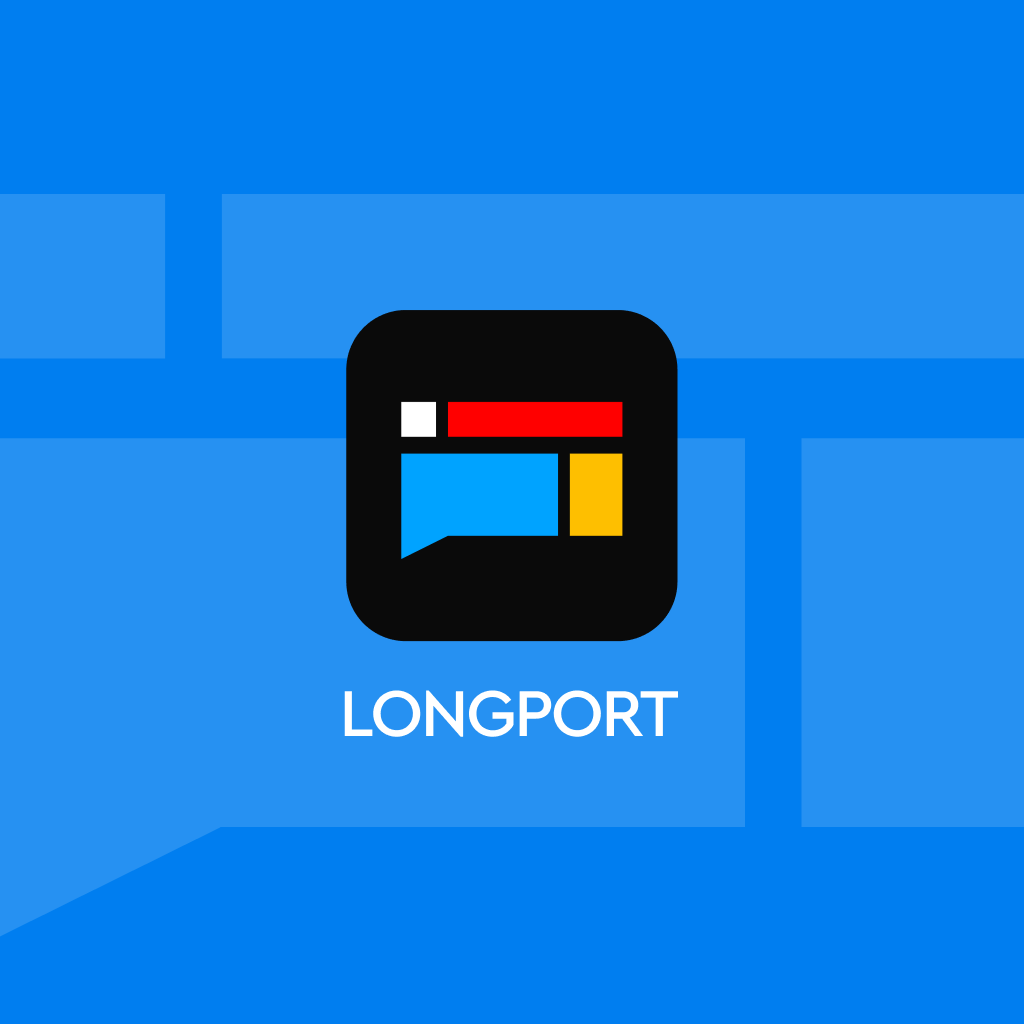
Apple officially announces: Supporting the training of Apple Intelligence models on Google's custom chips
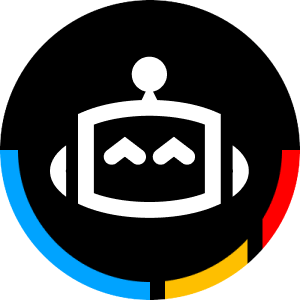
Apple's paper reveals that a large server language model, the Apple Fundamental Model (AFM), was trained on 8192 Google TPUv4 chips, with 63 trillion tokens trained; the edge-side AFM was trained on 2048 TPUv5p chips; AFM and AFM services were trained on the "Cloud TPU cluster"
Author: Li Dan
Source: Hard AI
Public documents show that Apple's development of its own artificial intelligence (AI) system Apple Intelligence relies on the support of Google's custom chips.
On Monday, July 29th, Eastern Time, Apple's official website published a technical paper detailing the development of basic language models to support Apple's personal intelligent system Apple Intelligence. This includes an on-device efficient running model with about 3 billion parameters - the on-device "Apple Basic Model" (AFM), and a large server language model designed for Apple's cloud AI architecture "Private Cloud Compute" - the server AFM.
In the paper, Apple introduces that the on-device AFM and server AFM are members of Apple's generative model family, all of which are used to support users and developers. Apple disclosed in the paper that the training models used Google's fourth-generation AI ASIC chip TPUv4 and the next-generation chip TPUv5. The article states:
"We trained the server AFM from scratch on 8192 TPUv4 chips, using a sequence length of 4096 and a batch size of 4096, for training with 6.3 trillion tokens."
"The on-device AFM was trained on 2048 TPUv5p chips."
In this 47-page paper, Apple did not mention the names of Google or NVIDIA, but stated that its AFM and AFM services were trained on a "cloud TPU cluster". This means that Apple rented servers from cloud service providers to perform computations.
In fact, during this year's Worldwide Developers Conference (WWDC) in June, the media had already discovered from the technical details released by Apple that Google has become another winner for Apple in the field of AI. Apple's engineers used the company's self-developed framework software and various hardware, including the tensor processing unit (TPU) available only on Google Cloud, when building the basic models. However, Apple did not disclose how much it relies on Google's chips and software compared to other AI hardware suppliers like NVIDIA.
Therefore, comments on social media X on Monday pointed out that news about Apple using Google chips had already come out in June, and now we have more details about the training stack.
Some comments mentioned that Apple does not dislike NVIDIA, but TPU is faster. Others mentioned that TPU is faster, so it makes sense for Apple to use it, and it may also be cheaper than NVIDIA's chips.
Some comments stated that Apple does not dislike NVIDIA, but TPU is faster. Others mentioned that TPU is faster, so it makes sense for Apple to use it, and it may also be cheaper than NVIDIA's chips.
This week, a media commentary stated that Google's TPU was originally created for internal workloads and is now being more widely used. Apple's decision to train models with Google chips indicates that in the field of AI training, some tech giants may be seeking and have found alternatives to NVIDIA's AI chips.
Wall Street News previously mentioned that last week, Meta's CEO Zuckerberg and Alphabet's CEO Pichai both hinted in their speeches that their companies and other tech companies may be investing excessively in AI infrastructure, "possibly overinvesting in AI." However, they both acknowledged that the business risks would be too high if they did not do so.
Zuckerberg said:
"The consequence of falling behind is that you will be at a disadvantage in the most important technologies for the next 10 to 15 years."
Pichai said:
AI is costly, but the risk of underinvestment is greater. Google may have overinvested in AI infrastructure, mainly including the purchase of NVIDIA's GPUs. Even if the AI boom slows down, the data centers and computer chips purchased by the company can be used for other purposes. For us, the risk of underinvestment is far greater than the risk of overinvestment