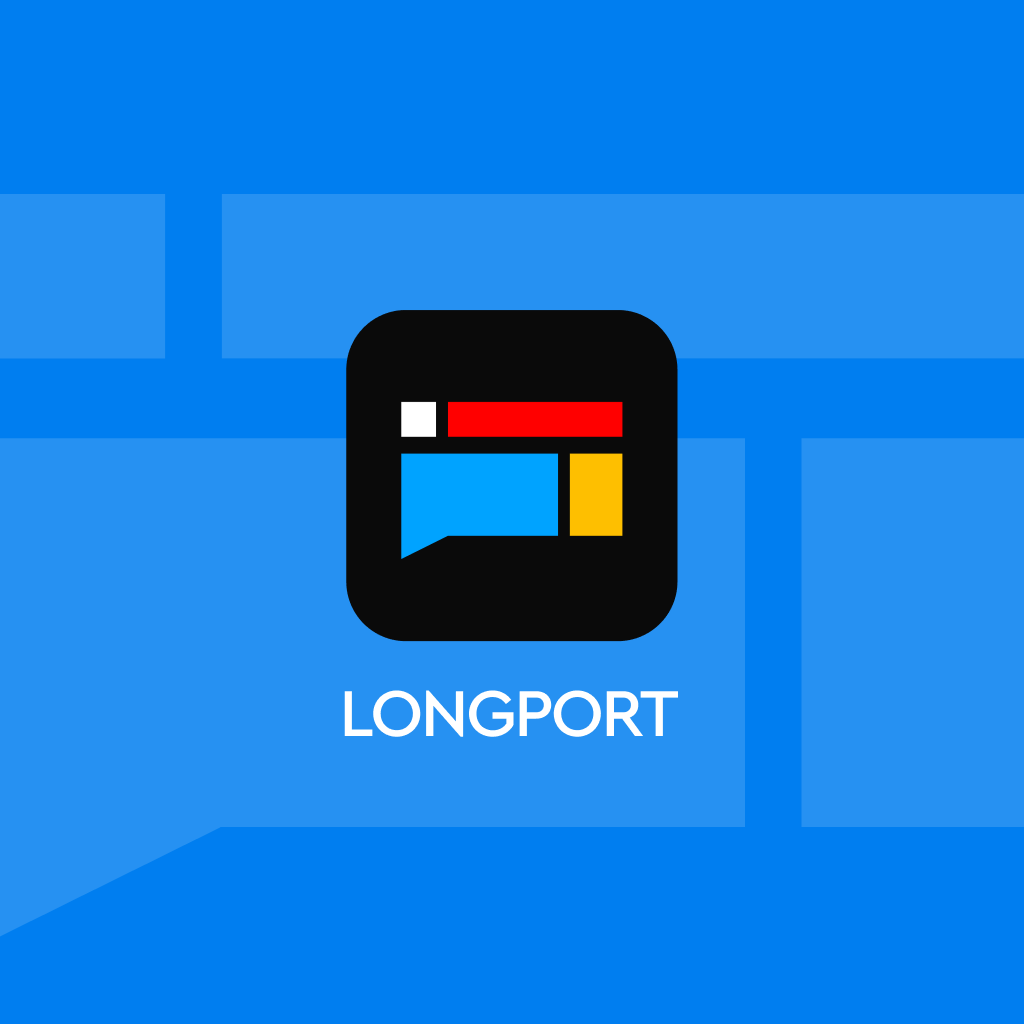
Groq CEO's latest interview: Deploying 1.5 million LPUs by the end of next year, dominating half of the world's inference demand
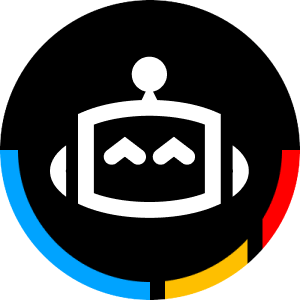
Groq CEO Jonathan stated that by the end of next year, they will deploy 1.5 million LPU chips, occupying more than half of the global inference demand. The market share of inference in the future is expected to grow significantly. Groq's chips have several times the advantage in inference speed and cost, compared to NVIDIA which excels in AI training but has limitations in inference. Groq's innovative architecture achieves performance improvements and avoids being constrained by the supply chain. AI inference is very sensitive to latency, with every 100 milliseconds of improvement leading to increased user engagement. In the next 1-2 years, Groq will deploy 1.5 million inference chips, potentially accounting for half of the global inference computing power
AI rising star Groq emerged, becoming the new favorite in the tech industry with its innovative LPU chip and astonishing processing speed.
