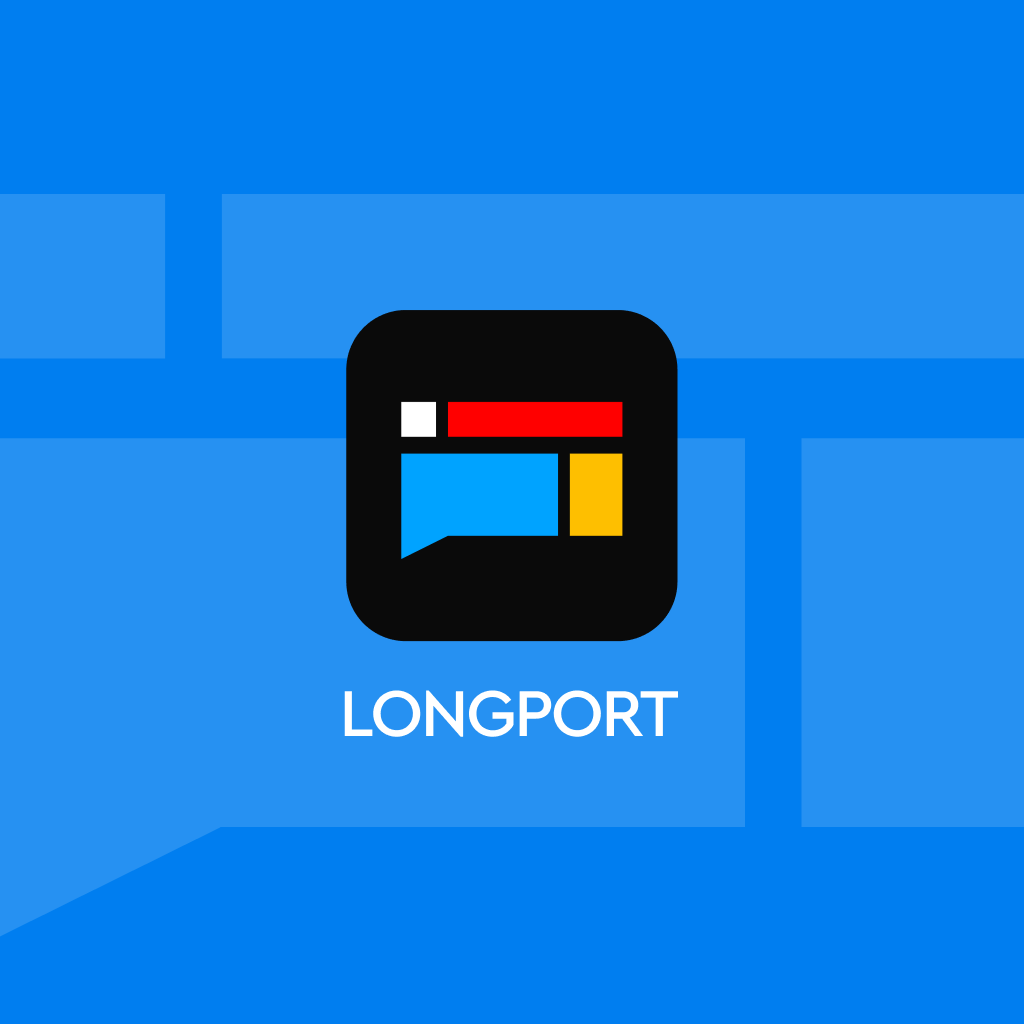
Lao Huang: Computing power will increase by another 1 million times in the next 10 years
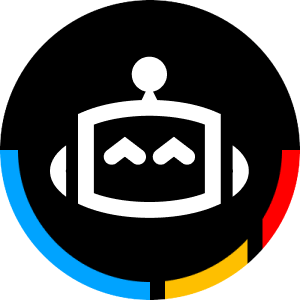
Huang Renxun: Liquid cooling technology will become the next trend in AI computing power. In the future, computer calculations will rely heavily on generation rather than retrieval
Comprehensive insights from intelligent investors.
In early March 2024, NVIDIA CEO Jensen Huang returned to his alma mater, Stanford University in the United States, to participate in the SIEPR Economic Summit at the Stanford Graduate School of Business and the View From The Top series of events.
