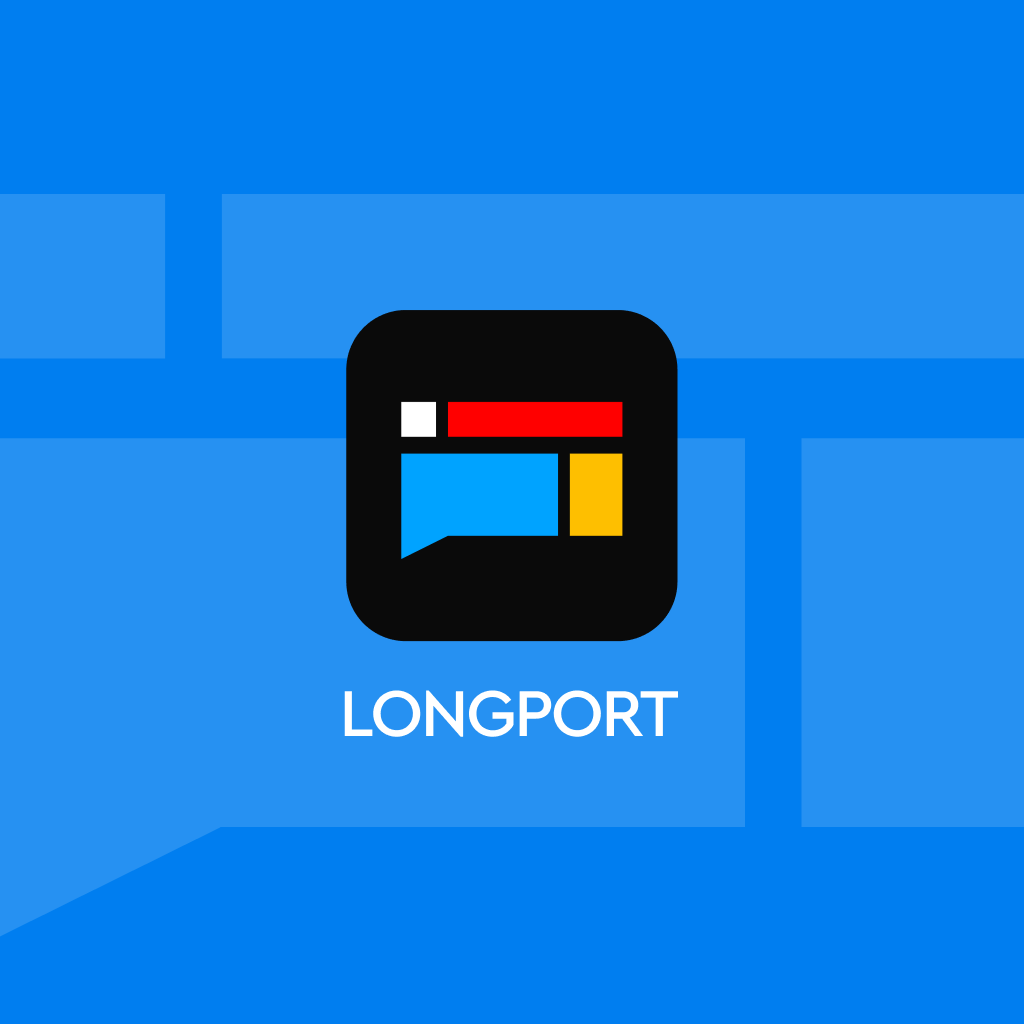
The key model behind Sora, the breakthrough to achieving AGI?
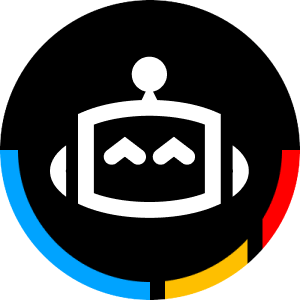
The contribution of Transformer to the Diffusion process is similar to an engine upgrade.
Sora, who emerged out of nowhere, has crushed other text-to-video models, making global film industry practitioners tremble, injecting a strong stimulant into the surging AI trend, and further solidifying OpenAI's position as the leader in cutting-edge GenAI technology.
