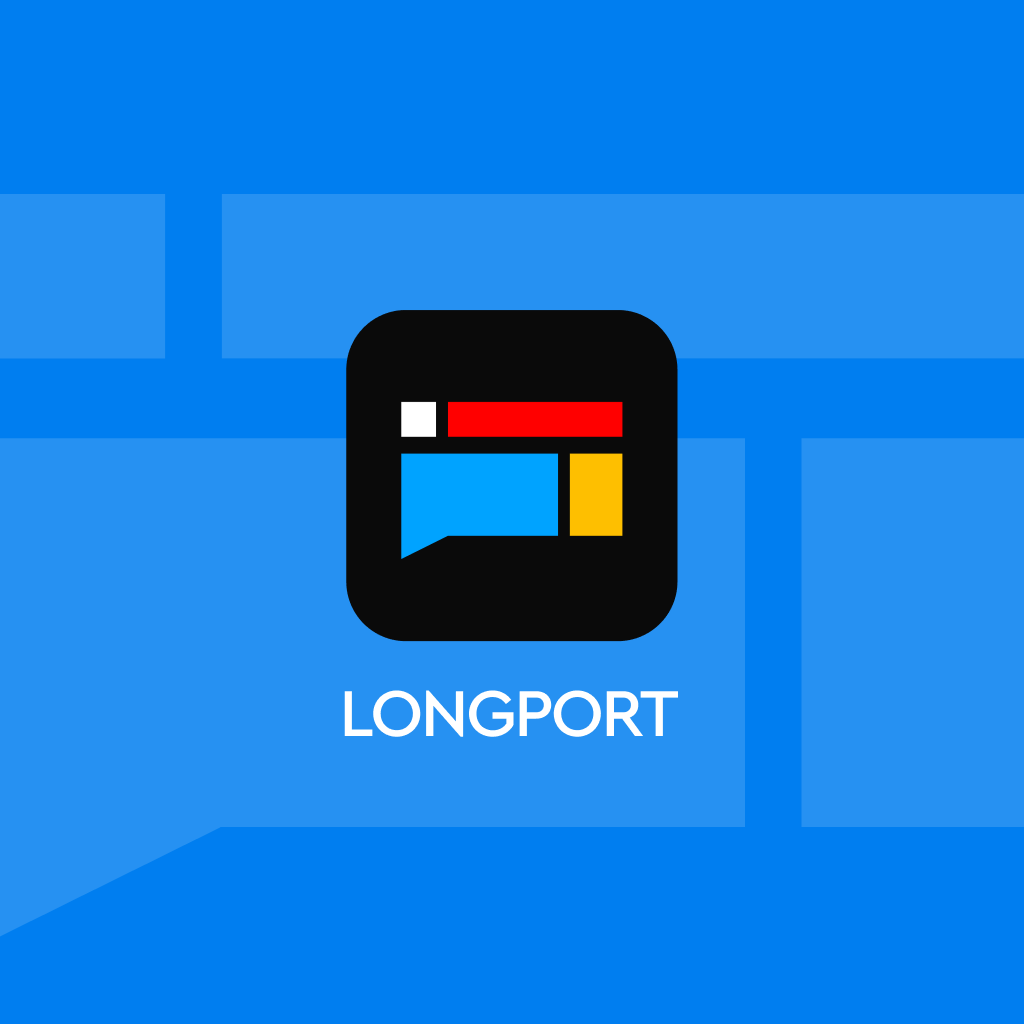
Mark Zuckerberg's latest 20,000-word interview: The "most powerful open-source large model" Llama3 worth billions of dollars and everything behind it
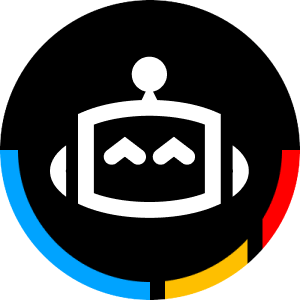
In a recent 20,000-word interview, Mark Zuckerberg discussed Meta's launch of the Llama 3 models and the potential challenges in AI development, such as GPU supply, funding, and energy. He emphasized that the purpose of AI is to empower people with more powerful tools rather than replace humans. Furthermore, he mentioned that AI is transitioning from question-answering tools to reasoning systems, requiring an understanding of context, knowledge integration, and logical reasoning. For Meta, emotional understanding is an important area, and a breakthrough in this area could make interactions between humans and machines more natural and profound. The emergence of AI is expected to significantly improve the efficiency of programmers
On April 18th, Meta launched Llama 3, calling it "the most powerful open-source large model to date", once again impacting the competitive landscape of AI large models and igniting the AI circle.
On the same day, Meta CEO Mark Zuckerberg's interview with well-known tech podcast host Dwarkesh Patel was also released simultaneously. In this 80-minute interview, they mainly discussed Llama 3, Artificial General Intelligence (AGI), energy issues, AI security issues, risks and significance of open source.
Zuckerberg stated that AI has become the core of Meta, and Meta AI is currently the most intelligent AI assistant available for free use. The upcoming large-scale version of Llama 3 will have over 400 billion parameters.
Regarding the training and development of AI models, Zuckerberg mentioned that the emergence of Llama 3 confirms the importance of large-scale data and computing resources for AI models. In the future, training large AI models may face challenges such as capital and energy limitations. He emphasized that the emergence of AI is not an attempt to replace humans but to empower people with more powerful tools to accomplish more challenging tasks. The key points of the interview are as follows:
- The smallest parameter Llama 3 with 80 billion and the largest parameter previous generation Llama 2 with 700 billion model performance are of the same order of magnitude, while the most powerful 405 billion parameter version is still in the works.
- The emergence of Llama 3 confirms the importance of large-scale data and computing resources for AI models. AI is transitioning from a "question-answering" tool to a more general "reasoning" system, which requires understanding the context of the problem, integrating knowledge from various aspects, and using logical reasoning to draw conclusions.
- Multimodality is a key focus area for Meta, with a special focus on emotional understanding. If breakthroughs can be made in this area, allowing artificial intelligence to truly understand and express emotions, the interaction between humans and machines will become unprecedentedly natural and profound.
- AI will indeed change the way humans work, significantly improving the efficiency of programmers. However, the emergence of AI is not an attempt to replace humans but to empower people with these tools to have more capabilities, enabling them to accomplish tasks that were previously unimaginable.