In two public replay videos, Jensen Huang detailed NVIDIA's market positioning, the development of AGI (Artificial General Intelligence), the growth of AI computing power, and how AI can be rooted in human values through human feedback.
He did not touch on topics like "AI ends with photovoltaics and energy storage" that were trending online over the weekend, in fact, we couldn't even find the original source.
But these two dialogues contained a lot of information.
Jensen Huang admitted that AI technology is narrowing the technological gap for humanity.
He mentioned that currently about 10 million people have jobs because they know how to program, leaving the other 8 billion people "behind", and in the future, if generative AI gradually replaces programming, programming skills may become less valuable.
"AI and the future of human communication are no different. This is the great contribution of the computer science industry to the world. We are narrowing the technological gap," Jensen Huang said.
Regarding AI computing power, Jensen Huang emphasized that in the next 10 years, NVIDIA will increase the computing power for deep learning by another 1 million times, allowing AI computers to continuously train, reason, learn, apply, and continuously improve, transforming the concept of super AI into reality in the future.
"Therefore, we will do more computing. We will reduce the marginal cost of computing to near zero," Jensen Huang said.
In another Stanford dialogue, Jensen Huang discussed the process of founding NVIDIA and obtaining funding, pointing out that "we are in a world of computing."
Faced with the "low point" when NVIDIA plummeted by 80%, Jensen Huang admitted that at that time he hoped the company would return to the "core" of things—to stick to what I believe in, and then change nothing and continue moving forward.
Jensen Huang also believes that liquid cooling technology will become the next trend in AI computing power. The future computation of computers will heavily rely on generation rather than retrieval.
He mentioned, "If your definition of AGI is passing human tests, then I will tell you, in five years we will pass all tests."
Thanks to Titanium Media for the complete translation and revision, we have condensed the transcripts of these two dialogues totaling 23,000 words and shared the most noteworthy information with everyone. Some individual questions referenced the translation from the WeChat official account Information Equality.
01 His first job before becoming CEO was a dishwasher
Question: At that time, you joined LSI Logic (an electronics company headquartered in San Jose, California, USA), which was one of the best companies at the time. What was the reason for leaving to start your own business? Huang Renxun is a friend of Chris and Curtis (the two co-founders of NVIDIA). At that time, I was working as an engineer at LSI, while they were at Sun. I was working with the smartest people in the field of computer science, creating various workstations including graphic workstations.
One day, Chris and Curtis mentioned that they wanted to leave Sun. They asked me for ideas on what to do next.
Before becoming CEO, my first job was as a dishwasher, which I excelled at. In any case, we often gathered together, and that period coincided with the microprocessor revolution.
It was around 1992 to 1993, right at the beginning of the PC revolution. The revolutionary Windows 95 had not been released yet, and even the Pentium processor had not been launched. All of this happened before the explosion of the PC revolution, and it was evident that microprocessors would be very important.
So we thought, why not establish a company to solve problems that general-purpose computers couldn't solve?
This became the mission of the company: to manufacture special computers to solve problems that general-purpose computers couldn't solve. And to this day, we have remained focused on this.
Look at the markets we have pioneered and the various problems in those markets, such as computer-aided drug design, weather simulation, material design, robotics, autonomous driving cars, and artificial intelligence autonomous software. We have continuously driven technological advancements, ultimately reducing computing costs to near zero.
This has led to a whole new way of software development, where computers write software themselves, which is what we now know as artificial intelligence. That's it.
Q: At that time, the CEO of LSI convinced his biggest investor, Don Valentine (known as the godfather of Silicon Valley venture capital, founder of Sequoia Capital), to meet with you. How did you convince Silicon Valley's hottest investor to invest in you?
Huang Renxun: Laurie and I only had about six months' worth of living expenses in the bank at that time. We already had Spencer and Madison, as well as a dog, so the five of us could only rely on this small amount of savings to get by.
Therefore, I didn't have much time. I didn't write a business plan but went directly to Wilfred Corrigan (founder and CEO of LSI, and also a former president and CEO of Fairchild Semiconductor).
He then called Don Valentine and said, "Don, I'm sending you a young man. I hope you can invest in him. He is one of the best employees at LSI."