Recently, venture capital fund Social Capital's founder and CEO Chamath Palihapitiya and Groq's founder and CEO Jonathan Ross engaged in a dialogue at the RAISE AI Summit. The discussion covered Groq's current development, thoughts on the future of AI, comparison between NVIDIA and Groq, Groq's advantages, and more.
Ross mentioned that the cost of AI inference is high, so his company specifically provides "super fast" and more affordable chip options for large models. By the end of next year, they will deploy 1.5 million LPUs, while NVIDIA deployed a total of 500,000 H100s last year. 1.5 million implies that Groq may account for half of the world's inference computing power. Key points of the dialogue include:
- Groq attracted 75,000 developers in over 30 days, while NVIDIA took 7 years to reach a developer scale of 100,000. Developers are crucial for the development of AI applications.
- NVIDIA excels in AI training but has limitations in inference. Groq's chips have several times the advantage in inference speed and cost. The market share of inference will increase from the current 5% to 90-95% in the future.
- Groq does not use advanced but limited-supply devices like HBM, instead achieving performance improvement through innovative architecture to avoid supply chain constraints.
- AI inference is very sensitive to latency and must be controlled within 300 milliseconds, while current systems often require several seconds or longer. Every 100 milliseconds of improvement can bring an 8% (desktop) to 34% (mobile) increase in user engagement.
- NVIDIA is a leader in the training field, but there is no clear winner in inference yet, making it very difficult to win in both markets.
- In the next 1-2 years, Groq will deploy 1.5 million inference chips, potentially accounting for half of the world's inference computing power, surpassing the total of major cloud computing manufacturers.
- The competition for AI talent in Silicon Valley is fierce, with astronomical salary costs. Groq's strategy is to recruit experienced engineers and have them learn AI knowledge.
- Large language models are like telescopes of thought, revealing the vastness of intelligence. Initially intimidating, but eventually we will realize its beauty and understand our position in it.
Below is the original dialogue translated by AI:
Chamath Palihapitiya: First of all, I am very happy to be here. I have some notes, so let's make sure we cover everything. I want to take this opportunity to introduce Jonathan. Obviously, many of you have heard of his company, but you may not know his entrepreneurial story. To be honest, he has been working in Silicon Valley for 25 years. This is one of the most unique origin stories and founder stories you can hear We will talk about his achievements at Google and Groq. We will compare Groq with NVIDIA, which I think may be one of the most important technical considerations that people should understand. We will discuss software, hardware, and provide some data and key points that I think are impressive.
So I want to start with something you do every week, which is that you usually post some developer metrics on Twitter. How was the situation this morning? Why are developers so important? Can you let me try this test?
Jonathan Ross: Our number of developers has reached 75,000, which is the number after more than 30 days since we launched the developer console. In comparison, NVIDIA took 7 years to reach 100,000 developers, while we reached 75,000 in just over 30 days. This is important because developers are building all the apps. So, each developer has a multiplier effect on the total number of users you can have, everything is about developers.
Chamath Palihapitiya: Let's review, in this context, this is not an overnight success story, this is 8 years of trekking in the wilderness, frankly accompanied by many mistakes, which is the hallmark of a great entrepreneur.
But I want everyone to hear this story, Jonathan, maybe you have all heard of those entrepreneurs who dropped out of college to start billion-dollar companies. Jonathan may be the only person who dropped out of high school and founded a billion-dollar company. Let's start with your background, because the path you took to become an entrepreneur is very tortuous.
Jonathan Ross: As I mentioned, I dropped out of high school and eventually found a job as a programmer. My boss noticed that I was smart and told me that even though I dropped out, I should go to college.