- AI will fundamentally change human life, bringing many new applications that were previously impossible, and reasoning will profoundly change almost all product forms.
- When AI development encounters GPU bottlenecks or insufficient funding issues, it will first encounter energy problems. If humans can solve the energy problem, it is entirely possible to build larger-scale computing clusters than now.
- I believe that in the future, there will be META AI general assistant products, and every company hopes to have an AI that represents their interests. AI will drive progress in science, healthcare, and various fields, ultimately impacting all aspects of products and the economy.
- I believe that in the future, if artificial intelligence becomes overly centralized, its potential risks may be no less than its widespread dissemination. If an institution has more powerful artificial intelligence than everyone else, is this also a bad thing?
- I believe there are multiple possibilities for the development of training, and commercialization is indeed one of them. Commercialization means that as the choices in the market increase, the cost of training will be greatly reduced, making it more affordable.
- The issue of existential risk is indeed worth our attention. Currently, we are more concerned about content risk, that is, the model may be used to create violence, fraud, or other harmful behaviors.
- Open source is becoming a new and powerful way to build large models. Although specific products will continue to evolve, appear, and disappear over time, their contributions to human society are enduring.
- Meta may soon train large models on self-developed chips, but Llama-4 may not be able to do so yet.
Here is the full interview:
Llama 3 Ultimate Edition is still in training
Dwarkesh Patel: Mark, welcome to this podcast.
Mark Zuckerberg: Thank you for inviting me. I am a loyal fan of your podcast.
Dwarkesh Patel: Thank you very much for your praise. Let's first talk about the products that will be released simultaneously with this interview. Can you tell me about the latest developments in Meta AI and related models? What are the exciting aspects?
Mark Zuckerberg: I think most people will notice the new version of Meta AI. The most important thing we are doing is upgrading the models. We have released Llama-3. We provide it to the developer community in an open-source manner, and it will also support Meta AI. There are many aspects of Llama-3 worth discussing, but I think the most important point is that we now consider Meta AI to be the smartest AI assistant that people can get for free, and we have integrated Google and Bing to access real-time knowledge.
We will make it more prominent in our apps, at the top of Facebook and Messenger, where you can directly use the search box to ask questions. We have also added some cool creative features that I think people will love. I think animation is a great example, where you can basically make any image come to life.
One amazing thing people will find is that it can now generate high-quality images so quickly, actually generating and updating in real-time as you type. You input your query, and it will adapt, like "show me a picture of a cow standing in a field with mountains in the background, eating a Hawaiian fruit, and drinking beer," and it will update the image in real-time, which is very cool and I think people will love it. I think this will be something most people can experience in the real world. We are rolling it out, starting from a few countries, and will expand in the coming weeks and months. I think this will be a great thing, and I'm really excited to put it in people's hands. This is a big step for Meta AI But if you want to delve deeper, Llama-3 is obviously the most interesting technically. We are training three versions: We actually trained three versions, with dense models of 8 billion, 70 billion, and 405 billion parameters, with the 405 billion model still in training, so we did not release it today. But I am very excited about the performance of the 8 billion and 70 billion versions, which are leading in terms of their scale. We will release a blog post with all the benchmark results attached, so people can take a look for themselves. It is obviously open source, so everyone has the opportunity to try it out.
We have a new roadmap for the upcoming version, which will bring multimodality, more multilingual capabilities, and a larger context window. We hope to launch the 405 billion parameter version later this year. Based on the current training progress, it has already reached around 85 on MMLU, and we expect it to perform well in many benchmark tests. I am very excited about all of this. The 70 billion version is also great. We are releasing it today. It scores around 82 on MMLU, with leading performance in mathematics and reasoning. I think it will be very cool to put it in people's hands.
Dwarkesh Patel: Interesting, this is the first time I've heard of MMLU as a benchmark. This is very impressive.
Mark Zuckerberg: The 8 billion parameter version is almost as powerful as our largest Llama-2 version we released. So the smallest Llama-3 is basically as powerful as the largest Llama-2.
Dwarkesh Patel: Before we delve into discussing these models, I want to go back in time. I guess you started acquiring these H100s in 2022, or you can tell me specifically when. The stock price was hit hard at that time. People were questioning these capital expenditures. People didn't buy into the metaverse. I think you spent capital to buy these H100s. How did you know to buy H100 at that time? How did you know you needed GPUs?
Mark Zuckerberg: I think it was because we were developing Reels at that time. We always wanted enough computing power to build things for the future that we couldn't see yet. We encountered this situation when developing Reels, where we needed more GPUs to train models. This was a significant evolution for our service. We were not just sorting content from people or pages you follow; we started heavily recommending what we call non-associated content, content from people or pages you don't follow.
The content candidate pool we might show you expanded from the order of thousands to the order of millions. It required a completely different infrastructure. We started working on this, but we were limited in infrastructure and couldn't keep up with the pace of TikTok as we wanted. I basically thought, "Hey, we have to make sure we don't get into this situation again. So let's order enough GPUs to do what needs to be done for Reels, content ranking, and the information feed. But let's double it." Again, our general principle is that there will always be things in the future that we can't see yet
The Road to AGI
Dwarkesh Patel: Do you know what will be AI?
Mark Zuckerberg: We think it will be something related to training large models. At that time, I thought it might be related to content. It's just a pattern matching of running a company, there will always be another direction to deal with. At that time, I was deeply involved in trying to make the Reels and other content recommendation systems work well. This was a huge breakthrough for Instagram and Facebook, being able to show people interesting content from others they didn't even follow.
But in hindsight, this decision was very correct, it came from our setbacks. It's not because "oh, I thought too much". In fact, most of the time, the reason we make some decisions that later seem good is because we messed up something before and just don't want to repeat the mistake.
Dwarkesh Patel: This is completely off-topic, but I want to ask now. We'll get back to the topic of AI later. In 2006, you didn't sell for $1 billion, but I'm sure you had a price in mind that you would sell for, right? Have you ever thought, "I think the actual valuation of Facebook at the time was this much, and the price they offered was not reasonable"? If they offered $5 trillion, you would of course sell. So how did you weigh this choice at the time?
Mark Zuckerberg: I think some things are just personal. I don't know if I had enough savvy at the time to do that kind of analysis. People around me were looking for all kinds of arguments for $1 billion, like "we need to create this much revenue, we need to reach this size. This was obviously many years later." It was far beyond our scale at the time. I didn't really have the financial expertise needed to engage in that kind of debate at the time.