The lesson I learned is: You can ace an interview, or you can mess it up, but you can't escape your past, so make sure you have a good "past."
In many ways, when I say I was a good dishwasher, I mean it. I might have been the best dishwasher in the history of Denny's restaurant.
I had a plan, I was organized, I worked diligently, and I cleaned the dishes with all my heart. After that, I was promoted to a waiter, and I was the best waiter at Denny's I never leave my workspace empty-handed, nor do I return empty-handed. I am very efficient. In the end, I became the CEO, but I am still striving to be an excellent CEO.
Source: Internet
02 Everything is about creating technology and exploring the market
Q: When a company's funds are only enough to sustain it for 6 to 9 months, how do you decide on the next steps to save the company?
Huang Renxun: We founded the company "Accelerated Computing" (NVIDIA). The question was, what is it used for? What is its killer application? This was our first major decision and also a project of Sequoia Capital.
Our first major decision was that the primary application area would be 3D graphics. The technology would be 3D graphics, and the specific application would be electronic games.
At that time, cheap 3D graphics technology was impossible. Silicon Valley graphics chip products cost millions of dollars, making it difficult to create a low-cost version. And the value of the electronic game market at that time was zero dollars, non-existent.
You have a technology that is difficult to commercialize, targeting a market that does not yet exist. This intersection is the starting point of our company.
I still remember what Don said after my presentation, which made sense at the time and still does today: "A startup should not invest in or collaborate with another startup."
His point was, for NVIDIA to succeed, we needed another startup to succeed as well, which was Electronic Arts.
We realized that in order to commercialize million-dollar computer graphics technology and make it compatible with computers priced at $300, $400, $500, you not only need to create new technology but also invent new ways of computing graphics processing.
At the same time, you also need to explore entirely new markets. Therefore, we must constantly create new technology and new markets.
This philosophy of "creating technology and exploring the market" defines our company. Almost everything we do is about creating technology and creating markets. This is the essence of what people call an "ecosystem."
Over the past 30 years, NVIDIA's core insight has been: In order for others to buy our products, we must personally develop this new market.
Q: When the products we were making were incompatible with Microsoft's Direct 3D standard, how did we deal with it?
Huang Renxun: We had to change tracks, otherwise we would have to close down. But we didn't know how to build it the Microsoft way.
I remember the discussion at that meeting: We now have 89 competitors, we know the previous way was wrong, but we don't know what the right way is.
Fortunately, one weekend I took my daughter Madison to the bookstore, and then I saw this book, the OpenGL manual, which defined the computer graphics processing method of Silicon Valley graphics. It was $68, and I brought a few hundred dollars, bought three books There is a large fold-out insert in the middle, which is the OpenGL pipeline computer graphics processing pipeline. I handed it over to those geniuses who co-founded the company with me.
We implemented the OpenGL pipeline in a way we had never done before, creating something the world had never seen.
There were many lessons learned. For our company, that moment gave us great confidence: even if we know nothing about what we are doing, we can still successfully create the future.
Now this is my attitude towards anything. When someone tells me something I haven't heard of, or heard of but don't understand the principles, my thought is always: how hard can it be? Maybe reading a book will solve it, maybe reading a paper will clarify the principles.
Even with today's company, I often go back to basics and rethink from scratch. The way we think about software and computers today is constantly changing. Often prompting the company and myself to return to the essence of the problem will create a lot of opportunities.
Source: Internet
03 Focus on the "importance of work" as the most core issue
Q: When the apple finally falls from the tree, and you are wearing a black leather jacket waiting to catch it, how do you do it with such certainty?
Huang Renxun: It always feels like a diving catch, just like a diving catch. Your actions stem from core beliefs.
We believe that we can create a kind of computer that can solve problems that general computing cannot solve. We believe that the capabilities of CPUs are limited, and so are the capabilities of general computing. At the same time, we also know that we can solve some interesting problems.
But are these problems just interesting? Or can they expand into interesting markets? Only when they become markets can sustainability be guaranteed.
NVIDIA has invested in the future for ten years, but the market does not exist. At that time, there was only one market: computer graphics.
For more than a decade, the market that has driven our growth today simply did not exist. So, how do you continue to lead everyone around you: the company, the management team, excellent engineers, shareholders, the board of directors, and partners?
