence and innovation in specific verticals, specific technologies, or specific use cases, allowing you to rapidly leap to the forefront. I believe this has largely changed my personal view on the model layer and the potential early investments in the model layer.
-
For teams at the technological forefront, they are no longer particularly constrained by GPU resources, especially when pursuing inference or computation during testing. Compared to 2022, the challenges in computing are no longer as severe, especially for teams serving a small number of enterprise clients or optimizing consumer solutions for specific use cases.
-
By 2025, we will be very close to or reach the level of general artificial intelligence. Given the current progress and innovation, coupled with the shift towards testing during computation and inference, from this perspective, general artificial intelligence is on the horizon.
-
If OpenAI chooses to claim that it has achieved general artificial intelligence, I believe this will create a very interesting dynamic between them and Microsoft, intensifying the already interesting dynamics that are currently at play. So, this will definitely be worth watching next year, not only for public market investors but also for its impact on the broader ecosystem.
-
Six to nine months ago, there were significant concerns about incremental capital. However, the perspective drawn from third-quarter data is that there are application scenarios. Inference is underway. Technology is playing its rightful role, the cost of inference is plummeting, and utilization is skyrocketing. Combining these two, you get a steadily growing substantial revenue, and everything is going well.
-
One of the things happening in the private market is the sharp decline in computing prices, whether for inference or training, or anything else, as it becomes increasingly accessible. If you are sitting here today as an application developer, the inference costs of these models have dropped by 100 times or 200 times compared to two years ago. Frankly, this is outrageous. I've never seen a cost curve look so steep and so fast.
The following is the full conversation:
Patrick
Today my guests are Chetan Puttagunta and Modest Proposal. If you are as obsessed with the cutting edge of artificial intelligence and its impact on business and investment as I am, you will definitely enjoy this conversation. Chetan is a general partner and investor at Benchmark Capital, while Modest Proposal is an anonymous investor managing a large amount of capital in the public markets. Both are good friends of mine and regulars on this show, but this is their first time appearing together.
The timing couldn't be better. As top labs reach the limits of scale and shift from pre-training to testing computations, we may be witnessing a critical shift in the development of artificial intelligence. We discuss how this change could democratize AI development while reshaping the investment landscape in both public and private markets. Please enjoy this engaging discussion with my friends Chetan Puttagunta and Modest Proposal.
The state of LLMs and their scale
Patrick
So, Chetan, perhaps you can start by telling us from your perspective what the most interesting aspect of the story around large language models and their scaling is at the moment.
Chetan
Yes, I think we are at a stage where there is a general consensus or awareness that all labs have encountered some sort of leveling effect in how they view scaling over the past two years, particularly in the realm of pre-training. According to the laws of scaling, the more computational power you add in pre-training, the better the resulting model becomes. Everything is considered in orders of magnitude. So, increasing computational power by a factor of 10 on this issue will lead to a step-function improvement in model performance and intelligence.
This has undoubtedly brought incredible breakthroughs here, and what we see from all labs are very impressive models. But the shadow of all this has been looming since the end of 2022, at some point we will run out of human-generated text data.
And we are soon entering the world of synthetic data. All the knowledge in the world has actually been tokenized and absorbed by these models. Of course, there are niche data, private data, and all these small repositories that have yet to be tokenized, but in terms of orders of magnitude, this will not significantly increase the amount of usable data for these models.
Looking ahead to 2022, you saw whether synthetic data could allow these models to continue scaling as a major question.
As you can see from that line, everyone believes this question will really come to the forefront in 2024. And now we are here, we are in the present, and large model providers are struggling to train with synthetic data. And now, as reported by the media, and as all these AI lab leaders have publicly stated, we are encountering limitations due to synthetic data The synthetic data generated by large language models themselves cannot sustain the scale expansion during pre-training. Therefore, we now turn to a new paradigm known as test-time computation. At a very basic level, test-time computation is when you actually have the large language model examine the problem, come up with a series of possible solutions, and pursue multiple solutions in parallel. You create something called a validator, iteratively processing the solutions, and this new scaling paradigm can be said to measure time on a logarithmic scale on the X-axis and intelligence on the Y-axis.
This is our current situation, where it seems almost everyone is moving towards a world where we evolve from pre-training and training-based scaling to what is now referred to as reasoning-based scaling, or reasoning time, test time, however you want to call it. This is our status as of the fourth quarter of 2024.
Patrick
This is a follow-up question about the overall situation. So, putting aside capital expenditures and all the other issues we will discuss later with large public tech companies, based on what you know now, can we say that the shift to time-variable test-time scaling is like "who cares?" As long as these things become increasingly powerful, isn't that the most important thing? And how does the fact that we are doing this in a way that is different from merely being pre-training based matter? Does anyone really care? Is it important?
Chetan
In the test-time or reasoning paradigm, two things will quickly stand out: large language models (LLMs) will rapidly explore the space of potential solutions. As model developers or those working with models, it will soon become apparent that the algorithms used for test-time computation may quickly exhaust the useful solution search space. This is the first point.
The second point is that there is something called a validator that is examining which might be good solutions, which might be bad solutions, what should be pursued, and is able to discern which are good solutions, which are bad solutions, or which are the best paths and which are not. It is currently unclear whether this will scale linearly with the infinite enhancement of computational power. Ultimately, the task itself may be complex and ambiguous, and the limiting factor may or may not be computational power.
So, thinking about these questions is always very interesting, as if you had infinite computational power to solve this problem, could you do it faster? Certainly, in reasoning, there will definitely be some issues if you can just scale up computation to do it faster. But many times, we are starting to see evidence that, with the technology we have today, this is not necessarily something that can scale linearly with computation.
Can we solve all these problems now? Of course, there will be improvements in algorithms, improvements in data, improvements in hardware, and various optimization improvements here. What we are still discovering is that the inherent knowledge or available data possessed by the foundational models for reasoning is still limited. Just because you are pursuing test time does not mean you can break through all previous data limitations by scaling computation at test time So, this doesn't mean that we have encountered obstacles in reasoning, nor does it mean that we have faced challenges in testing time. It's just that the problem set, challenges, and computer science issues are beginning to evolve. As a venture capitalist, I am very optimistic and believe that we can solve all these problems. But they are solvable.
Macro Perspective
Patrick
So, if this is the perspective of the research lab, Modest, I'm curious if you can tell us about the pessimistic views of large public tech companies, because much of the discussion on this topic revolves around capital expenditure, strategic positioning, the so-called return on investment for all these expenditures, and how they will gain returns from this massive capital outlay. Do you think everything Chetan just mentioned is well reflected in the stance, pricing, and valuation of public tech companies?
Modest
I think you have to start from a macro level and then delve into the micro level. Why is this important? Because everyone knows that large tech companies now account for a larger proportion of the S&P 500. But beyond that, I think thematically, artificial intelligence has penetrated more broadly into industries and utilities, and I believe that as a direct investment in this field, its market capitalization accounts for between 40% and 45%.
Moreover, if you even extend it to other parts of the world, you would involve ASML, TSMC, and the entire Japanese chip industry.
So, if you look at the cumulative market capitalization, this is a massive direct investment in artificial intelligence right now. Therefore, I think when you examine the entire investment landscape, you are almost forced to form an opinion on this because almost everyone will compare themselves to some index, and that index will be a derivative investment in artificial intelligence at the micro level. I think this is a fascinating time because all public market investments are scenario analyses and probability-weighted on different paths. If you think back to about four months ago when we were discussing this, I would say the distribution of outcomes has changed.
At that time, at that level, pre-training and scaling up was absolutely the way to go. We discussed its implications then. We talked about Pascal's wager and the prisoner's dilemma. To me, it's easy to talk about these things when the stakes are $1 billion or $5 billion. But we are quickly approaching a point where the stakes will be $20 billion or $50 billion. You can look at the cash flow statements of these companies. It's hard to quietly add a $30 billion deal.