Deep down, I believed in what we were doing. I did some analysis, "If I don't do this, what would I do? Well, I really like creating things, I like helping people communicate. I like understanding what's happening and the interactions between people. So I thought, if I sold this company, I might start another similar company, and I quite like the one I have now. So why bother?" I think many of the biggest bets people make are often based on beliefs and values. In fact, doing forward-looking analysis is often very difficult.
Mark Zuckerberg: I don't know the specifics of the timeline. I think these things will progress gradually over time.
Dwarkesh Patel: But in the end, Llama-10.
Mark Zuckerberg: I think this question contains a lot of content. I'm not sure if we are replacing people, or more so providing tools for people to do more things.
Dwarkesh Patel: With Llama-10, will the programmers in this building become 10 times more productive? Mark Zuckerberg: I hope for more than just a 10x improvement. I don't think there's a single threshold of intelligence for humans because people have different skills. I think at some point, AI may surpass humans in most things, depending on the strength of the models.
But I think it's incremental, I don't think AGI is just one thing. You're basically adding different capabilities. Multimodal is a key point we're focusing on now, initially it was photos, images, and text, but eventually it will extend to videos. Because we're very focused on the metaverse, so 3D types of things are also important. One modality that I'm very focused on, that I haven't seen many others in the industry focus on, is emotional understanding. The human brain has so many parts that are just specialized for understanding people, understanding expressions, and emotions. I think that in itself is a complete modality, allowing artificial intelligence to truly understand and express emotions, making interactions between humans and machines unprecedentedly natural and profound.
So besides significant improvements in reasoning and memory, there are many different capabilities you want to train models to focus on, and memory itself is a complete thing. I don't think in the future we'll mainly stuff things into a query context window to ask more complex questions. There will be different memories or different customized models, they will be more personalized. These are just different capabilities. Obviously, there's also making them bigger or smaller. We focus on both. If you're running something like Meta AI, it's very server-based. We also want it to run on smart glasses, and there's not much space in smart glasses. So you want something very efficient to achieve this.
Dwarkesh Patel: If you use intelligence at an industrial scale for reasoning worth hundreds of billions of dollars, or even eventually worth trillions of dollars, what are the use cases? Is it simulation? Is it artificial intelligence in the metaverse? What are we using the data centers for?
Mark Zuckerberg: Our bet is that it will basically change all products. I think there will be a kind of Meta AI general assistant product. I think it will transition from something more like a chatbot where you ask a question, it formulates an answer, to you giving it more complex tasks, and then it goes off and completes those tasks. So, this requires a lot of reasoning, as well as a lot of computation and other ways.
And then I think interacting with other people's other intelligences will be a big part of what we do, whether it's for businesses or creators. An important theory I have about this is that there won't be just one AI you interact with, every business will want an AI that represents their interests. They won't want to primarily interact with an AI that sells competitors' products.
I think creators will be a large group. We have about 200 million creators on our platform. They basically all have this pattern where they want to engage their community, but they're limited by time. Their community usually wants to engage with them, but they don't know they're limited by daytime. If you can create something where creators can basically have an AI, train it the way they want, and get their community involved, I think this will also be very powerful, and all these things will have a lot of involvement.
These are just consumer use cases. My wife and I run our foundation, the Chan Zuckerberg Initiative. We have done a lot of work in science, and obviously a lot of AI work will advance science, healthcare, and all these things. So, it will ultimately impact virtually every field of products and the economy.
Dwarkesh Patel: You mentioned that AI can do some multi-step things for you. Is this a larger model? For example, for Llama-4, will there still be a version with 700 billion parameters, but you just need to train it on the right data and it will be very powerful? What is the progress like? Is it vertical scaling? Or is it like you said, the same size but with different databases?
Mark Zuckerberg: I don't know if we know the answer to that question. I think something that seems like a pattern is that you have the Llama model, and then you build some kind of other application-specific code around it. Some of it is fine-tuning for use cases, but some of it is, for example, how should Meta AI use tools like Google or Bing to introduce real-time knowledge logic. This is not part of the basic Llama model. For Llama-2, we have some of that, it's more hand-designed. Part of the goal for Llama-3 is to incorporate more of these things into the model itself. For Llama-3, as we start to get into more of these agent-like behaviors, I think some of that will be more hand-designed. The goal for Llama-4 will be to incorporate more of these things into the model.
At each step, you feel what is possible on the horizon. You start tinkering with it, doing some hacks around it. I think that helps you hone your intuition, knowing what you want to try to train in the next version of the model. It makes it more general because obviously for anything you manually code, you can unlock some use cases, but it's inherently fragile and non-general.
Dwarkesh Patel: When you say "incorporate into the model itself," do you mean training it on what the model itself wants? What do you mean by "incorporate into the model itself"?
Mark Zuckerberg: For Llama-2, the use of tools is very specific, while Llama-3 is much better at using tools. We don't have to manually write everything to make it use Google and search. It can do that directly. Similarly, for coding and running code, and many similar things. Once you have this ability, you can glimpse what we can start doing next. We don't necessarily have to wait for Llama-4 to start building these features, so we can start doing some hacks around it. You do a lot of manual coding, at least in the transitional period, which makes the product better. Then this helps point the direction for what we want to build in the next version of the model Dwarkesh Patel: Which community fine-tuning of Llama-3 are you most looking forward to? Perhaps not the most useful one for you, but the one you enjoy playing the most. They made adjustments to it in ancient times, and you will be able to converse with Virgil and so on. What are you interested in?
Mark Zuckerberg: I think the essence of these things is that you will be surprised. Anything specific that I find valuable, we may be building. I think you will get a distilled version. I think you will get a smaller version. One thing is, I think 8 billion is still not small enough to meet a large number of use cases. Over time, I am happy to get a model with 10-20 billion parameters, or even a model with 5 billion parameters, to see what you can do with it.
If there are 8 billion parameters, we are almost as powerful as the largest Llama-2 model, so with 1 billion parameters, you should be able to do some interesting things, and faster. Before providing it to the most powerful model to refine what the prompts should be, it is very suitable for classification, or many basic things people do in understanding user query intent. I think this may be a gap that the community can help fill. We are also considering starting to distill some of these things ourselves, but right now GPUs are all being used to train 40.5 billion models.