However, the technology driving Sora is actually based on the Diffusion Transformer architecture that appeared in the field of artificial intelligence research several years ago.
The most outstanding feature of this architecture is that it allows AI models to surpass previous technological limitations. The larger the parameter scale, the longer the training time, and the larger the training dataset, the better the video generation effect. Sora is a product of this "miraculous effort".
What is a Diffusion Transformer
In machine learning, there are two key concepts: 1) Diffusion; 2) Transformer.
First, let's talk about Diffusion. Most AI models that can generate images and videos, including OpenAI's DALL-E3, rely on a process called Diffusion to output images, videos, audio, and other content.
The working principle of Diffusion is to disrupt training data by continuously adding Gaussian noise (forward process), and then learn to restore the data by reversing this noise (reverse process). That is, random sampled noise is first input into the model, and data is generated through the learning denoising process.
During the reverse process of the model, diffusion relies on an engine called U-Net to learn to estimate the noise to be removed. However, U-Net is very complex, and its specially designed modules significantly reduce the speed at which diffusion generates data.
Transformer is the technological foundation behind mainstream LLMs such as GPT-4 and Gemini. It can replace U-Net and improve the efficiency of the Diffusion process. Transformer has a unique "attention mechanism". For each input data (such as image noise in Diffusion), Transformer balances the relevance of every other input (other noise in the image) and learns from it to generate results (estimated value of image noise).
The attention mechanism not only makes Transformer simpler than other model architectures but also makes the architecture parallelizable. In simple terms, it means that larger Transformer models can be trained, significantly improving computational capabilities. The concept of Diffusion Transformer was jointly proposed by Xie Saining, a computer professor at New York University, and William Peebles, the current co-director of OpenAI Sora.
Professor Xie Saining mentioned in a media interview:
"The contribution of Transformer to the Diffusion process is similar to upgrading an engine. The introduction of Transformer... signifies a significant leap in scalability and effectiveness. This is particularly evident in models like Sora, which benefit from training on massive video data and utilize higher model parameters to demonstrate the transformative potential of Transformer in large-scale applications."
Sora is the result of "great power comes great responsibility"
According to the analysis by Huafu Securities, the process of generating videos in Sora is roughly as follows:
- Video Encoding: VisualEncoder compresses the original video into a low-dimensional latent space, then decomposes the video into spatiotemporal patches and flattens them into a series of video tokens for processing by the transformer.
- Noise Addition and Denoising: In the diffusion model under the transformer architecture, spatiotemporal patches are conditioned with text, undergo noise addition and denoising successively to reach a decodable state.
- Video Decoding: Mapping the denoised low-dimensional latent representation back to pixel space.
It can be seen that the main feature of Sora is the replacement of the U-Net engine with a transformer. Analyst Shi Xiaojun believes that replacing U-Net with DiT's transformer as the model architecture in Sora has two major advantages:
- Transformer can decompose input videos into 3D patches, similar to how DiT decomposes images into blocks, breaking through limitations such as resolution and size, and can simultaneously process multi-dimensional information in time and space.
- Transformer continues the Scaling Law of OpenAI, with strong scalability. The larger the parameter scale, the longer the training time, and the larger the training dataset, the better the quality of the generated videos. For example, as the training iterations increase, the quality of the video of a puppy in the snow in Sora significantly improves.
However, the biggest drawback of Transformer is its high cost.
The memory requirements of its full attention mechanism will quadratically increase with the length of the input sequence, making it inadequate for processing high-resolution image signals. When dealing with high-dimensional signals like videos, the growth pattern of Transformer will make the computational cost very high. In other words, Sora's birth is the result of OpenAI, backed by Microsoft, burning computational power like crazy. Compared to the U-Net architecture, Transformer highlights the "brutal aesthetics" under the Scaling Law, where the larger the parameter scale, the longer the training time, and the larger the training dataset, the better the video generation effect; furthermore, under the large-scale training of Transformer, the scale effect gradually emerges, unleashing the emergent capability of the model.