Therefore, the overall success of GPT-5 has collapsed. Let's apply this to all the different labs. I think this would have been a significant proof point regarding the amount of capital invested, as these are all three to four-year investment commitments. If you go back to when this article was written, it was discussing Stargate, the hypothetical $100 billion data center discussed by OpenAI and Microsoft, with a delivery commitment in 2028 But at some point in the next six to nine months, it will either work or it won't. We already know that a supercluster of 300,000 to 400,000 chips will be delivered by the end of next year to early 2026. However, we may need to see some evidence of success with the next model to secure the next round of commitments. So, I think all of this is background. On a micro level, if we shift from pre-training to inference time, it will be a very powerful transition with several significant implications.
First, it can better align revenue generation with expenditure. I believe this is a very, very beneficial outcome for the entire industry, as that is not the case in the pre-training domain.
Investing $20 billion, $30 billion, or $40 billion in capital expenditures, training models over 9 to 12 months, doing post-training work, then rolling it out, and hoping to generate revenue from inference. In a world where testing time calculates scaling, you are now aligning your expenditures with the underlying usage of the models. Therefore, in terms of pure efficiency and financial scalability, this is much better for hyperscale enterprises.
I think the second significant implication is that, similarly, we must say that we do not know whether the scaling of pre-training will stop. But if you do see this shift towards inference time, I think you need to start thinking about how to redesign network architecture? Do you need to build millions of chip superclusters on land with low energy costs, or do you need to have a nationwide distributed layout of smaller, lower-latency, more efficient inference time data centers? And when you redesign the network architecture, what are the implications for power utilization and grid design?
I want to say that many narratives that support a large part of the investment world, I think need to be rethought. And I want to say that since this is a relatively new phenomenon, the public markets have not yet begun to address what this potential new architecture looks like and how it might affect some potential expenditures.
Small teams are also building excellent models
Patrick
Chetan, I'm curious if you could talk about DeepSeek and other similar cases, where you see small teams building new models with relatively little funding that compete in performance with some cutting-edge models. Can you discuss this phenomenon and what it makes you think of, or what impact it has on the entire industry?
Chetan
It's truly astonishing. In the past, over the last six weeks or so, we've seen teams at Benchmark with member counts ranging from two to five. Modest has talked about this on your podcast before, that the story of technological innovation has always been that in some garage in Palo Alto, there are always two to three people doing something, rapidly catching up with established companies.
I think we are now seeing this situation at the model level, and frankly, this is something we haven't seen in two years. Specifically, I think we still cannot be 100% certain that a return to pre-training and training scale will not happen We currently do not know. But in this relatively stable period, we are starting to see these small teams catch up to the forefront. By "forefront," I mean where the most advanced models are, especially in text processing. We see these small teams, specifically, only two to five people, leap to the forefront with funding several orders of magnitude lower than that of large laboratories.
I believe part of the reason is the astonishing surge in the number of open-source models. Specifically, Meta's work on LLaMA has had a huge impact here. LLaMA 3.1 has three versions: 405 billion, 70 billion, and 8 billion. LLaMA 3.2 has versions of 1 billion, 3 billion, 11 billion, and 90 billion.
You can access these models, download them, put them on local machines, upload them to the cloud, place them on servers, and you can use these models for refinement, optimization—tuning, training, improving, and so on, and keep up with the forefront with quite interesting algorithmic techniques.
Moreover, because you do not need a lot of computing resources, or you do not need a lot of data, you can demonstrate particular cleverness and innovation in specific verticals, specific technologies, or specific use cases, thus rapidly leaping to the forefront. I think this has largely changed my personal view on the model layer and the potential early investments in the model layer. There is a lot of uncertainty, a lot of dependent variables, and in fact, within six weeks, all of this may no longer hold.
But if this state holds, that pre-training is not expanding due to synthetic data, it simply means you can now do more, jump to the forefront quickly with minimal funding, find your use case, find your strongest points, and then from there, frankly, super giants will become your best friends.
Because today, if you are at the forefront, you are driving a use case, you are no longer particularly limited by GPUs. Especially if you plan to do work like inference at test time, compute at test time, and you are serving, say, 10 enterprise customers. Or perhaps this is a consumer solution optimized for a specific use case.
The challenges in computing are no longer as significant as they were in 2022. In 2022, when you talked to these developers, it became a question like: well, can you piece together a hundred thousand clusters? Because we need to train, and then we have to buy all this data, and even if you know all the technology, suddenly when you calculate, you would say, to start the first training run, I need to spend a billion dollars. And that is not a viable model.
Historically, this has been the venture capital model. The venture capital model has always been about whether you can assemble an extraordinary team, achieve technological breakthroughs, do it in a capital-light manner, quickly surpass existing companies, and then somehow gain a foothold in distribution and advance. In the past two years, this seemed utterly impossible. But in the past six to eight weeks, this situation has indeed changed Modest
I think this is very important. Regarding Meta's open source and the push for open source by large-scale companies, small models can scale to a very successful extent, which is extremely beneficial, especially for AWS, which does not have a native large language model. But if you take a step back and think about the history of cloud computing, it has provided developers and builders with a set of tools. AWS was the first to clearly articulate this vision.
In September, at a conference at Goldman Sachs, Matt Garman publicly talked about this. But their point has clearly always been that large language models are just another tool, and generative artificial intelligence is another tool they can provide to their enterprise and developer customers to build the next generation of products. The risk this vision faces is an omnipotent and ubiquitous mob.
So, this again makes you rethink what would happen if we do not build these large-scale pre-trained entities, reduce training loss to nearly zero, and construct that metaphorical god in one form or another.
On the contrary, if the industry's focus is on the testing phase, the inference phase, and trying to solve real problems where customers need it, I think this redesigns and reconstructs the entire vision of how this technology is launched. And I think we need humility because we do not know what LLaMA 4 will look like. We do not know what Grok 3 is about to launch. These are two models being trained on the largest clusters ever.
So everything we are saying now may be wrong in three months. But I think the whole work right now is to absorb all available information and redraw various scenario paths based on what we know today. If this is correct, I feel that people have not updated their prior judgments about how these paths might develop.
Patrick
I am curious, Chetan, about this change. Perhaps now you would invest in a model company; what do you think? I remember two years ago when we had dinner together, you told me that as a company, you had just decided not to invest in these companies. As you said, it does not fit our model. We will not write checks for billions of dollars at the first trial run.
Therefore, we do not invest in that part of the stack. We invest more in the application layer, and we will return to this topic later in this discussion. But perhaps talk more about this updated perspective on how it works, what a sample investment might look like, and whether this would change even if LLaMA 4 maintains the pre-training scaling loss, as it seems to benefit just like DeepSeek. Well, it is not 3.2 now, but 4, and we are still doing our thing, and still better, cheaper, faster, and so on.
So, yes, regarding this new perspective that it is possible to invest in model companies, not just application companies, what are your thoughts?
Chetan
In Meta's last earnings call, Mark Zuckerberg talked about their beginning to develop LLaMA 4 and mentioned that LLaMA 4 is being trained on a cluster larger than any he has seen before The cited figures indicate that it is more powerful than 100,000 H100s, or stronger than anything else I have seen other companies doing. And he also mentioned that the smaller Llama 4 model should be ready by early 2025. This is really interesting because it doesn't matter whether Llama 4 is a step function compared to Llama 3; what matters is whether they have broken through the limits of efficiency, achieving even just incremental improvements, which would have a significant impact on the developer community. Nowadays, Llama's influence has two aspects, which I believe is very beneficial for Meta. First, the Transformer architecture used by Llama is a standard architecture, but it has its own nuances.
Moreover, if the entire developer ecosystem built on Llama starts to assume that the Llama 3 transformer architecture is the foundation and a sort of standard practice, it would be akin to standardizing the entire stack towards Llama's way of thinking, from how hardware vendors support your training runs to the super giants, and so on. Therefore, the standardization of Llama itself is becoming increasingly common.