Dwarkesh Patel: You have all these GPUs, I think you said you would have 350,000 by the end of this year.
Mark Zuckerberg: That's for the whole series. We built two, I think it's a cluster of 22,000 or 24,000, which is the single cluster we use to train large models, obviously in a lot of what we do. A lot of our stuff is used for training Reels models, Facebook News Feed, and Instagram feed. Inference is a big deal for us because we serve a large number of people. Given the massive scale of the communities we serve, the ratio of inference computation to training may be much higher for us than for most other companies doing this work.
Dwarkesh Patel: In the materials they shared with me in advance, there was an interesting point that the data you use during training is more than just the optimal data used for training. Inference is a big issue for you and for the community, and it makes sense to put tens of trillions of tokens in it.
Mark Zuckerberg: Despite having a model with 700 billion parameters, one interesting thing is that we think it will become more saturated. We trained it with about 1.5 trillion tokens. I think our initial prediction was that it would asymptote more, but even in the end, it is still learning. We could probably give it more tokens, and it would get a little better.
To some extent, you are running a company, and you need to deal with these meta-inference issues. Do we want to spend our GPUs on further training the 700 billion model? Or do we want to continue so we can start testing assumptions for Llama-4? We need to make this decision, and I think we struck a reasonable balance in this version of 700 billion Future will have another 70 billion, the multimodal one, will be launched in the next period. But what is fascinating is that at this point, the architecture can accept so much data.
Energy Bottleneck Restricts Development
Dwarkesh Patel: This is really interesting. What does this mean for the future models? You mentioned that the 8 billion of Llama-3 is better than the 70 billion of Llama-2.
Mark Zuckerberg: No, no, it's almost as good. I don't want to exaggerate. It's on the same order of magnitude.
Dwarkesh Patel: Does this mean that the 70 billion of Llama-4 will be as good as the 405 billion of Llama-3? What does the future look like?
Mark Zuckerberg: That's a great question, right? I think no one knows. In this world, the planning index curve is one of the trickiest things. How long will it continue? I think we are likely to continue. I think it's worth investing hundreds of billions or over 100 billion dollars to build infrastructure and assuming that if it continues to develop, you will get some really amazing things, which will create amazing products. I don't think anyone in the industry can really tell you for sure that it will continue to expand at that rate. Generally, in history, you will encounter bottlenecks at some point. With so much energy being put into this field now, maybe those bottlenecks will be broken soon. I think it's an interesting question.
Dwarkesh Patel: What would the world look like without these bottlenecks? Assuming progress just continues at this pace, it seems possible. From a broader perspective, forget about Llamas...
Mark Zuckerberg: Well, there will be different bottlenecks. In the past few years, I think there was this issue with GPU production. Even companies with money to buy GPUs may not necessarily get as many as they want because of all these supply constraints. Now I think this situation is decreasing. So you see a bunch of companies now considering investing a lot of money to build these things. I think this will continue for a while. There is a capital issue. When is it not worth investing capital anymore?
I actually think before we hit that problem, you will hit an energy constraint. I don't think anyone has built a gigawatt-scale single training cluster. The things you run into will eventually slow down in the world. Getting energy permits is a strictly regulated government function. You start with software, software is regulated to some extent, I think it's more regulated than many tech people think. Obviously, if you are starting a small company, maybe you feel this. We interact with different governments and regulatory agencies around the world, we have a lot of rules to follow and make sure we do well. Undoubtedly, energy is strictly regulated.
If you are talking about building a large new power plant or a large expansion, and then building transmission lines that cross other private or public lands, that is just a strictly regulated thing You mentioned years of preparation time. If we want to build some large facilities, supplying power to them is a very long-term project. I think people will do this, but I don't think it's something that can be achieved by reaching a certain level of artificial intelligence, raising a large amount of funds and investing in it, and then the model will... You will indeed encounter different bottlenecks in the process.
Dwarkesh Patel: Are you saying that even if Meta's R&D budget or capital expenditure budget is now 10 times larger, there are things that cannot be afforded? Is there such a thing, perhaps related to artificial intelligence projects, perhaps not, even companies like Meta don't have the resources? Is there something that has crossed your mind, but with the current Meta, you can't even issue stocks or bonds for it? Is it 10 times larger than your budget?
Mark Zuckerberg: I think energy is one aspect. I think if we can get energy, we might build clusters larger than what we have now.
Dwarkesh Patel: Is this fundamentally limited by funding in extreme cases? If you have $1 trillion...
Mark Zuckerberg: I think it's a matter of time. It depends on how far the exponential curve goes. Many data centers now are around 50 megawatts or 100 megawatts, or a large data center might be 150 megawatts. Take an entire data center, fill it with everything you need to train, and build the largest cluster you can build. I think there are companies doing this.
But when you start building a 300 megawatt, 500 megawatt, or 1 gigawatt data center, no one has built a 1 gigawatt data center yet. I think it will happen. It's just a matter of time, but it won't be next year. Some of these things take years to build. Just to illustrate this point, I think a gigawatt data center is equivalent to a meaningful nuclear power plant, used solely for training a model.
Dwarkesh Patel: Hasn't Amazon done this? They have 950 megawatts.
Mark Zuckerberg: I'm not exactly sure what they have done. You have to ask them.
Dwarkesh Patel: But it doesn't necessarily have to be in the same place, right? If distributed training is effective, it can be distributed.
Mark Zuckerberg: Well, I think that's a big question, how it will work. It seems likely in the future that the training of these large models we are talking about will actually be closer to generating synthetic data for inference, and then feeding it into the model. I don't know what the ratio will be, but I think generating synthetic data is more like inference than training today. Obviously, if you do this to train a model, it is part of a broader training process. So this is an unresolved issue, this balance and how it will develop Dwarkesh Patel: Could this also apply to Llama-3, maybe starting from Llama-4? Like you release it, and if someone has a lot of computing power, then they can use the model you released to make these things arbitrarily intelligent. Suppose there are some random countries, like Kuwait or the UAE, with a lot of computing power, they can actually just use Llama-4 to create smarter things.
Mark Zuckerberg: I do think there will be such dynamics, but I also think the model architecture has a fundamental limitation. I think a 700 billion model trained with the Llama-3 architecture like we did can get better, it can continue to evolve. As I said, we feel that if we continue to give it more data or rotate high-value tokens again, it will continue to get better. We have seen a group of different companies around the world basically adopting the Llama-2 700 billion model architecture and then building a new model. But when you make intergenerational improvements to the Llama-3 700 billion or Llama-3 4050 billion, there are no similar open-source models available today. I think this is a huge leap. What people can build on top of it, I think it can't develop infinitely from there. You can do some optimizations before you reach the next leap.