So I didn't formally enroll, but started auditing classes at Hunter College. Then I got guidance from a professor. I did well there, transferred to New York University, and started studying/Ph.D. program, but still as an undergraduate, and then I dropped out again.
Chamath Palihapitiya: Technically, do you have a high school diploma? Jonathan Ross: No. I also don't have a bachelor's degree.
Chamath Palihapitiya: So how did you go from New York University to Google?
Jonathan Ross: In fact, if it weren't for New York University, I don't think I would have gone to Google. It's interesting that even though I didn't have a degree, I happened to attend an event at Google. Someone from Google recognized me because they also went to New York University, and they recommended me. So even if you don't graduate, you can build some great connections in college, but that was one of the people I took the Ph.D. course with.
Chamath Palihapitiya: What were you doing when you first went to Google? Jonathan Ross: Advertising, but mainly testing, we are building a huge testing system. If you think building a production system is difficult, the testing system must test everything the production system does. Therefore, for every ad query, we have to run 100 tests, but we don't have the budget for a production system. We had to write our own threading library. We had to do all sorts of crazy things, which in the advertising industry is unthinkable, but in reality, it's even harder than the production system itself.
Google encourages employees to spend 20% of their regular working hours on projects they believe will benefit Google, where you can do anything you want. I call it mci time, as long as it's not during working hours, you can work on it for 20% of the time. But every night, I go up and work with the speech team. This is separate from my main project, and they bought me some hardware.
I started a sub-project called TPU. Its funding came from what a vice president called the "small treasury" or surplus funds. No one ever expected it to succeed. In fact, there were two other projects developing AI accelerators, and no one expected them to succeed, which gave us the cover we needed to do some truly abnormal, intuitive, and innovative things.
Chamath Palihapitiya: Stepping back. When these words were not yet in use, what problems did you want to solve in AI? And when you saw the opportunity, what was Google trying to do?
Jonathan Ross: It all started in 2012. At that time, no machine learning model had ever outperformed humans on any task. The speech team trained a model that could transcribe speech better than humans. The problem was they couldn't afford to put it into production. So, a very famous engineer, Jeff Dean, made a demo to the leadership team with just two slides.
The first slide was good news. Machine learning worked. The second slide, bad news. We can't afford it. They would have to double or triple the space of Google's entire global data centers. The average cost per data center is $1 billion, with 20 to 40 data centers, so it would require $20 to $40 billion. This was just for speech recognition. If they wanted to do other things, like search ads, the cost would be even higher. It was economically infeasible, that's the history of reasoning, you train it, and then you can't afford to put it into production.
Chamath Palihapitiya: In this context, what did you do? What made TPU one of the actual winning projects?
Jonathan Ross: Most importantly, Jeff Dean noticed that the main algorithm consuming most of Google's CPU cycles was matrix multiplication. We decided to accelerate that, but let's build something around it, we built a huge matrix multiplication engine When doing this, there were two other competing teams who took a more traditional approach to do the same thing. One of them was led by a Turing Award winner, and then we proposed the so-called pulsating array. I remember that Turing Award winner talking about TPU and saying that the person who came up with this idea must be very old because pulsating arrays are no longer popular. In fact, it was me who proposed it, I just didn't know what a pulsating array was at the time, and someone had to explain this term to me. It was just the obvious way to do this.
So the lesson is, if you know how to do something, you might know how to do it the wrong way. It is very helpful to involve those who do not know what should be done and what should not be done.
Chamath Palihapitiya: With the expansion of TPU, there may be a lot of recognition within Google. Why would you give that up?
Jonathan Ross: All big companies eventually become politicized? When you have something so successful, many people want to have it, and more senior people start fighting for it. I moved to the Google X team, a team that quickly evaluates all the crazy ideas at Google X, where I had a lot of fun, but nothing turned into a production system.
I wanted to start from scratch and do something real from start to finish. I wanted to turn some concepts into works. I started looking outward, that's when we met.
Chamath Palihapitiya: But the problem is, you had two ideas. One was more about building an image classifier that you thought could surpass the best ResNet at the time, and then you had this hardware path.