So, if you were to start a new model company, the end result is that starting from Llama today is not only great because Llama is open-source, but also extremely efficient because the entire ecosystem is adopting this architecture. So you are right, as an early fund with $500 million in capital, we try to make 30 investments in each fund cycle, and a $1 billion pilot essentially means you are putting in two rounds of funding for a pilot that may or may not succeed.
Thus, this is a capital-intensive business. By the way, the depreciation schedule for these models is daunting. Distillation as a technique makes the defensibility of these models and their annotations extremely challenging. It really comes down to what applications you are building on top of it, what your network effects are, how you achieve economic benefits there, and so on.
I think, for the time being, if you are a team of two to five people, you can take programming as an example, by fine-tuning training based on Llama to push for the development of a model that generates better programming answers faster, and then provide an application that includes your own customized model, which can indeed deliver extraordinary results for your clients, whether they are developers or others in similar roles. So, our specific approach and strategy here have always been to invest heavily in applications since we saw the OpenAI API start to gain popularity.
In the summer of 2022, we began to see developers talking about these OpenAI APIs. Since then, much of our effort has been to find those entrepreneurs considering leveraging these APIs to explore the application layer and really start thinking about what applications could not possibly exist before this current wave of artificial intelligence Clearly, we have seen some outstanding successful companies emerge, which are still in their early stages, but the momentum they exhibit, the customer experience they provide, the biometric technologies they adopt, and so on, are extraordinary. A few weeks ago, Brett Taylor mentioned in your podcast that Sierra is one such example. In procurement, we have a project called Levelpath. Throughout the portfolio, there are many other examples at the application layer, where you can examine every major SaaS market, invest in the application layer to expand it, and start thinking about what things are now possible that were not achievable two, three, or four years ago.
In-depth Exploration of Foundational Models and Key Players
Patrick
I'm curious to talk a bit about those major foundational model players we mentioned, like Llama, but I don't want to discuss xAI, Anthropic, and OpenAI, perhaps also Meta. Let's start with you; I'm curious about your thoughts on their strategic positioning and the important aspects of each.
Perhaps using OpenAI as an example, maybe the key here is how outstanding a brand they have built, they have a large user base, they have many excellent partners, people know and use their products, and many pay for them, like $20 or so. Perhaps in this model, the distribution channel is more important than the product itself.
I'm curious about your views on these three players, who have dominated so far, but through your current analysis, it seems important for them to continue innovating.
Modest
So I think the interesting part for OpenAI is that they just completed their latest round of financing, and there have been some quite public comments regarding the reasons for investment. Indeed, many comments revolve around the idea that they have achieved escape velocity on the consumer side, with ChatGPT now being a cognitive reference. Over time, they will be able to gather huge consumer demand and charge appropriately for it, while their investments in enterprise APIs and application building are much less.
If you think carefully about what we are discussing, it becomes super interesting.
In their financial data, if you exclude training costs, if you exclude this huge upfront expenditure requirement, according to their forecasts, it will actually soon become a profitable company. So in a sense, this might be better.
Now the question becomes, how defensible is a company that is no longer making leapfrog advances in the frontier field? In this regard, I think it ultimately comes down to one point: Google is also advancing at the frontier, and they are likely to give away products for free, as well as Meta. I think we could perhaps spend an entire episode discussing Meta and their embedded choices for enterprises and consumers. But let's first talk about the consumer side This is a company with over 3 billion consumer touchpoints. They are clearly applying Meta AI in various scenarios. It’s not hard to see that they should acquire Perplexity.
But you just saw the Department of Justice step in and say that Google should be forced to license its search index. I can’t think of anyone more profitable than Meta, which has the opportunity to take over Google’s search index at a very low cost. But the key is, I believe there will be two large-scale internet giants offering essentially similar products to ChatGPT for free. So this will be an intriguing case study exploring “can this product dominate in the minds of consumers?”
My kids know what ChatGPT is; they don’t know what Claude is. My family knows what ChatGPT is; they don’t know what Grok is. So I think the question for OpenAI is, can you go beyond free? If you can, and if training costs decrease, this will be a company that can quickly become profitable.
If you look into Anthropic, I think they face an interesting dilemma where people believe Sonnet 3.5 might be the best existing model. They have incredible technical talent. They are continuously attracting more and more OpenAI researchers, and I believe they will build excellent models, but they are somewhat stuck. Their brand recognition among consumers is low.
In terms of enterprise, I think Llama will make it difficult for cutting-edge model builders to create significant value there. So they are stuck in the middle. Excellent technical experts, quality products, but not a truly viable strategy. And look, they just raised $4 billion.
To me, this indicates that the scaling effect of pre-training is not very good, as $4 billion falls far short of their needs. If the path to scaling is pre-training, then I have no good judgment about their future strategic path. I think they are in a bind. As for xAI, I’ll just pretend I don’t know.
He is a unique talent, and they will have a 200,000 chip cluster, and they have a consumer-facing touchpoint, and they are building an application programming interface (API). But I think if pre-training is the path to scaling, then they will face the same mathematical challenges as everyone else, albeit possibly alleviated by Elon’s unique fundraising ability.
But again, in the next four to five years, the numbers will rapidly become so large that they might even surpass him. Then, if it comes to the testing phase, where are the differences in computation, algorithm improvements, and reasoning? What are their market entry points when you have someone firmly established on the consumer side, and on the enterprise side, there is an equally powerful open-source entity?
So when you examine these three, I think the easiest thing to see is what the future direction of OpenAI is However, regarding OpenAI, I want to mention Noam Brown. I found him to be one of the most outstanding communicators in the research field. He recently appeared on a podcast by Sequoia Capital, and when asked about general artificial intelligence, he said, "You see, I think when I'm outside of OpenAI, I have a skeptical attitude towards the whole general artificial intelligence thing. But in reality, this is what they are focused on."
When I joined OpenAI, I was very clear that they take general artificial intelligence (AGI) very seriously; it is their mission, and everything else serves AGI. It's easy for us to sit outside and articulate the strategies we might take if we were in charge there, but I think we need to recognize the fact that part of the reason they have come this far is that they carry a mission.
The task is to develop general artificial intelligence, and we should be very cautious about setting any other ultimate goals for it.
Chetan
And my personal view is that general artificial intelligence is very close to being realized.
Patrick
Let me add a few more words. So why isn't it here yet? These things are smarter than most of the people I deal with.
Chetan
Yes, I think so. Narrowly defined artificial general intelligence (AGI), or perhaps from a broader definition perspective, depending on your viewpoint, is a highly autonomous system that, in certain cases, surpasses human performance in economically valuable tasks. From this perspective, it's easy to say AGI already exists. I think it's very clear that if you look at the announcements OpenAI has made and the interviews their executives have given in recent weeks, one example is end-to-end travel booking, which is something we can expect to see in 2025, where you can prompt the system to book travel for you, and then it will go do it.
This is a new way of thinking about end-to-end task completion or end-to-end work completion. This clearly involves reasoning, involves autonomous work, involves using computers, as Claude has articulated. And you are combining these large language models with various ways of interacting with the ecosystem itself, putting it into a very impressive combination that can accomplish end-to-end work and do it better than humans. From my perspective, we are very, very close from this angle.
And I envision that by 2025, we will be very close to or at the level of general artificial intelligence. Given the current progress and innovation, coupled with the shift towards testing in terms of computation and reasoning, from this perspective, general artificial intelligence is on the horizon.
Modest
This is interesting because we are somewhat like frogs being boiled in water; we have quite easily passed the Turing test, yet no one is sitting here talking about, "Oh my gosh, we passed the Turing test." It came and went. So perhaps the announcement of general artificial intelligence is the same thing, like, "Yes, of course, the model can book end-to-end travel." It's actually not that hard However, two and a half years ago, if you said, "Hey, there's an algorithm that you can tell what you want to do, and it will arrange everything from start to finish and send you a receipt," you would have said, "No way." So this might be a bit like boiling a frog; suddenly one day you wake up, and a lab says, "Hey, we have achieved general artificial intelligence." Everyone might be like, "Oh, cool." However, the announcement of achieving general artificial intelligence by the lab is interesting in a broader sense for a special reason, which is the relationship with Microsoft. Microsoft first disclosed last summer that they have full rights to the intellectual property of OpenAI before the achievement of general artificial intelligence.