How far will AI develop in the future?
Dwarkesh Patel: Let's zoom out a bit from specific models or the years of preparation you need to get energy approvals. Looking at the big picture, what will happen to artificial intelligence in the next few decades? Does it feel like another technology, like the metaverse or social, or does it feel like something fundamentally different in the course of human history?
Mark Zuckerberg: I think it will be very fundamental. I think it will be more like the creation of computers themselves. You will get all these new applications, just like when you got the internet or mobile phones. People are basically rethinking all these experiences because many things that were previously impossible have become possible. So I think this will happen, but I think it's at a much lower level of innovation. My feeling is that it will be more like people going from not having computers to having computers.
On a cosmic scale, this will obviously happen rapidly over the next few decades. Some people are worried that it will really get out of control and go from a bit intelligent to extremely intelligent overnight. I just think there are all these physical limitations that make it unlikely to happen. I just don't think it will happen. I think we will have time to adapt a bit. But it will indeed change the way we work and provide people with all these creative tools to do different things. I think it will truly enable people to do more of what they want to do.
Dwarkesh Patel: So maybe not overnight, but can we think about these milestones in this way on a cosmic scale? Humans evolved, then artificial intelligence appeared, and then they went to the galaxy. Maybe it will take decades, maybe a century, but is this the grand blueprint that is currently unfolding in history? Mark Zuckerberg: Sorry, in what sense?
Dwarkesh Patel: In this sense, there are other technologies, such as computers, and even fire, but the development of artificial intelligence itself is as important as human evolution.
Mark Zuckerberg: I think this is tricky. Human history is basically about people thinking that certain aspects of human nature are truly unique in different ways, and then accepting the fact that this is not true, but human nature is still very special. We thought the Earth was the center of the universe, but that's not the case, yet humans are still great, very unique, right?
I think another bias people tend to have is thinking that intelligence is fundamentally connected to life in some way. It's actually not clear if that's the case. I don't know if we have a clear enough definition of consciousness or life to fully examine this. There are all these science fiction stories about creating intelligence, and it starts to exhibit all these human-like behaviors and similar things. Currently, the embodiment of all these things feels like it's heading in a direction where intelligence can be quite separate from consciousness, agency, and similar things, I think that just makes it a super valuable tool.
Mark Zuckerberg: Obviously, it's hard to predict where these things will go over time, which is why I think no one should dogmatically plan how to develop it or plan what to do. You have to look at it with each release. We obviously strongly support open source, but I haven't committed to releasing everything we do. I'm basically very inclined to think that open sourcing is good for the community and good for us because we benefit from innovation. However, if at some point, there is a qualitative change in the capabilities of this thing, and we feel that open sourcing it would be irresponsible, then we won't open source it. It's all very hard to predict.
Balancing the Risks of Open Source
Dwarkesh Patel: If you see any specific qualitative changes when training Llama-5 or Llama-4, would it make you think "you know, I'm not sure if I should open source it"?
Mark Zuckerberg: It's a bit difficult to answer this question abstractly because any product can exhibit negative behaviors as long as you can mitigate those behaviors, it's fine. Social media has bad things, and we work hard to mitigate them. Llama-2 also has its downsides, and we've spent a lot of time ensuring that it doesn't help people engage in violent behavior or similar things. This doesn't mean it's autonomous or an intelligent being, it just means it has learned a lot about the world, it can answer some questions that we think it's not helpful for it to answer. I think the issue is not what behaviors it will exhibit, but what we can't mitigate after it exhibits those behaviors.
I think there are too many ways things can go good or bad that it's hard to list all these ways in advance. Look at the situations and various harms we have to deal with in social media. We've basically identified about 18 or 19 categories of harmful things people might do, and we've basically built AI systems to identify what these things are and try to ensure that these things don't happen on our network As time goes on, I think you can also break it down into a more detailed classification. I think this is something we spend time researching because we want to make sure we understand this.
Dwarkesh Patel: In my view, this is a good idea. If in the future, artificial intelligence systems are not widely deployed and accessible to everyone, I would be disappointed. At the same time, I want to better understand mitigation measures. If the mitigation measures are fine-tuning, the issue of open weights is that you can remove the fine-tuning, and fine-tuning is usually a superficial function on top of these capabilities. If it's like talking to biologists on Slack... I think the models are far from that. Right now, they are like Google search. But if I could show them my culture dish, and they could explain why my ceiling sample didn't grow and what needs to be changed, how do you mitigate this problem? Because someone can fine-tune directly in, right?
Mark Zuckerberg: That's true. I think most people would choose to use ready-made models directly, but there are also some unscrupulous individuals who may try to use these models for malicious purposes. On the other hand, one of the reasons I philosophically support open source is that I think if artificial intelligence becomes overly centralized in the future, its potential risks may be no less than its widespread dissemination. Many people are thinking, "If we can do these things, will the widespread application of these technologies in society be a bad thing?" At the same time, another question worth considering is whether it is a bad thing if an institution has more powerful artificial intelligence than everyone else?
I thought of a security analogy, there are so many security vulnerabilities in many different things. If you could go back one or two years, assuming you just had a couple more years of knowledge about security vulnerabilities. You could almost break into any system. This is not artificial intelligence. So believing that a very intelligent artificial intelligence might be able to identify some vulnerabilities, basically like a human can go back one or two years and disrupt all these systems, this is not entirely far-fetched.
So how do we as a society deal with this situation? An important part is open-source software, which allows when software is improved, it is not limited to a company's product, but can be widely deployed in many different systems, whether it's in banks, hospitals, or government things. As software becomes more powerful, it's because more people can see it, more people can tap it, there are some standards about how these things work. The world can quickly upgrade together.
I think in a world where artificial intelligence is widely deployed, it has been gradually strengthened over time, and all different systems will be constrained in some way. In my view, this is fundamentally much healthier than a more centralized situation. So there are risks in all aspects, but I think this is a risk that people don't talk about as much. The risk of artificial intelligence systems doing bad things. But what keeps me up at night is the risk of an untrustworthy actor having super powerful artificial intelligence, whether it's a hostile government, an untrustworthy company, or something else. I think this could be a much bigger risk Dwarkesh Patel: Because they have a weapon that no one else has?