You are taking everyone on the road, but there is simply no evidence of the market's existence. This is really very, very challenging.
We have a phrase called EIOFS, which stands for "Early Indicators of Future Success." I often use this term, it can help people and give companies hope.
Host: What early indicators have you used?
Huang Renxun: There are all kinds. I once saw a paper where I met someone who needed my help in the field of "deep learning" long before. At that time, I didn't even know what deep learning was.
They needed us to create a domain-specific programming language so that all their algorithms could easily be implemented on our processor.
We created something called KU-DNN. It is essentially the SQL (database language) of the deep learning field. And SQL is applied in storage computing We have created a programming language for deep learning, just like OpenGL in this field. They need us to do this so that they can express their mathematical calculations.
They don't understand CUDA, but they understand deep learning. We created this tool for them in between.
The reason we did this is because even when the market size was zero at the time... these researchers were penniless, even if they couldn't see any financial returns, and it seemed distant, as long as you believe, the company is willing to do it.
From the very beginning, we have always focused on the importance of work rather than market size. Because the importance of work is an early indicator of the future market's existence.
We should do things that "will cause problems if we don't do them."
Host: During the financial crisis, the company's market value evaporated by 80%, experiencing a very difficult period. How did you control the situation and keep employees focused on the goal in that situation?
Huang Renxun: My reaction during that time was completely the same as in the past week.
Of course, it was a bit embarrassing when the stock price dropped by 80%. You just want to wear a T-shirt that says "It's not my fault" when you go out. Even worse, you don't want to get up, don't want to go out. These are all very real, but then you still have to focus on work.
I woke up at the same time, planned my day in the same way. I returned to my original intention: what do I believe in? You must always remember the core, what do you believe in? What is the most important thing? Confirm one by one.
Doing this is helpful. Does my family love me? Yes, very much. You have to confirm each one. Then return to the core of your work and continue working.
And then every conversation goes back to the core of work, keeping the company's attention focused on the core.
Do you believe? Has anything changed? Has the stock price changed but has anything else changed? Have the laws of physics changed? Has gravity changed? Have the things that prompted us to make decisions, those assumptions, those beliefs changed?
Because if these things have changed, everything has to change. But if they haven't changed, you don't need to change anything. Keep going, that's how you persist.
04 Persist in doing something extremely difficult, excel at it and love it
Q: You mentioned that generative artificial intelligence and accelerated computing have reached a critical point. As this technology becomes more and more mainstream, what application are you most excited about?
Huang Renxun: You must return to the original intention, ask yourself what generative artificial intelligence is? What happened? We have software that can understand things, they can understand why...
We have digitized everything.
But what does this mean? Through a lot of learning, a lot of data, and from patterns and relationships, we now understand their meanings.
We are not learning them separately. We are learning spoken language, text, paragraphs, and vocabulary in the same context. We have found the correlations between them, they are all related to each other.
Now, we not only understand the modes, the meanings of each mode, but we also understand how to transform between them.
Obvious applications such as: video generating text, which is subtitles; text generating images like Midjourney; and text generating text like ChatGPT, it's amazing We now know, we understand the meaning, and we can also transform. The transformation of certain things is equivalent to generating information.
Suddenly, you have to take a step back and ask yourself, what impact will this have on every aspect of everything we do?
We are in a computational world. The way we handle information in the future will fundamentally change. This is why NVIDIA manufactures chips and systems. The way we write software will also change fundamentally.
The types of software we will have in the future will change and will give rise to new applications. Moreover, the way these applications are processed will also change.
In the past, models were based on retrieving pre-recorded information, we wrote text, pre-recorded it, and then retrieved it based on algorithms. In the future, some information seeds will become the starting point. We call it a Prompt, and then generate other content.
Future computing will heavily rely on generation. For example, as we are chatting now, most of the information I am giving you is not retrieved, but generated, this is called Generative Artificial Intelligence (GAI).
Future computing operations will heavily rely on generation, rather than retrieval.
Going back to the beginning, when you start a business, you have to ask yourself which industries will be disrupted by this? Will we still hold the same views on the internet? Will we still hold the same views on storage? Will we continue to abuse internet traffic as we do today? Probably not.