So, if OpenAI chooses to claim that it has achieved general artificial intelligence, I think this will trigger a very interesting dynamic between them and Microsoft, which will intensify the already interesting dynamics that are currently at play. Therefore, this is definitely worth watching next year, not only for public market investors but also for its impact on the broader ecosystem. Because I again believe that if the path we are on now is correct, then as we move forward, there will be a lot of reshuffling of relationships and business partnerships.
Patrick
Chetan, is there anything else in Modest's assessment of big companies? And given that we haven't specifically talked about Google, we would love to hear your thoughts on Google. Is there anything he said that you disagree with or want to follow up on?
Chetan
No, I think what we just don't know is the potential discussions happening in all these rooms; we can speculate and understand what we might do. But I think, ultimately, every internet company or tech company boils down to two scenarios.
On the consumer side, distribution then combines with some kind of network effect and lock-in effect, and then you can stand out and gain an advantage in competition. On the enterprise side, this is largely driven by technological differentiation and a business model that delivers excellent service level agreements, high-quality service, and very unique solution delivery methods. So, Modest's comments on consumers and how consumers will evolve.
I think that is completely correct. Meta, Google, and XAI all have consumer touchpoints. OpenAI today has an excellent brand, thanks to ChatGPT and a multitude of consumer touchpoints. On the enterprise side, the challenge is that these APIs have largely not been as reliable as developers expect.
Due to the excellent work of hyperscale cloud service providers, developers have become accustomed to the idea that if you provide an API for a product, that product should be infinitely scalable, available 24/7, and the only reason for an API to fail is something like a power outage at a large data center. There are few reasons for an API to fail. This has become the mindset of developers regarding enterprise solutions. Over the past two years, the quality of AI APIs has been a huge challenge for application developers Therefore, the final result is that people have found workarounds and solved all these problems through pure innovation. But continuing to push forward in this regard, we come back to this point again. If pre-training and scaling are not the solution, and it completely depends on the computational power at the time of testing, this is where we return to the traditional ways of the hyperscale operators. I believe AWS has a significant advantage in this regard, as both Azure and Google have excellent cloud services, but AWS has the largest cloud.
It has indeed built resilience in a very unique way. Even today, if you run the LLaMA model, you would want to run the LLaMA model on AWS, or for some specific use cases where you need to support local customers, you can also run these models locally if you wish.
In large financial institutions where regulatory environments are complex or there are compliance reasons, you can run these models locally if you want.
Moreover, AWS has even taken action in this regard, such as VPC (Virtual Private Cloud), GovCloud, and things like that. So, if we assume that pre-training and scaling work has been completed, then suddenly, AWS becomes extremely powerful. Over the past few years, their strategy has been to befriend everyone in the developer ecosystem rather than working on large language models on their own.
Well, they are making progress, but not in the same way as other companies, which is likely to become quite a good strategy because suddenly you have the best API services. I think another part is Google, which we haven't talked about yet; their cloud is excellent in some ways. So they have enterprise business. If you look at the latest earnings report, you'll find that their enterprise business has actually scaled quite significantly. Obviously, their consumer business dominates, and there has always been a perception that they are currently under pressure.
I think these forces are very disruptive to them. But it is still unclear whether this disruption has already occurred. What actions have they taken in response? Clearly, they are trying hard, and it is evident that they are making a significant effort.
But I think this is something worth paying attention to, and it is the kind of thing I like because it is a typical innovator's dilemma. Obviously, as an existing enterprise, they are trying hard to stand on the advantageous side of not being replaced by innovators. They are working very hard. So in business history, it is quite rare for existing enterprises to successfully fend off attacks from innovators.
And if they do defend their business in this era, it would be an extraordinary achievement.
Modest
Yes, Google is very intriguing because there was an outstanding sell-side analyst, Carlos Kirjner, who unfortunately passed away. But in 2015 and 2016, he spent a lot of reports writing about Google's progress in artificial intelligence and the foundational work they did at DeepMind. He actually loved this work and eventually went to work at Google, but he first revealed the idea of the foundational work they did in neural networks and deep learning Clearly, they are shocked by this large-scale violent expansion, driven by substantial computational investment. But if you have read any interviews with those who foresaw this data wall, one point they mention is that self-play might be a pattern to overcome data scarcity. And who is better at self-play than DeepMind?
If you examine the achievements DeepMind had before the emergence of Transformers, and the results they achieved by combining Transformers with expanded computational power, it seems they already have all the conditions to win. But the question I have always posed is not whether Google can win in the field of artificial intelligence, but rather, regardless of what winning looks like, is it possible to replicate the glory of winning in the current paradigm? That is the real issue.
As Chetan said, if they can overcome the difficulties and achieve victory, it would be astonishing, but I believe they have the conditions to do so. The real question is whether they can build a business with their existing assets that can be as outstanding in any aspect as what could be considered the greatest business model we have seen—internet search. So I am equally looking forward to following them. I think in terms of business, they have incredible models and assets.
I think they need to earn a lot of trust. I feel that over time, they have had ups and downs in that world, so I think this is a more challenging aspect for them to break through. But in terms of consumers, certainly in model building, they already have all the winning conditions.
The question is, what does that prize look like? Especially now, if it seems that there are not one or two models that can dominate.
Investors' Views on the Application Layer
Patrick
Chetan, I am curious, as an investor seeking returns, what path do you personally hope to take?
Chetan
Personally, I hope artificial intelligence can last a long time. As a venture capitalist, you need massive disruption to unlock distribution. If you look at what has happened in the internet or mobile space, and where value is generated, in both of these waves, value has primarily been generated at the application layer. Clearly, our hypothesis, and my hypothesis, is that this layer will once again be very favorable for unlocking distribution due to innovations in the artificial intelligence application layer. I think this has largely manifested itself so far. Although still in the early stages, those vendors launching AI application products for consumers and enterprises have found that these solutions exist entirely because of artificial intelligence. They are unlocking distribution channels in a way that frankly cannot be achieved in areas like Software as a Service (SaaS) or consumer-facing SaaS.
We will give you a very specific example, an AI-driven application. We are now showcasing these demonstrations to the Chief Information Officers of Fortune 500 companies. Two years ago, there were indeed some good demonstrations. Today, this is an outstanding demonstration, which also incorporates five customer reference cases, all of whom are peers that have used it in production and achieved tremendous success During that communication, one thing became very clear: what we are demonstrating is not a 5% improvement on existing SaaS solutions. Rather, it is about our ability to significantly reduce software spending and human capital expenditure and shift that to this artificial intelligence solution. Moreover, your definition of a 10x traditional return on investment for software is easily recognized, and people can understand it within 30 minutes.
So you start to see that in the past, software as a service (SaaS) and AI applications typically had very long sales cycles, but now decisions can be made in 15 minutes, and 30 minutes can lead to a decision. Furthermore, for enterprises, the procurement process is completely unstable. Now, Chief Information Officers say things like, "Let's get this done as soon as possible." We plan to conduct a 30-day pilot. Once successful, we will sign a contract and deploy immediately. Such situations were completely impossible in the SaaS space three or four years ago because back then, you were competing with established companies, competing against their distribution advantages, service advantages, and all that sort of thing. It was also difficult to prove that your specific product was unique.
So, since 2022, I can say that since the launch of ChatGPT in November 2022, this seems to be a very clear before-and-after boundary in this world. We have made 25 investments in AI companies, which is an extraordinary pace for a $500 million fund made up of five partners. The last time we reached this pace was during the launch of the App Store in 2009. Then, we reached this pace again during the internet era in 1995 and 1996. Between these two periods, you can see that our investment pace was quite slow.