Mark Zuckerberg: Or just creating a lot of chaos. My intuition is that due to economic, security, and other reasons, these things eventually become very important and valuable. If people or opponents you don't trust get something more powerful, then I think that could be a problem. Perhaps the best way to mitigate this situation is to have good open-source artificial intelligence, make it a standard, and be a leader in many ways. It just ensures that it is a more fair and balanced competitive environment.
Dwarkesh Patel: This seems reasonable to me. If this becomes a reality, that would be a future I prefer. I want to understand mechanistically, how does the fact that open-source artificial intelligence systems exist in the world prevent someone from using their artificial intelligence systems to create chaos? For example, with a specific example of someone carrying a biological weapon, would we do a lot of research elsewhere in the world to quickly find a vaccine? What happens?
Mark Zuckerberg: If you take the security issue I mentioned as an example, I think the likelihood of someone with weaker artificial intelligence trying to invade a system protected by stronger artificial intelligence is smaller. In terms of software security.
Dwarkesh Patel: How do we know that everything in the world is like this? What if biological weapons are not like this?
Mark Zuckerberg: What I mean is, I don't know if everything in the world is like this. Biological weapons are one of the areas that people who are most concerned about these types of things focus on, I think that makes sense. There are some mitigations. You can try not to train certain knowledge into the model. There are different approaches, but to some extent, if you encounter a very bad actor and you don't have other artificial intelligence to balance them and understand what the threat is, that could be a risk. This is one of the things we need to pay attention to.
Dwarkesh Patel: When deploying these systems, what can you see? For example, when you are training Llama-4, it deceives you because it thinks you haven't noticed something, and then you think, "Wow, what's going on?" It may be unlikely in a system like Llama-4, but can you imagine any similar situations that would truly worry you about deception, and billions of such copies spreading in the wild?
Mark Zuckerberg: What I mean is, right now we see a lot of illusions. More like this. I think it's an interesting question, how do you distinguish between illusions and deception. There are a lot of risks and things to consider. At least in running our company, I try to balance these long-term theoretical risks with what I actually think are quite real risks today. So when you talk about deception, the form I am most concerned about is people using it to create misinformation, and then spreading it through our network or other networks. The way we combat this harmful content is by building more intelligent artificial intelligence systems than the adversarial ones This is also part of my theory. If you look at the various harms people do or attempt to do through social networks, some are not very confrontational. For example, hate speech is not super confrontational in the sense that people have not become better in terms of racism. At this point, I think artificial intelligence is becoming increasingly complex overall, at a much faster pace than people are on these issues. We both have problems. People do bad things, whether trying to incite violence or something else, but we also have a lot of misinformation, basically things we shouldn't be censoring. I think it can be understandably frustrating for many people. So I think over time, having artificial intelligence that becomes increasingly accurate in this regard will be a good thing.
In these cases, I still consider the ability for our artificial intelligence systems to become more complex at a faster pace than them. It's an arms race, but I think we are at least currently winning this arms race. These are many things I have spent time thinking about.
Yes, whether it's Llama-4 or Llama-6, we need to think about the behaviors we observe.
Dwarkesh Patel: One reason you open source it is because many others are also researching this.
Mark Zuckerberg: So, yes, we want to see what others are observing, what we are observing, and what we can improve. Then we will assess whether it can be open sourced. But I am optimistic about our ability to do this in the foreseeable future. In the short term, I don't want to ignore the actual harm people are trying to do today using these models, even if they don't exist, but they pose quite serious daily harms that we are familiar with and run our services. In fact, I think this is also something we have to spend a lot of time on.
I find the thing about synthetic data really strange, and I'm actually interested in why you don't think like current models, why repeatedly synthesizing data might make sense. If they get smarter and adopt the kind of techniques I mentioned in my papers or blog posts, these techniques will be widely applied on the day of release, leading to the right chain of thought. Why wouldn't this form a loop?
Of course, this won't happen overnight, but will require months or even years of training. It may use more intelligent models that will become smarter, produce better outputs, and then become smarter again, and so on. I think this is achievable within the parameter range of the model architecture.
To some extent, I'm not sure, I think like today's 8 billion parameter models, I don't think you can be as good as the most advanced models with tens of billions of parameters that incorporate new research into the architecture itself. But these models will also be open source, but I think it depends on all the issues we just discussed.
We hope it will be like this. However, at each stage, just like when you develop software, you can do a lot with software, but to some extent, you will be limited by the chips running it, so there will always be different physical limitations. The size of the model will be limited by the energy you can obtain and use for inference So I am also very optimistic that these things will continue to improve rapidly.
I am more cautious than some people, I just think that a situation of being out of control is unlikely to happen. I think it makes sense to keep options open. There are too many unknowns we are facing. There is a case where maintaining a balance of power is really important. It's like there's an intellectual explosion, they like to win. Many things seem possible. Just as keeping your options open, considering all options seems reasonable.
Dwarkesh Patel: Meta as a big company. You can have both. As for the other dangers of open source, I think you have raised some really valid points about the issue of power balance, and the harms we can eliminate through better aligning technology or other means. I hope Meta has some kind of framework. Other labs have such a framework, they would say "if we see this specific thing, then it cannot be open sourced, and may not even be deployable." Just write it down, so the company is prepared, people expect it, and so on.
Mark Zuckerberg: Regarding existential risks, that's a good point. Now we are more focused on the types of risks we see today, more of these content risks, we don't want models to do things that help people commit violence, fraud, or harm people in different ways. Talking about existential risks may be more interesting intellectually, but I actually think that the real harms that need more attention to mitigate are when someone uses a model to harm others. In practice, for current models, I guess the next generation of models, and even the next next generation, these are the more common harms we see today, like people deceiving each other. I just don't want to underestimate that. I think we have a responsibility to make sure we do a good job in this area.
Dwarkesh Patel: Meta is a big company. You can have both.
Mark Zuckerberg: Right.
Views on the Metaverse
Dwarkesh Patel: Let's talk about something else. The Metaverse. Which period in human history would you most like to visit? From 100,000 years ago to now, do you just want to see what it was like back then?
Mark Zuckerberg: Does it have to be in the past?
Dwarkesh Patel: Yes, it has to be in the past.