Jonathan Ross: What happened was, I also built the highest performing image classifier. I noticed that all the software was provided for free. TensorFlow was free, the models were free, it was clear that machine learning AI would be open source, even at that time, in 2016.
I just couldn't imagine building a business around it, it would be a tough chip business. Building them takes a long time, and if you build something innovative and launch it, it would take anyone 4 years to replicate it, let alone surpass it. So it felt like a better way, it was atomic, you could monetize it more easily.
That would just be hard spelling chips. Making these chips takes a long time, and if you manufacture an innovative product and launch it, then four years later someone will imitate your product. Let alone surpass it. So I think this approach is better. You can monetize it more easily. Just then the TPU paper was published, my name appeared in it and people started asking what different approach you and I would have.
Just then, the TPU paper was published. My name was on it, and people started asking about it.
Chamath Palihapitiya: At that time, I was also investing in the public market. It was a bit crazy in the public market. PiChai mentioned TPU in a press release, I was very shocked, I thought Google shouldn't build its own hardware They must know something that the rest of us don't.
We need to know this so that we can commercialize in other parts of the world. I may have met you in a few weeks, which may be the fastest investment I have ever made. I remember the key moment when you didn't have a company. We had to set up the company after the check was issued, which was a completely foolish sign, or in 15 or 20 years, you will either look like a genius, but the latter is very unlikely.
You founded this company, telling us about the design decisions made at Groq at that time, based on your understanding at the time, because the situation was very different then.
Jonathan Ross: Again, when we started fundraising, we weren't actually 100% sure we would do something in hardware. I think you asked some questions, like what different things you would do. My answer was software, because we could manufacture these chips at Google, but every team at Google had a specialist manually optimizing models.
I thought it was crazy at the time, and then we started hiring some people from NVIDIA, and they said, no, you don't understand, we do it too, that's how it works. We do it too. We have these things called kernels, CUDA kernels. Yes, we manually optimize them. We just make it look like we don't. But in terms of scale, like the algorithms and big O complexity you all understand, it's linear complexity. For every application. You need an engineer, NVIDIA now has 50,000 people in their ecosystem.
So we focused on compilers in the first 6 months. We banned the use of whiteboards at Groq because people kept trying to draw pictures of chips.
We didn't know what it would be. But the inspiration came from the last thing I did, which was running DeepMind's AlphaGo software on TPUs, and seeing that, it was obvious that reasoning would be a scale issue. Others have always seen reasoning as taking a chip and running a model on it. It runs anything. But what happened with AlphaGo is that we ported the software. Despite having 170 GPUs compared to 48 TPUs, 48 TPUs still won 99 out of 100 games with the exact same software. This means that computation will bring better performance. Insight is what allows us to build scalable reasoning. We built interconnects, we built for scale.
That's what we're doing now. When we run one of these models, we have hundreds or thousands of chips contributing, just like we did with AlphaGo, but it's built for this, not pieced together.
I think this is a great springboard, many people think this company deserves a lot of respect, but NVIDIA has been working hard for decades. They have obviously built an incredible business. But in some ways, when you delve into the details, the business is slightly misunderstood Chamath Palihapitiya: So can you break it down first? Where is NVIDIA naturally good at? Where are they trying to excel more?
Jonathan Ross: The classic saying is that you don't have to outrun the bear, you just have to outrun your friends.
NVIDIA surpasses all other chip companies in terms of software, but they are not a software-first company. They actually have a very expensive approach, as we have discussed. But they have an ecosystem, which is a two-sided market. If you take a kernel-based approach, they have already won, with no followers, so we adopted a non-kernel approach. But another way they excel at is vertical integration and forward integration. NVIDIA repeatedly decides to elevate the stack, no matter what the customers are doing, they start doing it.
For example, I think it was Gigabyte or some other PCI board manufacturers recently announced that despite 80% of their revenue coming from NVIDIA, NVIDIA's graphics cards are what they manufacture, but they exited this market. Because NVIDIA moved up and started doing something with lower profit margins.