During non-disruptive periods, we typically invested about five to seven times a year. Clearly, our investment pace has significantly accelerated now. If you look at these 25 companies, four are infrastructure companies, and the rest are application companies. We have just invested in our first model company, although this has not been announced yet.
But these are two individuals, two extraordinary and talented people, who have ventured into the frontier with very little funding. So, we have clearly bet and anticipated that there will be significant innovation and distribution unlocking at the application layer. We are already seeing this happening. As software investors, these products are indeed amazing.
They require a complete rethinking of how these things are architected, starting from first principles. You need a unified data layer, new infrastructure, new user interfaces, and things like that. Clearly, startups have a significant advantage over established software vendors. This is not to say that established software vendors are stagnant; it’s just that today, in the enterprise software space, the innovator's dilemma is playing out more intensely before our eyes than in the consumer space.
I believe that in the consumer space, consumer participants have realized this, are driving change, and are taking action. However, I think in the enterprise space, even if you realize this, even if you have the desire to take action, the way solutions are built cannot respond to significant restructuring of architecture So, can we see this situation happen? Will a large SaaS company pause sales for two years and then completely restructure its application stack?
Of course, but I just feel that this won't happen. So, if you look at any analysis of what's happening with artificial intelligence software spending, for instance, pure spending has increased eightfold year-over-year between 2023 and 2024. In just one year, it has grown from hundreds of millions to far exceeding one billion dollars. You can see this pull, you can feel this pull.
If you are in any of these AI application companies, you will find that these companies are more constrained by supply than by demand. We talk to the CEOs of these application companies, and they just say things like, "Well, from what I can see, I see the demand." I just don't have the capacity to serve everyone who expresses agreement with me. So I plan to segment it and go to where they are.
As an investor, what I hope is that this situation can continue, and we can maintain stability so that we can focus on these aspects. Frankly, the stability of the model layer is a huge boon for the application layer, mainly because as an application developer, you sit there watching the model layer achieve leaps every year.
And to some extent, you don't know what to build and which things should be waited on because clearly, you want it to align perfectly with the model layer, as the model layer is now shifting towards inference. This is a great place for application developers.
As an application developer, one thing you know is that humans are impatient. Therefore, you need to always build solutions optimized for performance and quality. As an application developer, you can't tell users, for example, that I'm going to provide a high-quality response.
Patrick
In 30 minutes...
Chetan
It just takes longer. This is not a compelling argument. Now, for certain use cases in those situations, is it feasible? Can you have it running in the background 24 hours? Of course.
But these use cases are not common and dominant, and people are also less willing to purchase such things. So, if as an application developer, in the past few weeks, all my board meetings have been these companies saying, in this new inference model, we are very confident in investing in these four things, and for the past year and a half, we have been very hesitant about these investments, but now we are going all in, and our systems will bring huge performance improvements.
Patrick
Sorry, why is that? Why would inference boost their confidence, as if explaining the reasons clearly?
Chetan
Well, if you are an application developer, you are looking at today's models and saying, "I can clearly see that my use case can gain efficiency from this, but I have to invest in these five infrastructure layers and these user interface things. But if a new model comes out in six months, just because the model itself can do it, it renders all those investments moot, so why should I invest in those things? "I just plan to wait for the model to complete and then use that as a basis. But in this reasoning example, if all laboratories pursue reasoning, and reasoning is intelligence on the y-axis while time is on the x-axis, this is our direction of development. Therefore, any improvements I make in my own tools, whether due to the way I algorithmically provide reasoning or my ability to acquire and process data, etc., which significantly shortens reasoning time, I should invest in now.
If reasoning is now the new paradigm, and delivering the last mile for these reasoning models at the application layer means I am building technology and using tools that model companies are unlikely to build, then as these reasoning systems continue to improve, my last mile advantage and last mile delivery system still have advantages and defensibility.
Patrick
Besides programming and customer service, do you both have any other favorite examples? These two seem to be the main and incredibly exciting and cool use cases that many companies are chasing after. Do you have any other favorite examples that would fit a CIO of any company in Fortune magazine saying, 'We need this right now'?
Modest
Chetan loves all his children, so he can't give you specific examples.
Chetan
I can give you 20.
Patrick
Maybe statements like 'explicitly' are where my problem lies. There’s coding here, and essentially top-down support.
Chetan
Look at the biggest spending in enterprise software; you can address it with an AI-driven, AI-first solution. So we have a great company called 11X that is dedicated to sales automation. We also have a great company called Leya that lawyers are using to significantly improve efficiency. I think law has always been a very interesting issue because people think lawyers work on a billable hour basis.
If you are automating billable hours, wouldn't the economics change? Well, two years later, there is evidence that lawyers have significantly increased profits by using AI. The reason is that many mechanical, repetitive, and difficult tasks that were previously done by junior staff within law firms could not be billed anyway. So if you can reduce the time for document analysis from three or four days to 24 hours, suddenly you free up all the lawyers to do all the strategic work that can be billed and the work that is highly valuable to clients.
For example, we have a company that is automating accounting and financial modeling. We have a company that is changing the way game development operates. Someone is getting into the field of circuit board design, which has traditionally been extremely manual and labor-intensive, and computer systems excel in this area. Recently, we also invested in a project targeting advertising networks.
This has been a long-untouched area in startups, but it turns out that in the world of AI, matching those with inventory to those who want to advertise is much more efficient. So we invested in a company with a new document processing model that is challenging OpenText When was the last time startups considered OpenText? It's been a long time, and it has been a while since these huge existing SaaS markets were considered open to new startups.
So you have to pursue more niche and vertical businesses. I often joke that, for example, I've seen this, it's the payroll of field staff working in Eastern Europe. This is a SaaS company that you really had to consider in 2019. And now, we are back to large horizontal expenditures, like, hey, here’s an existing enterprise worth over $10 billion, and the market here spends $10 billion annually.
Artificial intelligence can easily make the products here ten times better, faster, and equipped with all the features users expect. And to gain this advantage, a new platform is needed. That’s the significance of this platform.
Modest
Patrick, you asked about the debate on return on investment (ROI), capital expenditures (CapEx), and all of that from the very beginning. When you listen to Chip's remarks, when you listen to other investors in the application layer, when you listen to the remarks from hyperscale enterprises, the most important takeaway from the past three months is that use cases are emerging. Yes, everyone knows programming, everyone knows customer support, but this is really starting to permeate and integrate into a broader ecosystem. And revenue is becoming a reality. The challenge with ROI has always been, well, you put the funds in here and then amortize it over the investment period, but at the same time, you are putting the next round of funding into the next model.
So everyone can make these inferences and say, “Oh my gosh, it’s not just that Microsoft is going to put $85 billion in cash capital expenditures (including leases) in 2025, but what does this mean for 2026, 2027, and 2028?” Because pre-trained models are becoming so large, if... and again, this is a hypothesis, we tend to stabilize and the spending on pre-training decreases, shifting funds to inference. We know this spending is coming, we know the revenue sources from customers are coming.
So it becomes much easier to say that this spending is justified. I think it’s important for people to remember the potential clouds over these companies, which means that regular storage and computing are still growing at double-digit highs. So, some funds need to be allocated to this area. When you are a $100 billion business growing at 18%, you are a $60 billion business growing at 25%.
This is the incremental capital that everyone was very worried about six or nine months ago. My personal takeaway from the third-quarter data is, okay, I get it, there are application scenarios here. Inference is happening. Technology is doing what it’s supposed to do.
The cost of inference is plummeting, and utilization is skyrocketing. Combine these two, and you get a steadily growing substantial revenue, and everything is great. Satya Nadella talked about this. The issue is that you put funds into the model and get returns on inference, but then we are putting funds into the next model If we could start saying, hey, maybe we won't invest this model in the next $50 billion, then the calculation of return on investment would look much better. Regarding your question to Jason about why the stability of the model layer is important, I think Sam Altman gave the right answer six months ago when he said in a podcast that if you are afraid of our next model release, we will roll over you. If you are looking forward to our next model launch, then you are in a favorable position.