Mark Zuckerberg: I am very interested in American history and classical history. I am also interested in the history of science. I actually think it would be interesting to see and try to understand more about how some major advances happened. About these things, we only have limited knowledge. I'm not sure if the Metaverse can allow you to do that, because it's hard to go back to things we don't have records of. I'm actually not sure if going back in time would be important. I think it would be cool for history classes and such, but that might not be the most exciting use case for the Metaverse as a whole The main thing is to be able to feel like you are together with others, no matter where you are. I think that would be fatal. In our conversation about artificial intelligence, much of the content is about all the physical constraints behind all of this.
I think one lesson of technology is that you want to move things from the realm of physical constraints to software as much as possible, because building and developing software is much easier. You can make it more democratic because not everyone will have a data center, but many people can write code and modify open source code. The goal of the metaverse version is to achieve a true digital existence. This will be an absolutely huge difference, so people won't feel like they have to be together for many things. Now I think that being together might have some better things. These things are not black and white. It won't be like, "Okay, now you don't need to do this anymore." But overall, I think this will be very powerful for social, connecting with others, work, certain parts of industry, medicine, and many other things.
Dwarkesh Patel: I want to go back to something you said at the beginning of the conversation. You didn't sell the company for $1 billion. About the metaverse, you know you want to do this, even if the market fiercely criticizes you for it. I'm curious. What is the source of this advantage? You said "values, I have this intuition," but everyone says that. If you were to say something unique to you, how would you express it? Why are you so sure about the metaverse?
Mark Zuckerberg: I think these are different questions. What drives me? We've talked about many topics. I just really like creating things, especially around how people communicate and understanding how people express themselves and work. I studied computer science and psychology in college, and I think many others in the industry studied computer science. So for me, the intersection of these two things has always been important.
It's also a very deep-seated drive. I don't know how to explain it, but I feel from the bottom of my heart that if I'm not creating new things, I'm doing something wrong. Even as we plan to invest $100 billion in artificial intelligence or pour huge amounts of money into the metaverse to develop business cases, we have plans, I think these plans are very clear, and if our stuff works, it will be a good investment. But you can't know from the beginning, and people have all sorts of arguments, whether with advisors or different people.
Dwarkesh Patel: Well, how can you, how do you have enough confidence to do this? You can't be certain from the beginning. People have all sorts of arguments, discussing with advisors or different people. How do you have enough confidence to do this?
Mark Zuckerberg: The day I stop trying to create new things is the day I'm done, I'll go somewhere else to create new things. I fundamentally can't operate something or in my own life, not trying to create new things that I find interesting. For me, whether we should try to create the next thing, that's not even a question. I just can't help it, I don't know **.
It's like this in all aspects of my life. Our family built this ranch in Kaoai, and I was involved in designing all these buildings. When we started raising cows, I thought, "Well, I want to raise the best cows in the world, so how do we design this ranch so that we can figure out and build everything we need to try to do. I don't know, that's just me.
Dwarkesh Patel: I'm not sure, but I'm actually curious about something else. At 19, you read a lot of ancient and classical works, both in high school and college. What important lesson did you learn from them? Not just the interesting things you discovered, but like... by the time you were 19, you hadn't consumed many tokens. Many of them were about classics. Obviously, this is important to some extent.
Mark Zuckerberg: You didn't consume many tokens... that's a good question. That's one of the things I find very interesting. Augustus became emperor and he tried to establish peace. There wasn't really a concept of peace at that time. People's understanding of peace was a temporary period between inevitable attacks from enemies. So you could get a brief respite. He had this view of shifting the economy from things like mercenaries and militarism to actually positive-sum games. It was a very novel idea at the time.
This is a very fundamental thing: The boundaries that people could imagine as rational ways of working at the time. This applies to both the metaverse and things like artificial intelligence. Many investors and others couldn't understand why we wanted to open source. It's like "I don't get it, it's open source. It must be just you making things proprietary for a temporary period, right?" I think this is a very profound thing in technology, it actually creates a lot of winners.
I don't want to overemphasize this analogy, but I do think that many times, there are patterns of building things that people usually can't understand. They can't understand how it could be valuable to people, or how it could be a rational state of the world. I think there are many more rational things than people imagine.
Dwarkesh Patel: That's very interesting. Can I tell you what I'm thinking? About what you might get from it? This may be completely wrong, but I think the key is that some people play very important roles at a very young age in empires. For example, Caesar Augustus, at the age of 19, was already one of the most important figures in Roman politics. He was leading battles and forming the Second Triumvirate. I wonder if at 19, you were thinking, "I can do this because Caesar Augustus did it."
Mark Zuckerberg: That's an interesting example, in many histories and in American history as well. One of my favorite quotes is this one from Picasso, that every child is an artist, the challenge is to remain an artist as you grow up. When you're young, it's easier to have crazy ideas. There are all these analogies to the innovator's dilemma in your life, and for your company or anything you build You are at an earlier position on the trajectory, so it's easier for you to turn and accept new ideas without breaking commitments to other things. I think this is an interesting part of running a company. How do you stay dynamic?
Open Source a $100 Billion Model
Dwarkesh Patel: Let's go back to the topic of investors and open source. A $100 billion model, assuming it's completely safe. You've done these evaluations, and unlike this example, evaluators can also fine-tune the model, hoping to do the same in future models. Would you open source this $100 billion model?
Mark Zuckerberg: As long as it's helpful for us, we will.
Dwarkesh Patel: But would it be helpful? $100 billion in R&D, now it's open source.
Mark Zuckerberg: That's also a question we need to evaluate over time. We have a long history of open source software, but we don't tend to open source our products, we won't open source Instagram's code.
We've adopted a lot of underlying infrastructure and open-sourced it. Perhaps our biggest one historically was our Open Compute project, where we took the designs of all our servers, network switches, and data centers and open-sourced them, and it turned out to be very helpful. While many people can design servers, the industry now revolves around our design standards, meaning the supply chain is basically built around our designs. This has increased production, made it cheaper for everyone, and saved us billions of dollars, which is great.
So, there are many ways in which open source could be helpful for us. One is if people figure out how to run the model more efficiently. Over time, we'll be spending tens of billions or more on all these things. So, if we can improve efficiency by 10%, we could save billions or even hundreds of billions. That alone could be very worthwhile. Especially if there are other competitive models out there, our stuff isn't just giving some crazy advantage away.