Host: If you close your eyes and magically change one thing about tomorrow, what would it be?
Huang Renxun: Personally, there are many things in the world that we cannot control. Your job is to make a unique contribution, live a purposeful life, do things that only you can do or will do.
Make a unique contribution, so that when you leave the world, everyone will feel that the world has become a better place because of you. For me, that's how I live my life.
I will fast forward to the future and look back. I will look back, review history. We solved some problems using this method, that way... does it make sense?
This is a bit like how you solve problems. You figure out the end result you want, and then work backwards to achieve it. So I envision NVIDIA making a unique contribution to driving the development of the computing field, because computing is the greatest driving force for human progress.
This is not self-aggrandizement, but because this is our area of expertise, and it is extremely challenging.
To this day, the company has been around for 31 years, but our journey has just begun. This is an extremely difficult goal.
When I look back on the past, I believe we will be remembered as a company that changed the world, not because we preached everywhere about changing the world through words and actions, but because we persisted in doing something extremely difficult, something we are good at, passionate about, and have been doing for a long time.
05 The boundary between security and AI will become blurred and closely integrated Question: Are you concerned about the speed at which we are developing AI?
Huang Renxun: The answer is both yes and no. The greatest breakthrough in modern AI is deep learning, which has made significant progress.
But another incredible breakthrough is a capability that humans often have and use, which is reinforcement learning and human feedback. That is my work.
Now, we are just beginning to understand how to systematically apply this to artificial intelligence. There are many other preventive measures, such as fine-tuning and basics.
Currently, some models generate objects that float in space and do not follow the laws of physics. This requires technology to solve. Prevention requires technology, fine-tuning requires technology, aligning AI with human goals requires technology, and safety also requires technology.
The reason why airplanes are safe is because all the autopilot systems are supported by diverse and redundant systems, as well as various newly invented functional safety and active safety systems. We need to invent all similar technologies faster and faster.
The boundary between safety and artificial intelligence will become blurred and closely integrated. In the field of cybersecurity, we need technology to progress very, very quickly to protect us from the harm of artificial intelligence.
In many ways, we need technology to advance much faster than it is now.
How should we respond to the impact that AI brings to society? I don't have a good answer. It is important to break everything down into many sub-problems so that we do not overly focus on one area and forget about the many routine areas where things can still be done.
We should ensure that we pragmatically address these routine areas.
06 The marginal cost of computing has dropped to near zero, making many possibilities happen
Question: In the past, the breakthrough in semiconductor technology was the transistor, which is now a very basic invention. Should we rethink that technological breakthroughs should turn into artificial intelligence?
Huang Renxun: First of all, the transistor is obviously a great invention, but its greatest ability is that it made software possible. Humans can express our ideas and algorithms in a repeatable computational way, which is a breakthrough.
For the past 31 years, our company has been dedicated to a new form of computing called accelerated computing. Our idea is that general-purpose computing is not suitable for every field of work.
We have introduced a new way of software development that was previously done by humans. Now we can let computers write software because the cost of computation is close to zero.
In the past 10 years, we have reduced the cost of deep learning computation by 1 million times.
Large language models extract all human knowledge from the internet and put it into a computer to figure out what knowledge is.
The idea is to scrape all the content from the entire internet, put it into a computer, and let the computer figure out what the program is.
This is a crazy concept, but you would never consider doing it if you didn't reduce the marginal cost of computing to zero.
We have made this breakthrough. Now we have enabled this new way of software development Artificial intelligence, this is what we call a new form of accelerated computing. We have spent thirty years working on it, and it may be the greatest invention in the computer industry.
Question: I know you are launching the H200, planning to upgrade it annually. So, will the H700 in 5 years allow us to do things we can't do now?
Huang Renxun: The next thing coming is liquid cooling technology, which calculates on the scale of data centers. In the next 10 years, we will increase the computing power for deep learning by another 1 million times.
What happens when you do this? Today we learn, then we apply. We train for reasoning, we learn, then we apply.
In the future, we will have continuous learning and can decide whether to deploy the results of that continuous learning to applications in the world, but computers will observe videos and new text, continuously improving themselves from all interactions.
Learning, training, reasoning, deployment, and application processes will all become one. That's what we do.