Well, if the reality is that the next model will be used for inference rather than retraining, then you might not have to worry too much about them going out of control. So I think, Pat, everything we are talking about here is very beneficial for the entire ecosystem to form a favorable economic reality, which is why all the attention capital is invested in inference. What is really concerning is whether we need to spend $50 billion, $100 billion, or $200 billion to build these more precise models in pre-training.
What Primary Market Valuations Reveal to Us
Patrick
In what areas do prices best reflect extreme optimism or speculation? Of course, I have seen quite a few private market companies, say Series A type companies, that have extremely high valuations. They often have incredible teams and are very exciting, but in their fields, if something works, you can imagine there will be many other very smart investors funding some competitors. So you see situations like great teams, high prices, high potential competition, which are very exciting, and everything is developing rapidly.
I am curious about what signals you both are reading from valuations and price-to-earnings ratios now.
Chetan
One of the things happening in the private market is the sharp decline in the cost of computing prices, whether for inference or training, etc., because computing has become more accessible. If you are sitting here today as an application developer, compared to two years ago, the inference costs of these models have decreased by a hundred times, two hundred times. Frankly, it's outrageous. You have never seen a cost curve so steep and declining so quickly.
This conclusion is based on a 15-year cloud cost curve, which is already astonishing and incredible. The cost curve of artificial intelligence is completely on a different level. We previously looked at the cost curves of the first wave of application companies we funded in 2022. You see the inference costs, in the latest cutting-edge models, are about $15 to $20 per million tokens.
And nowadays, most companies don't even consider inference costs because it's like, well, we break down this task, and then we use these small models for these fairly basic tasks, while our cutting-edge models mostly handle this very small number of prompts. The rest, we just created this smart routing system. So our inference costs are basically zero. And the gross margin for this task is 95% All you have to do is look at this, and you'll think, wow, the way we consider the growth profit margins of application is completely different from what we've done for SaaS and basically for software over the past decade or so. So I think this is where you start to focus and think about the entire application stack of these new AI applications. It starts with the people providing the reasoning. It starts with the tools and orchestration layer.
So we have a very popular portfolio company called LangChain, and for the reasoning layer, we have Fireworks. These types of companies are being heavily used by developers. Then all the way up to the applications themselves. I think just the speed of innovation and the speed of commercial success is making private investors very excited.
The attractiveness of model stability is that now we can finally assume that if this works, then all these companies will save quite a bit of money. Because if you don't have to spend a lot on pre-training, if you don't have to spend a lot on reasoning, because most of the hyperscale companies will now provide you with very reliable APIs at such costs. Now is a good time to be in the application development business, and it's a good time to be in the application development stack.
Patrick
Modest, what are your thoughts on valuations?
Modest
I think, overall, you have to start with animal spirits. If you go back to the week before ChatGPT was released, which was in the fall of 2022, the tech industry may have just gone through the most brutal bear market since the dot-com bubble burst. You could say that for median tech stocks, the situation was even worse than during the financial crisis. Some very large growth funds were down 60%, 70%.
You witnessed supergiants laying off employees for the first time. You witnessed cuts in capital expenditures and operating expenses. There was a completely different atmosphere throughout the tech world and even in the public markets. The release of ChatGPT sparked a resurgence of venture spirit, which is a gradual process.
So I think, overall, there is a lot of optimism in the public markets, much of which is related to this theme: we are in a new platform era, and for many different new concepts, the sky is the limit. So if we are right, there is this global pending situation. I think, ultimately, it comes down to understanding what the new paths will look like if capital expenditures and the operating costs of hyperscale operators are more closely linked to revenue generation. If you listen to Amazon Web Services (AWS), one of the interesting things they say is that they consider AWS to be a logistics business.
I don't think anyone outside would look at cloud computing and say, oh yes, that's a logistics business. But their point is essentially that what they have to do is predict demand and build supply over the years to meet that demand.
And over the past 20 years, they have become extremely good at what has happened in the past two years. Last time I talked about how demand surged, impacting a supply that lacked elasticity because you can't increase data center capacity in three weeks. So if they can return to a more predictable demand rhythm, it allows them to look and say, okay, now we know where the revenue is coming from This comes from the testing phase, from Chetan and the products launched by his company. Now we know how to match supply with it. Now it's back to the logistics business. It's not about finding every idle nuclear power plant in the country and trying to get it operational.
So, I don't think this is a land-grabbing behavior, but rather a more reasonable, wise, and orderly approach. In fact, I guess if this path is correct, then this inference will surpass training faster than we imagine, and its effects will be greater than we might expect.
But I think, in network design, this aspect of the path will look very different, and it will have a very significant impact on those who build networks, power networks, and transmit optical signals through networks. And all of this, I believe, has not really begun to manifest in a large part of the probability-weighted distribution of the public market.
And you see, I think most people are overly focused on NVIDIA because they are considered a typical representative in this area, but there are many downstream from NVIDIA who may suffer greater losses because their business is worse. NVIDIA is an excellent company doing excellent things. They just happen to have achieved significant earnings growth. I think the impact of this goes far beyond who is making the cutting-edge GPUs, although I do think there is a question of whether this new paradigm of testing time calculation can greatly increase the level of customization at the chip level, that is, whether we can achieve a higher level of customization if we simply expand based on pre-training.
But I think whenever this issue arises in normal conversations, people are overly focused on NVIDIA. I feel people like to argue about that specific name, but I think in the many derivative applications built on artificial intelligence, the distribution of outcomes has changed, and this has not yet been reflected.
Chetan
I just think it's very important to think about this during the testing period and from the reasoning paradigm at the application layer, that is, how much of your prompts actually use reasoning as a way to respond to those prompts. And yes, as this technology becomes more available and easier to use, application developers will use it much more than they do now.
However, if you just look at the current technology and the amazing things that have already been obtained from the application layer, then what percentage of prompts or queries will use reasoning? It's hard to casually estimate and say that 90% of queries will use reasoning.
It doesn't seem to be that way because, again, your users are not going to wait. Humans are inherently impatient, and you have a solution that is just idling, thinking your users are still there. No matter what field they are in, they are gone. So, yes, there may be certain specific tasks that take a long time to complete and can achieve high precision.
But so far, speed is the most important consideration for these application developers. So, will we have a system that continuously backtracks and reuses all this computing power, and in which market share queries will use this capability? It's hard to imagine that this will be the vast majority of queries. So, at least from the perspective of private markets and early investors, what does this mean? Apart from my own field, it's hard for me to imagine what impact this would have on anything else.
But the implication here is simply that the amount of computation you need during training is far less than during training itself. Training is just a continuous task. You are constantly expanding and fully utilizing all computational capabilities, but it's all happening at the application layer, which is extremely abrupt. You will have some tasks that require immediate large-scale processing, and many times you actually don't need that much.
Therefore, this again proves how excellent the super giants and services like EC2 and S3 are. In this new world, the solutions provided by super giants are indeed fantastic. I think Amazon's training and Google's TPUs are truly outstanding, providing developers with an excellent experience. I think it's well known among application developers that in this use case, using GPUs is indeed very difficult.
To achieve maximum utilization of connected GPUs, whether you buy from Dell or from a hyperscaler, it's really hard to use. But with new software innovations, this will clearly improve. Moreover, the products launched by hyperscalers themselves are really, really great; during the testing phase of computation, you don't need to invest as heavily as you do during training.
Modest
I think this is a very important point regarding GPU utilization. If you consider a training task, you are trying to utilize them at the highest possible percentage over a long period of time. So you are trying to place 500,000, 1 million chips in one place and utilize them at the highest possible rate for up to nine months. The rest is a cluster of 100,000 chips, and if you want to repurpose it for inference, you could say this is not the most efficient build, as inference is peak and bursty, rather than continuous.
