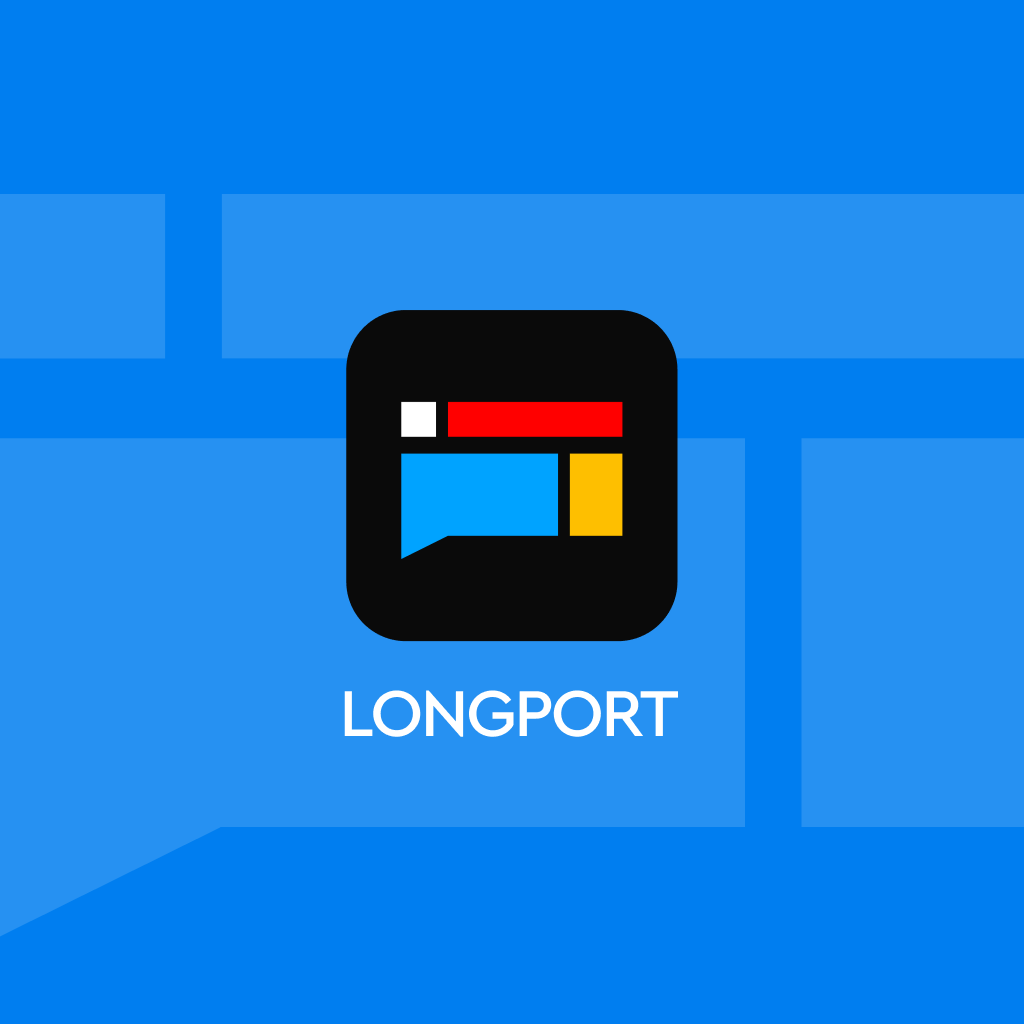
In the era of large models, are small companies sidelined?
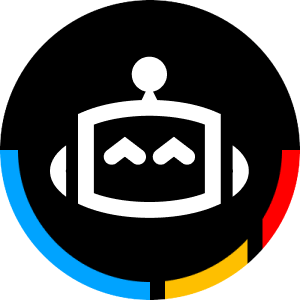
In the era of large models, small companies may be marginalized, but small models will create new opportunities for them. Morgan Stanley believes that NVIDIA is the key to future computing power growth. Google, Meta, Amazon, and Microsoft will be beneficiaries in the development of large models. Morgan Stanley has given these large tech companies an overweight rating. The development of large models will require unprecedented computing power, posing a huge challenge for small companies. Morgan Stanley's report points out that the cost of developing large models is high, and barriers such as chip technology and artificial intelligence technology have increased, posing significant obstacles to entering the field of large models. Future computing power will experience exponential growth, and NVIDIA's chip technology is one of the key drivers of computing power growth
Author: Bu Shuqing
Source: Hard AI
Meta's third-generation large model Llama 3 finally officially debuted this week: with a maximum parameter scale exceeding 400 billion, training tokens exceeding 15 trillion, and a human evaluation win rate of over 60% compared to GPT-3.5. The official claim is "the strongest open-source model on the planet."
In the "internal competition" of major technology giants, large models have finally reached a crucial turning point. Morgan Stanley pointed out that the world is entering a new era of rapid growth in large model capabilities driven by hardware and software together, significantly enhancing the ability of large models in creativity, strategic thinking, and handling complex multidimensional tasks.
The report emphasizes that the training of future large models will require unprecedented computing power, leading to a significant increase in development costs. Analysts from Morgan Stanley led by Stephen C Byrd, in a report released this week, estimate that the soaring cost of supercomputers needed to train the next generation of large models poses a huge challenge even for tech giants, let alone small companies.