I think another thing is that NVIDIA is very excellent in training, the design decisions they make include things like HBM, it really revolves around the world at that time, everything is about training. There are no real-world applications, none of you are really building anything out in the field where you need super-fast inference, I think that's another.
We see time and time again, you will spend 100% of the computation on training. You will get something good enough for production. Then it flips to about 5% to 10% training and 90% to 95% inference. But the amount of training will remain constant. Inference will grow massively. So every time we succeed at Google, suddenly we hit a disaster, we call it a success disaster, we can't afford enough computation for inference because it will immediately increase by 10 times, 20 times.
If you multiply this 10 times, 20 times by the cost of NVIDIA's leading solution, you are talking about a huge amount. So maybe explain to everyone what HBM is, why these systems are like. But NVIDIA just announced the B200, complexity and cost. In fact, if you try to do something. Complexity spans every part of the stack, but the supply of a few components is very limited.
NVIDIA has locked in these markets, one of which is HBM, which is high-bandwidth memory, which is necessary to achieve performance. Because the speed at which you run these applications depends on how fast you read that memory. This is the fastest memory. Supply is limited, it is only used in data centers. They cannot touch the supply of mobile devices or other things like other components, and there are also packaging substrates. In addition, NVIDIA is the largest buyer of supercapacitors in the world, as well as various other components, cables, cables, 400 Gbps cables, they have all bought out If you want to compete, no matter how good the product you design is. They have bought the entire supply chain for years.
Chamath Palihapitiya: So what do you do?
Jonathan Ross: You don't use the same things they use, that's where we come in.
So how do you design chips? If you look at the leading solutions, they are using something, they have obviously been successful, what would you do? Is this just a completely orthogonal and different technological bet? Or is there something very specific that you say we can't rely on the same supply chain, because we will eventually be forced out of this industry.
In fact, there was a very simple observation at the beginning, that is, the performance differences in most chip architectures are very small, like 15% is considered great.
We realized that if we are just good by 15%, no one will switch to a completely different architecture. We need 5 to 10 times, so the small percentage points you get by chasing cutting-edge technology are irrelevant. So we used an older technology, 14 nanometers, which was not fully utilized. We didn't use external memory. We used older interconnects because our architecture needs to provide an advantage, it needs to be so powerful that we don't need to be at the forefront.
Chamath Palihapitiya: So how do you measure speed and value today? Give us some comparisons of the Llama, Mistral, and other models that you and others may be running.
Jonathan Ross: So we compare from two aspects. One is the number of tokens per dollar, and the other is the number of tokens per user per second. The number of tokens per user per second is the experience. That's the difference. The number of tokens per dollar is the cost, and of course there is also the number of tokens per watt, because power is currently very limited. If you compare us to GPUs, we are usually 5 to 10 times faster, like Apple to Apple, without using speculative decoding and other things.
So now, on a model with 180 billion parameters, we run about 200 tokens per second, I think this is less than 50 of the next generation GPU that NVIDIA is about to launch. Your current generation is 4 times better than the B200. And in terms of total cost, we are about 1/10 of a modern GPU. Per token. I want to drive this point home, 1/10 of the cost. I think the value really comes down to, you will have ideas, especially if you are part of the venture capital world and ecosystem, you raise funds, people like me will give you money, hoping you invest wisely.
In the past decade. We have entered a very negative cycle, where almost 50 cents of every dollar we give to startups will end up back in the hands of Google, Amazon, and Facebook. You spend money on computing, you spend money on advertising This time, the power of artificial intelligence should be that you can build a company at 1/10 or 1/100 of the cost. But if you put the money back again, it will not be possible, just this time it will be to NVIDIA, not someone else. So we will strive to ensure that this is a low-cost alternative. A few weeks ago, NVIDIA made a major announcement. They showed a chart pointing upwards and to the right. They displayed a huge chip, they displayed a huge package. They told us about the situation of B200 and compared it to what you are doing now.