So this is what I'm talking about; I just think from first principles, you will rethink how you want to build infrastructure to serve a world that is more focused on inference than training. Jensen has already talked about the wonderfulness of NVIDIA, that you leave this ready-made infrastructure that can then be utilized.
In this world of sunk costs, you might say, of course, if I am forced to build a supercluster with a million chips to train a $50 billion model, then after I finish, I can certainly treat this asset as sweat equity. But from first principles, clearly, you would never build a 2.5-gigawatt cluster of 350,000 chips to meet the kind of demand that Chetan is talking about.
So what does it mean for optical networks if you ultimately have more edge computing with low latency and high efficiency? What does it mean for the power grid? What does it mean for the ability to meet on-site power demands versus getting power from local utilities? I think these are the types of questions I am very interested in reading about.
But so far, much of the analysis is still focused on what happens when we ignite Three Mile Island, as the new patterns are indeed still premature
There Are Still Areas for Innovation in the Semiconductor Field
Patrick
Do you think that despite the significant innovations in the semiconductor field, we still need and will see these innovations? Whether in networking, optics, or the chips themselves, different types of chips.
Modest
I think this will further accelerate the process because it's hard to envision a world that adopts large-scale green energy during training. In my view, for centuries, there have been gold rushes and land grabs, where everyone is just focused on the immediate. But in the tech field, as some stability forms, we will enter an optimization phase. In terms of reasoning, you have already experienced such a period.
What Chetan refers to is that people have had time to optimize the underlying algorithms in computers, and the reasoning error rate has dropped by 99%. This is similar to the situation of internet transmission at the end of the internet bubble, when people said, no, you can never stream movies online. Do you know how much that would cost? And the transmission costs have consistently dropped by 25% every year for 20 years.
The actual profit pool of the business has remained unchanged over 20 years. So I think we have experienced this crazy surge in demand, and I feel that if we can stabilize a bit and let everyone catch their breath, there will be two people in a garage optimizing everything that can be optimized. In the long run, that's the charm of technology; it's deflationary because it's an optimization problem, but when you're frantically capturing the market, you don't have time to optimize. I mentioned this to you last time.
The data center industry is neutral in terms of power. The overall demand for power in the data center business has not grown in five years. This is because it was at a fully mature stage of cloud data center construction. I don't know when you will reach that stage.
What I mean is, we know that those who have at least three or four years until 2026 or 2027 will be fully committed to construction.
At what point will everyone have time to take a deep breath and say, okay, now let's figure out how to operate these more efficiently? That's the essence of things. It's the same in computing. I just think we haven't reached the level where technicians can apply their optimizations. They have been implementing.
Chetan
I will provide you with a few data points from my side. My partner Eric is a board member of an excellent semiconductor company, Cerebras, which recently announced that Cerebras' Llama 3.1 can generate over 40.5 trillion inference results per second, generating over 900 tokens per second, which is a significant order of magnitude increase. I think, for example, this is 70 to 75 times faster than GPU inference speed. So, as we enter the world of reasoning, semiconductor layers, networking layers, etc., startups have a lot of opportunities to truly differentiate themselves.
The second thing I want to mention is that I recently spoke with the Chief Information Officer of a large financial services institution, who said that over the past two years, they have pre-purchased a lot of graphics processing units (GPUs) because they believe there will be many AI applications Workload, who knows if they themselves need to undergo some training. So these systems are now being installed in their data centers and are already online. In this world, you don't need to create models yourself. Even if you do, it's just fine-tuning open-source models. It's not that complicated.
So his point is, you see, if you have AI applications running locally, it's basically free. I have all this capacity, and I'm not using it for anything. Inference is lightweight, so right now I have unlimited capacity to run AI applications locally, and this doesn't increase my marginal costs at all because all this stuff is up and running, and I'm not using it for anything, so I'm ready to buy.
So, not only are all these application things you talk about extremely exciting because they can unleash ROI and all related things, but once you can run any of them on our devices, it will greatly reduce our costs.
So, when you have something like this, it's a win-win-win situation everywhere. That's the current state of affairs. Now, how long will this overcapacity last? Application developers are known for fully utilizing all capacity and pushing limits, and suddenly, what was once excess capacity will eventually turn into insufficient capacity because suddenly we built extensively and then decided to stream video on top of it.
Of course, AI applications will become increasingly complex and consume all this capacity. But from an investment perspective, this is a more predictable and rational world, rather than an infinite expansion in the case of pre-training.
Modest
One thing I'm curious about and want to focus on is: it's important to remember that the report doesn't say the models aren't improving—rather, it says the models aren't improving relative to expectations or the computational load applied to them. So I think we do need to be cautious in concluding that the labs won't continue to work on cracking the problems.
Regarding pre-training, I think the issue is, first, what should we be looking for? But secondly, if they continue to push in that direction, do we believe, and this has been a question I've been pondering, that if scaling laws hold in pre-training, are people willing to spend $100 billion?
And I know everyone says if you're fighting for the ultimate prize, you'll definitely do that, but there have been enough voices of doubt raised about whether pure brute-force pre-training is the way to the ultimate unlock. Or is it now some combination of pre-training, post-training, and computational testing? In this case, again, I think the situation in the world is just much more reasonable from a mathematical perspective. And I've seen a lot of comments where people claim that the development of AI has come to an end, etc.
The insight I hope to gain from today is that I think those who really delve into this issue wouldn't say that. People would say AI is advancing at full speed. I think the question is what the axis of progress really is. From my perspective, this statement seems much more reasonable It seems much more reasonable to take this path rather than spending any possible amount in advance to build this hypothetical god. So I think if we ultimately go down this road, it would be a much better outcome.
Patrick
I'm curious about what you think is the most under-discussed aspect throughout the event, if there is one. Is there anything you find yourself thinking about much more than the discussions you hear from friends and colleagues?
Chetan
From the perspective of public investors, just reading sell-side reports, what we see are sell-side reports or analyses regarding what this new testing time calculation paradigm means and how things are changing. So I really look forward to more sell-side analysis on this new paradigm shift. I think there is also very little relevant reporting in the private market.
What people who meet these entrepreneurs learn is how efficient these entrepreneurs' investments are in cutting-edge fields today. And this is a shift that has only recently occurred. You would see people being able to invest less than a million dollars and achieve performance comparable to cutting-edge models in specific use cases, rather than broadly. And this is something we hadn't seen two years ago or even a year ago.
Modest
Pre-training is a significant test of capitalism. If we continue down this path, I think analyzing what will happen from a microeconomic background will be much better because you don't have to consider the intrinsic value of god. I just feel that, in terms of what I expect to read and hear, this is much better. Yes, I do hope to see thoughtful internal analysts really working hard to address...
Right now, I feel there's a bit of a defensive implication. People are defending the fact that scale hasn't expanded but has merely shifted. That's fine. But now we need to look at the second-order effects and third-order effects.
So how does this manifest? I think this is very beneficial for the entire ecosystem and the whole economy. But I think a lot of surplus will shift from places that previously seemed like winners, while a large surplus will emerge from those that seemed like losers.
Patrick
What kind of outcome in the next six months would leave you feeling the most perplexed?
Chetan
Well, on the positive side, there are two compelling examples: if someone comes out with results showing that pre-training is back in vogue and has made significant breakthroughs in synthetic data, suddenly, things would kick off again. Billion-dollar and ten-billion-dollar clusters would return to the negotiating table. You would go back, but suddenly, the paradigm shift would be astonishing. Suddenly, we would be talking about a super cluster worth a hundred billion dollars that is undergoing pre-training.
So clearly, if my expectations come true, that we will achieve general artificial intelligence next year, we will have general artificial intelligence, and we are building a cluster worth a hundred billion dollars because we have made breakthroughs in synthetic data, everything will work, and we can simulate everything I think another situation is that it is now very clear that while we have exhausted the data on text, we are far from exhausting the data on video and audio. And I believe that the capabilities of these models in new forms of patterns are still to be determined. So we just don't know, because the focus hasn't been there before. But now you are starting to see large labs talking more about audio and video.