Dwarkesh Patel: So, do you think training will be commoditized?
Mark Zuckerberg: I think there could be many ways this evolves, and that's one of them. So, "commoditized" means it becomes very cheap because there are many options. Another direction it could evolve is qualitative improvement. You mentioned fine-tuning. Now, what you can do with fine-tuning other major models is very limited. There are some choices, but they usually don't apply to the largest models. Being able to do that, things for different specific applications or specific use cases, or building them into specific toolchains. I think this will not only lead to more efficient development but could also lead to qualitative differences.
Here's an analogy. I think a common problem in the mobile ecosystem is that you have these two gatekeeper companies, Apple and Google, that can tell you what you're allowed to build There is an economic version, where it's like we build something and then they take away a lot of your money. But there is also a qualitative version, which actually makes me more unhappy.
Many times, we have launched or wanted to launch some features, and Apple would say "No, you can't launch." That's bad, right? So the question is, have we built a world for artificial intelligence like this? You will have a few companies running these closed models, controlling the APIs, so they can tell you what you can build?
For us, I can say it's worth building a model ourselves to ensure we are not in that position. I don't want any other company to tell us what we can build. From an open-source perspective, I think many developers also don't want those companies to tell them what they can build.
So the question is, what is the ecosystem built around this point? What interesting new things are there? To what extent does this improve our products? I know in many cases, if this eventually becomes our database or caching system or architecture, we will receive valuable contributions from the community, which will make our products better. And then the work we do for specific applications still has such a big difference that it actually doesn't matter, right?
Perhaps the model ends up being more like the product itself, in which case I think whether it is open-source becomes a more complex economic calculation, as doing so largely commoditizes oneself. But from what I currently see, we don't seem to have reached that level yet.
Dwarkesh Patel: Do you expect to generate substantial income from licensing your model to cloud providers? So they would have to pay a fee to actually use that model.
Mark Zuckerberg: We hope to have such an arrangement, but I don't know how important it will be. It's basically our license for Llama, in many ways, it's a very permissive open-source license, we just have a restriction on the largest companies using it. That's why we set that restriction. We're not trying to stop them from using it. We just want them to come talk to us if they plan to basically take what we've built, resell it, and make money from it. If you are a company like Microsoft Azure or Amazon, if you plan to resell the model, then we should have a share in it. So come talk to us before you do it. That's how things evolve.
So for Llama-2, we have deals with basically all these major cloud companies, Llama-2 is available as a hosted service on all these clouds. I assume, as we release larger and larger models, this will become a bigger thing. It's not the main thing we're doing, but I think if these companies are going to sell our models, we should somehow share in the benefits, that makes sense.
Dwarkesh Patel: Regarding other dangers of open-source, I think you raised some really reasonable points about the issue of power balance and the harms we can eliminate through better aligning technology or other means I hope Meta has some kind of framework. Other labs have such frameworks, and they would say, "If we see this specific thing, we can't open source it, and we may not even be able to deploy it." Just write it down, so the company is prepared, people have expectations, and so on.
Mark Zuckerberg: Regarding existential risks, that's a good point. Now we are more focused on the types of risks we see today, more of these content risks. We don't want models to do things that help people commit violence, fraud, or harm people in different ways. Although discussing existential risks may be more intellectually interesting, I actually think the real harm that needs more attention to mitigate is someone using a model to harm others. In practice, for current models, I guess the next generation of models, and even the next-next generation, these are the more common harms we see today, like people deceiving each other. I just don't want to underestimate that. I think we have a responsibility to ensure we do a good job in this regard.
Dwarkesh Patel: As for open source, I am curious, do you think the impact of open source projects like PyTorch, React, Open Compute, etc., on the world could surpass Meta's impact in social media? I have spoken with users of these services, and they believe this possibility exists, considering that much of the internet's operation relies on these open source projects.
Mark Zuckerberg: Our consumer products do indeed have a huge user base globally, covering almost half of the world's population. However, I believe open source is becoming a new, powerful way of building. It may be like Bell Labs, where initially they developed transistors to enable long-distance calls, which they did achieve and brought them substantial profits. But 5 to 10 years later, when people look back at their proudest inventions, they may mention other technologies with more profound impacts.
I firmly believe that many of the projects we are building, such as Reality Labs, certain AI projects, and some open source projects, will have lasting and profound impacts on human progress. While specific products will evolve, emerge, and disappear over time, their contributions to human society will endure. This is an exciting part that we as tech professionals can collectively engage in.
Training Models on Custom Chips
Dwarkesh Patel: When will your Llama model be trained on your own custom chips?
Mark Zuckerberg: Soon, we are working hard to drive this process, but Llama-4 may not be the first model trained on custom chips. Our approach is to have custom chips handle our ranking and recommendation inference tasks first, such as Reels, news feed ads, etc. Once we can shift these tasks to our own chips, we can use more expensive NVIDIA GPUs to train more complex models In the near future, we hope to have our own chips. We can first use them to train some simpler things, and then eventually train these very large models. At the same time, I want to say that this project is progressing very smoothly, we are making steady progress, and we have a long-term roadmap.
If Mark Zuckerberg became the CEO of Google+
Dwarkesh Patel: One last question. This is completely off-topic, but if you were appointed as the CEO of Google+, could you make it successful?
Mark Zuckerberg: Google+? Oh. Well, I don't know. I don't know, it's a very difficult counterfactual.
Dwarkesh Patel: Okay, the real last question is: when Gemini was launched, did anyone in the office say "Carthago delenda est" (Carthage must be destroyed)?
Mark Zuckerberg: No, I think we're more gentle now. That's a good question. The issue is Google+ doesn't have a CEO. It's just a department within the company. You asked earlier what the scarcest commodity is, but you asked in terms of dollars. I actually think, for most companies of this scale, the scarcest commodity is focus.
When you're a startup, maybe you're more constrained financially. You're focused on one idea, you may not have all the resources. At some point, you cross a threshold and get to the essence of what you're doing. You're building multiple things, creating more value between them, but you become more constrained in the energy you can put into them.
There are always cases where great things happen in an organization randomly, and I don't even know about them. Those are great. But I think in general, an organization's ability is largely limited by what the CEO and management team can oversee and manage. This has always been a focus for us. As Ben Horowitz said, we should put the main thing first and try to focus on your key priorities.
Dwarkesh Patel: Very good, thank you very much. Mark, you're doing great