First of all, B200 is a miracle in engineering, in terms of complexity, integration, and the number of different components in silicon, they spent $10 billion developing it. But when it was released, I received some messages from NVIDIA engineers, they said that we felt a bit embarrassed about their claim of 30 times performance because it is far from that high. As engineers, we feel that this damages our credibility. The claim of 30 times. Let's take a look. There is a picture showing that in terms of user experience, it can reach up to 50 tokens per second at most, with a throughput of 140, which gives the value or cost. If you compare it to the previous generation product, that is, if you divide 50 by 30, the number of tokens per second that users get is less than 2, which would be very slow, right? Nothing runs that slow.
Then from the perspective of throughput, this would make the cost unbelievably high.
We realize that if our performance improves by 15%, no one will switch to a completely different architecture. What we need is a 5 to 10 times improvement, so the slight improvements gained from chasing cutting-edge technology are not important. Therefore, we used an older technology, namely 14-nanometer technology, which has low utilization. We did not use external memory. We used older interconnect technology because our architecture needs to provide an advantage, and it needs to be overwhelmingly advantageous so that we do not need to be in the lead. So.
Chamath Palihapitiya: Why don't you break down this difference now? Because I think this is a good place to really understand the huge difference between training and inference. What is needed, why don't you define the difference, and then we can compare where things are heading.
Jonathan Ross: The biggest difference is that when you are training, the number of tokens you train is measured in months. For example, how many tokens can we train this month? It doesn't matter if a batch takes one second, ten seconds, or one hundred seconds, what matters is how many batches there are per month. In inference, what matters is how many tokens you can generate per millisecond or a few milliseconds, right? Not in seconds, not in months.
Chamath Palihapitiya: So, can it be said that NVIDIA is a model for training? And can it be said that there is not yet a truly scalable winner in inference, not yet. Do you think it will be NVIDIA? Jonathan Ross: I don't think it will be NVIDIA.
Chamath Palihapitiya: But specifically, why do you think it's not suitable for that market? Even though it obviously works well in training.
Jonathan Ross: In order to reduce latency, what we have to do is, we have to design a completely new chip architecture. We have to design a new network architecture, a new system, a new runtime, a new compiler, a new orchestration layer. We have to throw everything away, it has to be compatible with what PyTorch and others have actually developed.
What we are talking about now is the innovator's dilemma on steroids. It's hard to give up one of them, if you succeed in one of them, it will be a very valuable company. But throwing away all six of them is almost impossible. And, if you want to continue training, you also have to maintain what you have now. Now, you have to have a completely different architecture for networks, chips, networks, everything, for training and inference.
Assuming today's market is 100 training units or 95 training units, 5 inference units. I should say, this is probably where most of the revenue and dollars come from. What will it look like in 4 to 5 years?
In fact, 40% of NVIDIA's latest revenue comes from inference, and it's already starting to climb. It will eventually reach 90% to 95%. This trajectory will take off rapidly because now we have these open-source models that everyone is offering for free. You can download a model and run it, you don't need to train it.
One issue with building useful applications is that you have to either understand or be able to work with CUDA. It doesn't even matter because you can port directly.
Chamath Palihapitiya: So maybe explain to everyone the importance of being able to quickly replace these models in the inference market, and where you think these models are headed.
Jonathan Ross: For the inference market, there is a brand new model that needs to run about every 2 weeks. This is important. Either it sets the gold standard in every way, or it excels in a specific task. If you're writing kernels, it's almost impossible to keep up. In fact, when the Llama 27 billion parameter model was released, it was officially supported by AMD, but the first actual support we saw was achieved about a week later, and we achieved it in about 2 days.
So that speed, like now, everyone is developing for NVIDIA hardware. So by default, anything that is released can work there. But if you want anything else to work, you can't manually write these kernels. Remember, AMD has official support, and it still took about a week, right?
If you are starting a company today, you obviously want to be able to switch from Llama to Mistral as needed, then switch to Anthropic, you want to switch to the latest Chamath Palihapitiya: As someone who has seen these models in operation, what do you think of the quality of these models? Where do you think some of these companies are heading, or what have you seen? Some are doing well, some are doing poorly?
Jonathan Ross: They are all starting to catch up with each other, showing some leapfrog development. Initially, GPT-4 was far ahead, leading others by about a year. Now, Anthropic has caught up. We see that Mistral has performed well in all aspects.