From the perspective of human interaction, what these models will be able to achieve, I think will be quite astonishing. I think you have already seen how much of a leap there has been in image generation and video generation. And what it will be like a year or two from now could be incredibly hard to believe.
Modest
Yes, I think the difficult part for non-technical experts is that for the past year to year and a half, the question has been what GPT-5 will bring if we follow the scaling laws. No one has been able to articulate it clearly because what we know is that, okay, the training loss will decrease. So you would say, okay, this thing will be more accurate in the next token prediction. But from the perspective of capability, what does that actually mean?
What are the emerging capabilities that we did not realize before the release? So I think unless the labs come out and say that its accuracy is very good and worth continuing along this logarithmic linear normalization trajectory. If someone says that, I think regardless of the overall discussion or what you might believe, you have to say, okay, this situation has come up again. I just think you have to keep a super open mind.
If we had this conversation three months ago, there would have been private discussions, but not public ones. I just think you have to constantly update your prior knowledge. So obviously, as Jason said, I would look for something like that. Personally, I am closely watching Llama.
Obviously, there is a risk at some point that they decide not to remain open source. If I were another participant in the ecosystem, I would do everything I could to ensure that Llama remains open source. And there are certain ways to achieve that.
But I think that is just one aspect because their willingness to invest at the forefront, and the way they provide those models, I think has fundamentally changed the strategic dynamics of the model industry. So that is another aspect I would pay attention to.
Thoughts on Artificial General Intelligence (AGI) and Subsequent Developments
Patrick
As we approach the end of our discussion, I have a philosophical question about Artificial Superintelligence (ASI). So, if Artificial General Intelligence (AGI) already exists or will appear next year, how would you both view it? I think this builds on the previous discussion about what our expectations are for GPT-5, which is stuck at the scaling barrier. What does that mean?
Because at least in simple chat interactions, I can imagine that there are fewer and fewer things it could do much better, and I don't even know what that would look like. And we may still just be in the early stages of application development, fine-tuning, improvements, algorithm updates, and so on So I am very curious, from a philosophical perspective, what do you think the key criteria for evaluation would be for things beyond what we are inherently endowed with, or what they might be, given that existing models are constantly being adjusted, optimized, and improved. What does artificial intelligence really mean?
Does it mean that it solves previously unsolvable mathematical or physical problems, or is it something else?
What does that idea mean to both of you?
Chetan
None of this is my words. I don't remember who originally said it, but humans are really good at changing the standards of expectations. The significance of artificial intelligence in the 1970s is different from its significance in the 1980s, 1990s, early 21st century, and in 2024. So, if a computer can do something, humans will have a nice way to describe it as automation. Anything that computers cannot do now becomes the new standard for artificial intelligence.
So I think these systems are extremely intelligent, excelling in replicating human intelligence, and sometimes even surpassing human intelligence. I believe that if you look at some model developers like DeepMind and several startups in fields like mathematics, physics, and biology, it will be very clear that the applications and outputs of these models will be things that humans previously could not do at all. We have already seen this in areas like protein folding.
Today, we are starting to see some related things, which involve mathematical proofs. I am confident that this will also involve physical proofs. So my optimistic hope for humanity is that I don't know, we might be able to open wormholes or something like that, and we will be able to study general relativity on an unprecedented scale, study black holes, or simulate black holes in ways we could not do before.
At this moment, all of this sounds a bit absurd, but given the way things are progressing and have already developed, we do not know what is possible and what is impossible.
From an investor's perspective, when facing an unknown future, the possibilities depend on your imagination, which is often a good time for early investors because it means that technology has been unlocked. Typically, when technology unlocks in astonishing ways, distribution unlocks as well, and at that point, you can go after those customers that were once very expensive.
So previously, if you wanted to develop a consumer application, you had to consider app store taxes, search ad networks, and all that kind of stuff. And suddenly, this is just something that can be done quickly in terms of unit economics.
Similarly, in the Software as a Service (SaaS) space, the situation is similar in terms of A) productivity, gross margins, and infrastructure costs. You are just trying to do spreadsheet operations, and early investments start to look more like spreadsheet operations rather than true technological innovation. I think when you have such significant breakthroughs, everything will change again. Distribution becomes nearly free.
If you have something unique and it has word-of-mouth and viral factors, then technology spending actually goes back to just investing in your developers, your researchers, and R&D, and the return on investment from R&D will start to become significant As early investors, the most exciting thing is that we do not know what the future holds, so we return to human creativity and people's ability to break through these boundaries.
Modest
This is exciting for early investors, but I think it is frightening for somewhat skeptical public market investors. Prices are based on feelings rather than mathematical calculations. In the spreadsheet about ASI, I think we have discussed this concept before. This is why people spend so much.
Spending a lot of time on this is because it is so profound.
Ultimately, some people hold an almost religious view of what we are building. Whenever this happens, I think the risks become higher. It’s a bit elusive and super complex. So we all enjoy debating this.
But I think there is one thing we have not mentioned here, which is that there is a group of people who are quite eager to believe that recursive self-improvement will emerge at some point. And I think, regardless of what the hypothetical ASI means, this will be an important breakthrough path, that is, when machines are smart enough to self-learn and self-teach.
From a less dramatic perspective, I think about it this way: there is AlphaGo, which made a move that had never been seen before, I think it was the 37th move, and everyone was super confused, but it ultimately won. Another example I like is Noam Brown, because I love poker; he talked about his poker robot, which was betting amounts far exceeding what professionals had ever seen in high-stakes, no-limit games.
He thought the robot made a mistake. Ultimately, it greatly disrupted the stability of professionals. Think about it. A computer disrupted the stability of humans in their methods to the extent that they are now over-betting in competitions to some degree.
So these are two examples. If we think that pre-training is limited by the datasets we provide, and if we do not have the ability to generate synthetic data, here are two examples where algorithms did something beyond the scope of human knowledge. And this has always puzzled me about the idea that large language models themselves can achieve superintelligence, because functionally, they are limited by the amount of data we provide in advance.
So, if you have such examples where algorithms can exceed their initial limitations, that is very interesting. I am not smart enough to know where this will lead us, but I think the next thing to think about is how to break free from predetermined limitations.
Chetan
In my view, what is remarkable is the extent to which this innovation is happening in the United States, and particularly in Silicon Valley. We have gone through tough years since the pandemic, which is truly astonishing. I have an investment friend who is not in Silicon Valley, and he just said, I can’t believe this is happening again in Silicon Valley. It has become such a beacon, with all the laboratories concentrated here.
Many people working on these applications, these infrastructure companies, and so on are here, and even if they are not here, they are somehow connected to this place and often come here to visit. I want to say that the focus on innovation here is indeed outstanding In terms of artificial intelligence, the progress made in the United States, especially in Silicon Valley, is quite significant. I do believe that one aspect investors and entrepreneurs are currently focused on is how fragile this system is, and to what extent we need to protect it and continue investing in it.
Moreover, I think there is a lot of attention now on the fact that innovation is something that needs to be protected. I feel that many people are now putting in a lot of effort to ensure that all these innovations happening in the United States continue to benefit everyone. I think this is a very optimistic and encouraging realization.
Modest
If the report is true, the agglomeration effect is real. The way the paper "Transformer" came about was that someone was roller-skating in the hallway, overheard two people talking about something, walked in, wrote on the whiteboard, and then two more people came over; who knows how much of that was fictional?
But from an economist's perspective, what is fascinating is that these human network effects are real, the COVID-19 pandemic did not destroy them, remote work did not destroy them, and the gathering of people, the fusion of ideas, and the convergence of disciplines to build this world-changing architecture indeed has its tangible aspects.
Patrick
Guys, it's always a pleasure to chat with you two. I'm lucky to have this private conversation. It's also fun to do it in public. Thank you for taking the time.
Modest
Of course.
Chetan
Thank you!