The reinforcement learning loop of reasoning, training, and application will be continuous, and reinforcement learning will be based on real-time interaction and synthetic data we create in real time.
Just like when you learn, you get snippets of information, then you start from first principles, simulate in our brains, imagine states, future states that in many ways manifest as reality for us.
Future artificial intelligence computers will do the same. They will generate synthetic data, they will do reinforcement learning, they will continue based on real-world experiences.
They will imagine things, they will test it with real-world experiences. They will cycle back and forth based on this.
When you can reduce the marginal cost of computation to near zero, there will be many new ways to do what you want to do.
This is no different from me wanting to go further, because the marginal cost of transportation has been reduced to zero. I can fly relatively cheaply from here to New York. If it took a month, I might never go.
This is the same with everything we do, we will reduce the marginal cost of computation to near zero. Therefore, we will do more computation.
Source: Internet
07 The difficulty of reasoning chips lies in the need for a huge installation base
Question: There have been reports recently that NVIDIA will face more competition in the inference market than in the training market. But what you are talking about is actually one market. Can you comment on this?
Huang Renxun: Today, whenever you prompt NVIDIA, whether it's ChatGPT or Copilot, Mid-Journey, or the service platform you are using now, you are doing inference. Inference is happening there.
It generates information for you. What is behind it every time you do this? Almost 100% is NVIDIA's GPU Now, is reasoning difficult or easy? Many people see training and say, "This looks too hard. You have to invest $2 billion to prove something is effective."
You invest $2 billion and two years, and then you open it up and find it's not very effective. The risk of exploring new things is too high for customers.
So many competitors tend to say we don't do training, we do reasoning chips.
But in fact, reasoning is very difficult. The response time for reasoning must be very fast, which is part of computer science and is actually easy.
The difficulty of reasoning lies in the fact that the goal of reasoning is to attract more users and apply their software to a large installed base.
Reasoning is an installed base issue, similar to launching an app on the iPhone. Because the iPhone has such a large installed base, writing applications on it benefits from being able to reach everyone.
In the case of NVIDIA, our accelerated computing platform CUDA is the only truly ubiquitous accelerated computing platform.
Because we have been working on this for a long time, if you write an application for reasoning and deploy that model on the video architecture, it can actually run anywhere.
So you can reach everyone. You can have a greater impact. The problem with reasoning is actually the installed base. It requires tremendous patience and years of success and dedication, as well as investment in architecture, compatibility, and other aspects.
Q: How do you view threats from competitors like AMD?
Huang Renxun: First of all, we have more competitors than anyone else on Earth. We not only have competition from competitors, but also from customers (cloud computing).
But I not only show them my current chip, but also show them my next chip, and I will also show them my chip adapter.
The reason is, you see, if you don't try to explain why you are good at something, they will never have a chance to buy your product.
So when we work with almost everyone in the industry, we are completely open. Certainly, you can build a specific chip (ASIC), but remember, computing is not just Transformers, and we are constantly inventing new variants of transformers.
The types of software are very rich. Software engineers like to create new things, and what NVIDIA is good at is accelerated computing. Our architecture can not only accelerate algorithms, but is also programmable. We can accelerate quantum physics, accelerate all fluid and particle codes, and so on in a wide range of fields.
One of them is generative AI. For those who want a large number of customers in data centers, financial services, or manufacturing, we are an excellent standard.
We exist in every cloud service, in every computer company. Our architecture has become a standard after about 30 years, and that is our advantage. If customers can do something specific (on this basis), it is more cost-effective.
Remember, our chip is just a part. When you look at a computer now, it's a data center, and you need to operate it. So people who buy and sell chips consider the price of the chip The operators in the data center consider operating costs, performance, deployment time, and utilization, etc. Our total cost of ownership (TCO) is very good. Even if the competitor's chips are free, overall it is not cheap.
Our goal is to add more value. This requires a lot of effort, we must continue to innovate, and we must not take it lightly.
Source: Internet
Within 5 years, AGI can pass human tests
Q: When do you think we will achieve human-level general artificial intelligence? Is it 50 years from now? Or 5 years from now?
Huang Renxun: I will give a very specific answer, but first let me tell you some very exciting things that are happening.