So, an interesting phenomenon is that, especially Mistral, has been able to approach quality with smaller and cheaper models, which I think gives them a huge advantage. I find Cohere's approach to optimizing models also interesting. So, people are looking for niches. At the high end, there will certainly be a few products that are the best. But what we see is that many people complain about the cost of running these models. They are astronomical. You can't scale applications for users with them.
Chamath Palihapitiya: OpenAI has disclosed the total GPU capacity of Tesla and several other companies. You can infer how big the market will be, because as your scale grows, the inference market can really only be supported by them. Can you give people an idea of the scale that people are striving for?
Jonathan Ross: Facebook announced that by the end of this year, they will have the equivalent of 650,000 H100 GPUs. By the end of this year, Groq will deploy 100,000 of our LPUs, exceeding H100 in throughput and latency. We are likely to reach Facebook's level. By the end of next year, we will deploy 1.5 million LPUs, last year, Nvidia deployed a total of 500,000 H100s. So, 1.5 million means that Groq may have AI generative inference capabilities that exceed the sum of all super-large-scale cloud service providers, possibly accounting for half of the world's inference computing power.
Chamath Palihapitiya: Great. Please talk about team building in Silicon Valley. In the context of "you can work at Tesla, you can work at Google, you can work at OpenAI," how difficult is it to find real AI talent?
Jonathan Ross: By the way, you have an interesting thing, because with your initial chip, you were trying to find people who understand Haskell. So tell us, how difficult is it to build a team in Silicon Valley to do this, it's impossible.
So if you want to know how to do this, you have to start getting creative, just like anything you want to do well, don't compete directly. But these salaries are astronomical, because everyone thinks it's a winner-takes-all market. I'm like, isn't this about me becoming second place? Will I be third? They're all saying I must be the first. If you don't have the best talent, you're out.
Now, the mistake is. Many AI researchers are very good at AI, but they are still a bit green. They are very young. Right? This is a new field. I always advise people to hire the most experienced, rugged engineers who know how to deliver things on time, let them learn AI, because they will learn AI faster.
And then you will be able to train AI researchers with 20 years of experience in deploying code.
Chamath Palihapitiya: You appeared on stage with Saudi Aramco in Saudi Arabia a month ago and announced some big deals. Can you tell us what these deals are? What is the direction of the market? Are you competing with Amazon, Google, and Microsoft? Is that so?
Jonathan Ross: This is not competition. It's actually complementary. The announcement is that we will reach a deal with Aramco Digital. We have not announced the specific scale yet, but in terms of the amount of computing power we are going to deploy, the scale will be large. Overall, we have reached a deal that exceeds 10% of the 1.5 million LPU target. The difficult part is the first deal.
Once we announce that many other deals are being reached, but the scale of these deals is that they have more computing power than Meta and many other tech companies. Now, they think they have this advantage because they have locked in the supply. They do not want another choice to exist. So we are actually making deals with those who will have more computing power than super-scale cloud service providers.
Chamath Palihapitiya: This is a crazy idea. One last question. Everyone is worried about what AI means. You have been in this industry for a long time, so finally talk about your views, what we should think about, and your views on the future of AI, our future work, and all the things people usually worry about.
Jonathan Ross: So I am often asked, should we be afraid of AI? My answer is, if you think of Galileo, a man in trouble, the reason he got into trouble was that he invented the telescope, promoted it, and claimed that we are much smaller than everyone imagined, and that we should be the center of the universe. It turns out we are not, the better the telescope, the more obvious it is how small we are. To a large extent, large language models are like telescopes of thought. It is obvious that intelligence is much greater than us. This makes us feel very small. It's scary. But over time, as we realize that the universe is much larger than we imagined, we get used to it. We start to realize how beautiful it is, our position in the universe. I think that's what's going to happen. We will realize that intelligence is much broader than we imagined, we will understand our place in it. We will not be afraid of it
This is a great way to end. Jonathan Ross, everyone. Thank you all. Much appreciated. Someone told me that Groq means to deeply understand with empathy, which embodies this definition