The report further points out that in addition to high capital expenditures, barriers such as chip power supply and artificial intelligence technology are increasing. These factors together constitute significant obstacles to entering the field of large models, which may make it difficult for small companies to compete with powerful giant enterprises.
Therefore, Morgan Stanley has given overweight ratings to large tech companies such as Google, Meta, Amazon, and Microsoft, which, with their advantages in technology, capital, and market, are expected to take a leading position in the development of large models. At the same time, although small companies may be marginalized in the world of large models, smaller models with lower costs will create new opportunities for them.
Future Computing Power Exponential Growth, Is NVIDIA Key?
Morgan Stanley points out that in the near future, the computing power required to develop large models will experience exponential growth, closely related to advancements in chip technology, with NVIDIA's "most powerful chip in history" Blackwel being one of the key technologies driving this computational growth.
Taking the training of the GPT model by OpenAI as an example.
Morgan Stanley states that currently, training GPT-4 requires about 100 days, using 25,000 NVIDIA A100 GPUs, processing 13 trillion tokens, and involving approximately 1.76 trillion parameters.
The total computational power of these A100 GPUs (measured in FP8 teraFLOPs) is approximately 16 million. TeraFLOPs is a unit of measuring floating-point operation performance, indicating how many trillion floating-point operations can be executed per second. The total number of floating-point operations required for GPT-4 training is about 1.37 trillion.
For the upcoming GPT-5, Morgan Stanley predicts that the training of this model will require deploying 200,000-300,000 H100 GPUs and take 130-200 days A supercomputer will make it easier to achieve exponential growth expectations. According to Morgan Stanley's model, the computing power provided by supercomputers for developing large models later this decade will be more than 1000 times higher than current levels.
Using Blackwell's supercomputer, it only takes 150-200 days of training to develop a brand new large model. Compared to current large models like GPT-4, the computing power it provides is 1400-1900 times higher than what current models require.
The report also mentions that the annual computing power required for GPT-6 in the future will account for a significant percentage of NVIDIA's chip annual sales. The cost of a 100-megawatt data center using B100 or H100 GPUs is estimated to be around $1.5 billion.
Morgan Stanley sees NVIDIA as a key driver of computing power growth.
According to forecasts, NVIDIA's computing power is expected to grow at a compound annual growth rate of 70% from 2024 to 2026. This growth rate is calculated based on SXM (possibly a codename for a NVIDIA product or service) and FP8 Tensor Core (a performance metric).
In the era of large models, are tech giants the biggest beneficiaries?
However, developing super powerful models and the supercomputers needed for their training involve a series of complex challenges, including capital investment, chip supply, power demand, and software development capabilities. These factors constitute the main barriers to entry into this field, giving well-capitalized, technologically advanced tech giants more opportunities.
In terms of capital investment, Morgan Stanley compared the data center capital expenditures of Google, Meta, Amazon, and Microsoft in 2024 for a range of different-sized supercomputers. The estimated cost of a 1-gigawatt supercomputer facility is around $30 billion, while the cost of larger-scale supercomputers could be as high as $100 billion.
Morgan Stanley expects that the data center capital expenditures of these four U.S. mega-scale computing companies in 2024 and 2025 will reach approximately $155 billion and over $175 billion, respectively. These huge numbers will deter small businesses.
The institution also believes that Google, Meta, Amazon, and Microsoft will be direct beneficiaries of computing power growth and has given these four companies a buy rating
Where are the opportunities for small companies?
Although small companies may be marginalized in the development of more complex large models, the development of small models will create new opportunities for them.
Morgan Stanley stated that the development cost of small models is lower, and in the future, they may realize significant benefits in specific industry sectors, driving the rapid popularization of general artificial intelligence technology.
Our latest general artificial intelligence model includes a tool that can calculate the training cost of small model-related data centers. We believe this is a useful starting point for evaluating the return on investment capital (ROIC) of small models in specific fields.
We believe that the decrease in the cost and the improvement in the capabilities of small models strengthen our evaluation of the adoption of general artificial intelligence technology in many fields.
With software support, what can future large models do?
It is worth noting that in addition to hardware advancements such as chips, innovations in software architecture will also play a key role in enhancing the capabilities of large models in the future, especially the Tree of Thoughts architecture.
This architecture was proposed by researchers from Google DeepMind and Princeton University in December 2023, drawing inspiration from the way human consciousness works, especially the so-called "System 2" thinking. "System 2" is a long-term, highly thoughtful cognitive process, as opposed to the fast, unconscious "System 1" thinking, which is more similar to the current operation mode of large models.
This shift will enable large models to work in a way more similar to the human thinking process, highlighting AI's stronger creativity, strategic thinking, and ability to handle complex, multidimensional tasks.
Significant decrease in computing costs
Morgan Stanley's proprietary data center model predicts that with the rapid increase in computing power of large models, computing costs will decrease rapidly. Looking at the evolution from a single chip generation (from NVIDIA Hopper to Blackwell), computing costs have decreased by approximately 50%.
OpenAI CEO Sam Altman has previously emphasized the importance of the significant decrease in computing costs, considering it a key resource for the future. He believes that computing power may become one of the most valuable commodities in the world, with importance comparable to currency.
Furthermore, the report predicts that a few very large supercomputers are likely to be built, most likely near existing nuclear power plants.
In the United States, Morgan Stanley expects Pennsylvania and Illinois to be the best locations for developing supercomputers, as these regions have multiple nuclear power plants that can support the energy needs of supercomputers with multiple gigawatts