First, the models we are training are multimodal, which means we will learn from sound, from text, from vision, just like all of us watch TV and learn from it. Of course, this is where ChatGPT truly innovates, that is, RLHF.
But until reinforcement learning, humans will anchor AI in what we consider good human values.
Now, can you imagine, you have to generate images and videos, AI knows that hands won't go through the podium, and when you step on water you will fall in, so now AI is starting to anchor in physics.
AI watches a lot of different examples, such as videos, to learn the rules that govern this world. It must create a so-called world model. So, we must understand multimodality, as well as other modalities, such as genes, amino acids, proteins, cells, and so on.
The second point is that AI will have stronger and stronger reasoning abilities, much of the reasoning we humans do is encoded in common sense. Common sense is the ability that all of us humans take for granted.
There is a lot of reasoning and knowledge already encoded on the internet that models can learn. There are higher levels of reasoning abilities, for example, now when you ask me a question, most questions, I can quickly generate like a generative model.
But some questions, I need to think about, that is planning. AI is not good at this kind of "long thinking." Everything you input into ChatGPT, it will respond immediately.
We hope that if you input a question into ChatGPT, give it a goal, give it a mission, it can think for a while.
So, this kind of system, computer science calls it system 2, or long thinking, or planning. I think we are researching these things, and you will see some breakthroughs.
So in the future, your interaction with artificial intelligence will be very different. Some are just give me a question, I will give you an answer. Some are like, here is a question, go work on it for a while, tell me tomorrow. It will do as much computation as possible You can also say, I give you this question, you can spend $1000, but not more than that, and then it will give you the best answer tomorrow.
So, back to the question of AGI, what is the definition of AGI? In fact, this is the question that needs to be answered first.
If AI is given many math tests, reasoning tests, history tests, biology tests, medical exams, and even law exams, including SAT, MCAT, etc., if you list these tests and put them in front of the computer science industry, I guess we will do well on every test within 5 years.
So, if your definition of AGI is passing human tests, then I will tell you, in five years we will pass all the tests.
But if you ask me in a slightly different way, if AGI is to have human intelligence, then I am not sure how to specifically define all human intelligence, no one really knows, so this is difficult to achieve, but we are all working to make it better.
09 Resilience is very important in success
Q: According to your prediction, in the next 5 to 10 years, how much additional semiconductor manufacturing capacity will be needed to support the development of artificial intelligence?
Huang Renxun: Actually, I am very bad at predictions, but I am very good at reasoning based on first principles. So let me reason for you first.
I don't know how many wafer fabs are needed, but I know one thing. The way we do computation now. Information is written by someone, created by someone.
Basically, all text, all videos, all sounds are pre-recorded. Everything we do is based on retrieval.
In the future, because we will have an AI that understands the current situation, as it can access all the latest news in the world, etc., this is called retrieval-based.
It understands your context, meaning it understands what you are asking. When you and I talk about the economy, we may mean very different things. Based on that, it can generate completely correct information for you.
So in the future, it has already understood the context. And most of the computation will be generative. Today, 100% of the content is pre-recorded. If in the future, 100% of the content will be generative.
The question is, how will this change the shape of computation without making you feel troubled? That's how I reason.
How many networks do we need? How much memory do we need? The answer is, we need more wafer fabs.
But please remember, we are also greatly improving the efficiency of algorithms and processing. It's not that the efficiency of computation is the same as today. At the same time, demand is rising.
This must offset each other. And there is also technological diffusion, etc. It's just a matter of time, but that doesn't change the fact that one day, all the computers in the world will change 100%.
Every data center, infrastructure worth trillions of dollars, will be completely changed. And new infrastructure will be built on that foundation.
Q: For students majoring in computer science or engineering, what advice would you give them to increase their chances of success? Huang Renxun I think one of my major advantages is that my expectations are very low. I think most Stanford graduates have very high expectations.
People with high expectations usually have low resilience. Unfortunately, resilience is very important for success. I don't know how to teach you, except that I hope pain happens to you.
I am very lucky. In the environment I grew up in, my parents provided us with the conditions for success, but at the same time, there were enough setbacks and opportunities for pain.
Even today, I often use the words "pain and torment" in our company.
Greatness does not come from intelligence, greatness comes from character. Smart people need to experience pain to build such character